Automating Energy Demand Modeling and Forecasting Using Smart Meter Data
2019 IEEE International Congress on Internet of Things (ICIOT)(2019)
摘要
The Internet of Things (IoT) technology with a variety of smart devices, communication networks, and software systems for data processing is critical for optimizing Smart Grid operations. While energy demand forecasting is an integral part of Smart Grid management, there is no a general forecasting method which works best across different situations and scenarios. In this paper, we design, build, and compare three forecasting methods to show how these approaches can be trained on time-series data with limited intervention from users, i.e., enabling automated model selection. Using smart meters measurements collected from 114 residential apartments over two years, and weather information for the same period, we build the following three models: (i) a piecewise linear regression model, (ii) the univariate seasonal ARIMA model, and (iii) the novel multivariate LSTM model (a special form of Recurrent Neural Network). While the designed linear regression model could be used for longer-term planning, the constructed LSTM model significantly improves the accuracy of short term (24 hours) demand prediction compared to both the linear regression and ARIMA models. However, training LSTM and modeling with ARIMA require higher computing resources and longer runtimes (45 min and 125 min respectively) compared to 2 min for building and predicting with linear regression model. Therefore, the automated best model selection can be driven by the user-defined metrics of interest, such as model accuracy, required computing resources, model usability, and model construction/execution time.
更多查看译文
关键词
Smart Grid, smart meters, data analytics, demand forecasting, automated model selection
AI 理解论文
溯源树
样例
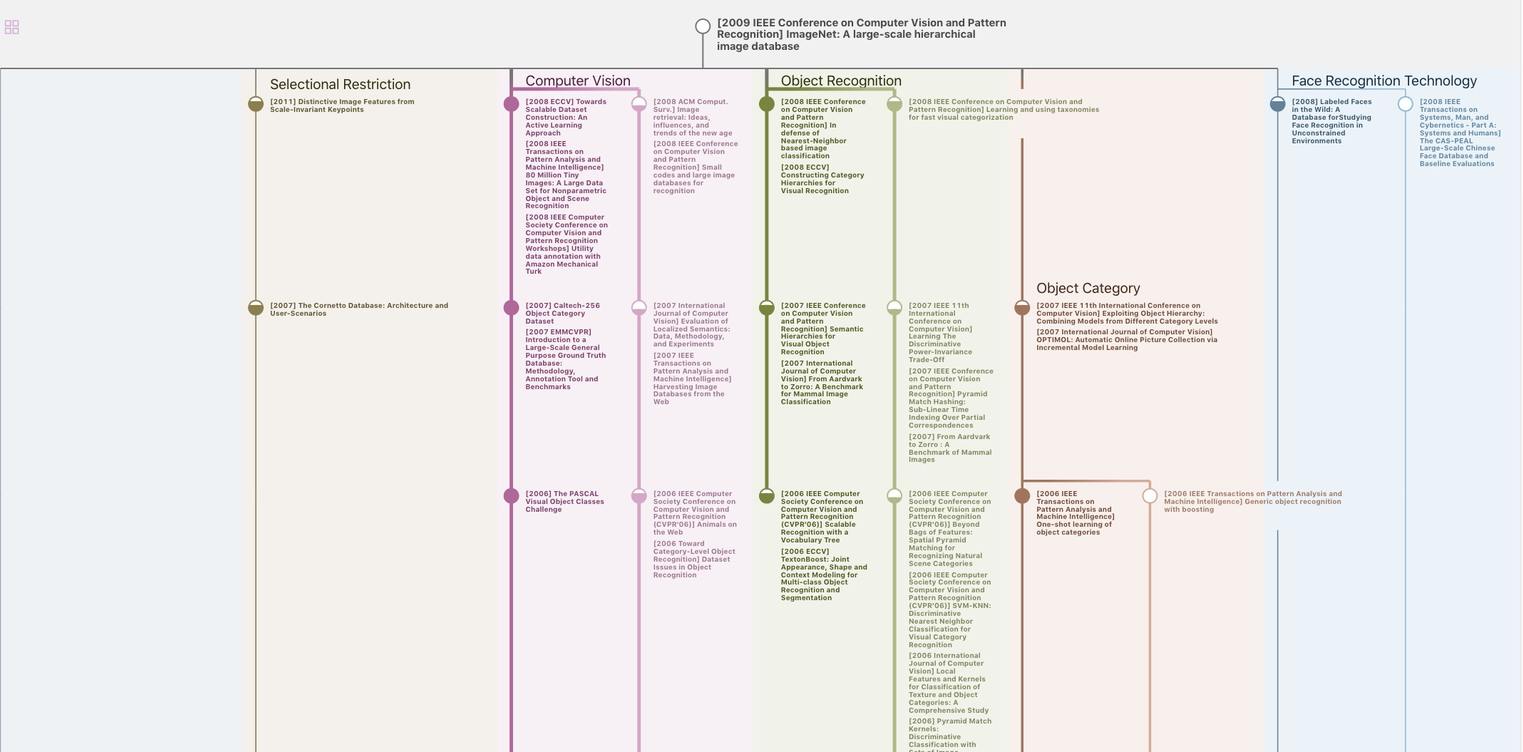
生成溯源树,研究论文发展脉络
Chat Paper
正在生成论文摘要