Driving Behavior Modeling Based On Hidden Markov Models With Driver'S Eye-Gaze Measurement And Ego-Vehicle Localization
2019 30TH IEEE INTELLIGENT VEHICLES SYMPOSIUM (IV19)(2019)
摘要
This paper presents a comparison of driving behavior modeling methods based on hidden Markov models (HMMs) with driver's eye-gaze measurement and ego-vehicle localization. Original HMMs are sometimes insufficient to model real-world scenarios. To overcome these limitations, extended HMMs have been proposed, e.g., autoregressive input-output HMMs (AIOHMMs). This paper first details AIOHMMs and presents ways to use them for driving behavior modeling. We compare the performance for behavior modeling and maneuver discrimination for six types of HMMs. The driving data for this work was gathered in our university campus with a car-like vehicle. Experimental results suggest that the hidden states can properly represent the average of the driving actions when the driving behaviors are accurately modeled by the HMMs. It is also suggested that surrounding and past information can be used to flexibly model the relationship between driving actions and related information.
更多查看译文
关键词
ego-vehicle localization,hidden Markov models,original HMMs,extended HMMs,autoregressive input-output HMMs,driving data,driving actions,driving behavior modeling methods,eye-gaze measurement,maneuver discrimination,university campus,car-like vehicle
AI 理解论文
溯源树
样例
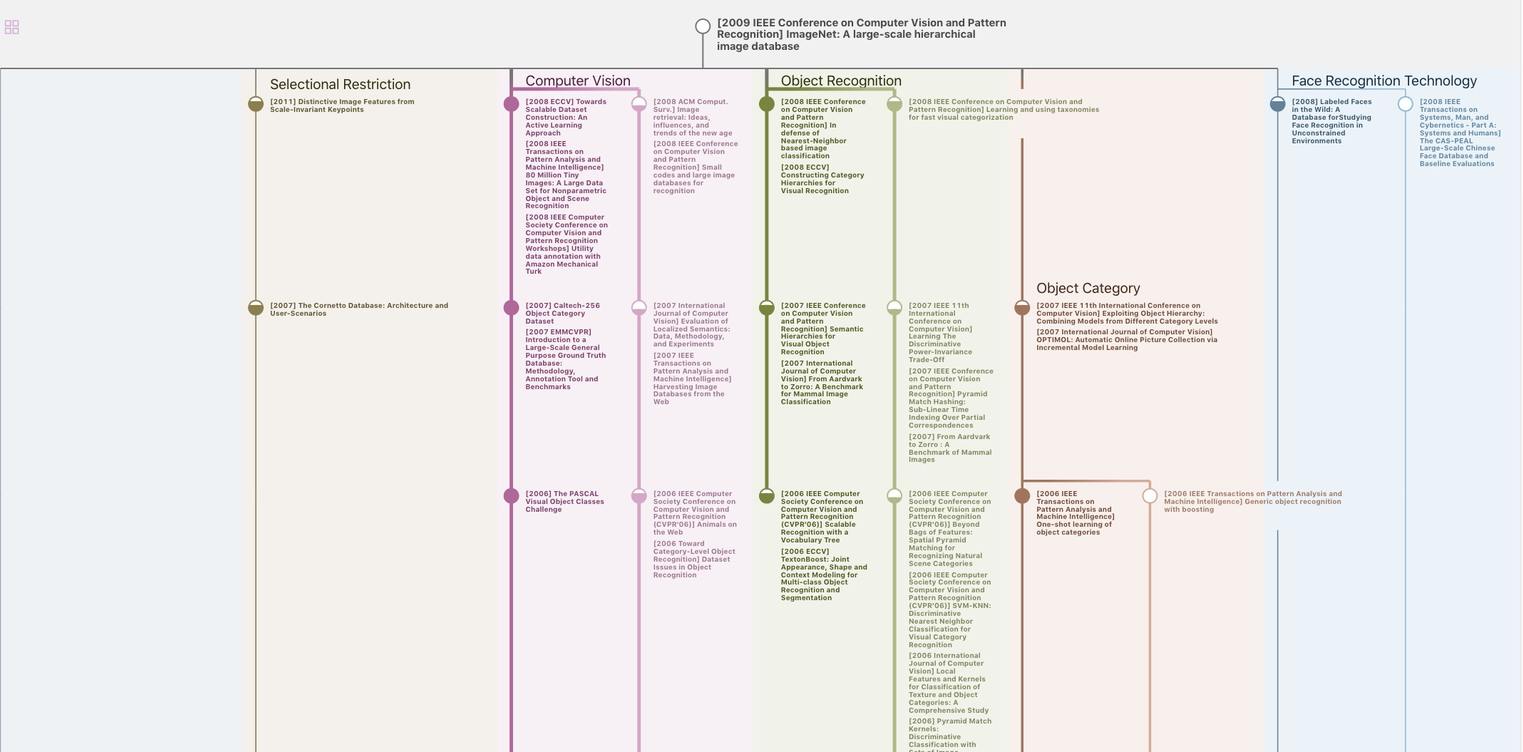
生成溯源树,研究论文发展脉络
Chat Paper
正在生成论文摘要