Cross-Sensor Deep Domain Adaptation for LiDAR Detection and Segmentation
2019 IEEE Intelligent Vehicles Symposium (IV)(2019)
摘要
A considerable amount of annotated training data is necessary to achieve state-of-the-art performance in perception tasks using point clouds. Unlike RGB-images, LiDAR point clouds captured with different sensors or varied mounting positions exhibit a significant shift in their input data distribution. This can impede transfer of trained feature extractors between datasets as it degrades performance vastly. We analyze the transferability of point cloud features between two different LiDAR sensor set-ups (32 and 64 vertical scanning planes with different geometry). We propose a supervised training methodology to learn transferable features in a pre-training step on LiDAR datasets that are heterogeneous in their data and label domains. In extensive experiments on object detection and semantic segmentation in a multi-task setup we analyze the performance of our network architecture under the impact of a change in the input data domain. We show that our pre-training approach effectively increases performance for both target tasks at once without having an actual multi-task dataset available for pre-training.
更多查看译文
关键词
supervised training methodology,point cloud features,trained feature extractors,input data distribution,mounting positions,LiDAR point clouds,RGB-images,perception tasks,annotated training data,LiDAR detection,cross-sensor deep domain adaptation,multitask dataset,target tasks,multitask setup,semantic segmentation,object detection,LiDAR datasets
AI 理解论文
溯源树
样例
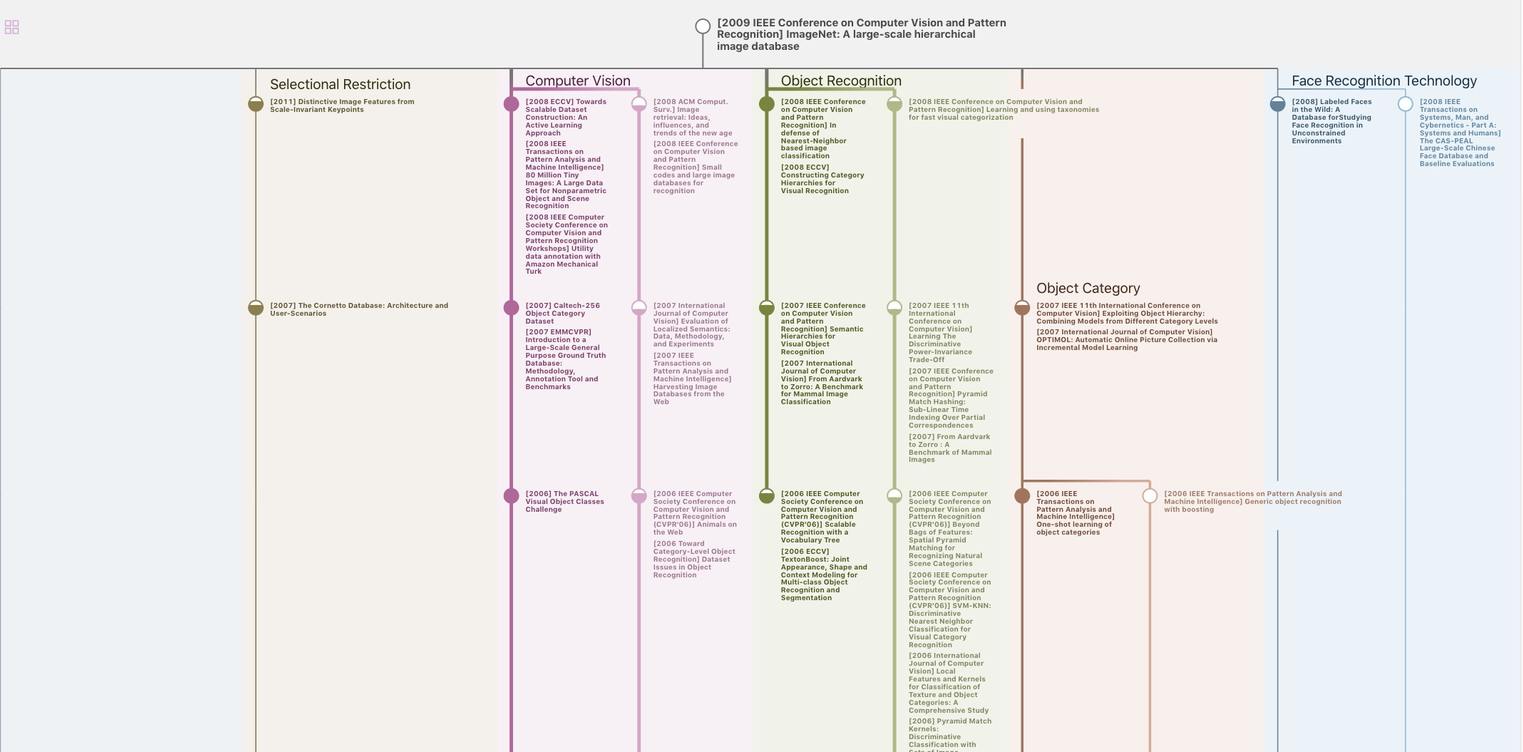
生成溯源树,研究论文发展脉络
Chat Paper
正在生成论文摘要