Contextual Minimum-Norm Estimates (CMNE): A Deep Learning Method for Source Estimation in Neuronal Networks
arxiv(2019)
摘要
Magnetoencephalography (MEG) and Electroencephalography (EEG) source estimates have thus far mostly been derived sample by sample, i.e., independent of each other in time. However, neuronal assemblies are heavily interconnected, constraining the temporal evolution of neural activity in space as detected by MEG and EEG. The observed neural currents are thus highly context dependent. Here, a new method is presented which integrates predictive deep learning networks with the Minimum-Norm Estimates (MNE) approach. Specifically, we employ Long Short-Term Memory (LSTM) networks, a type of recurrent neural network, for predicting brain activity. Because we use past activity (context) in the estimation, we call our method Contextual MNE (CMNE). We demonstrate that these contextual algorithms can be used for predicting activity based on previous brain states and when used in conjunction with MNE, they lead to more accurate source estimation. To evaluate the performance of CMNE, it was tested on simulated and experimental data from human auditory evoked response experiments.
更多查看译文
关键词
neuronal networks,source estimation,deep learning,cmne,deep learning method,minimum-norm
AI 理解论文
溯源树
样例
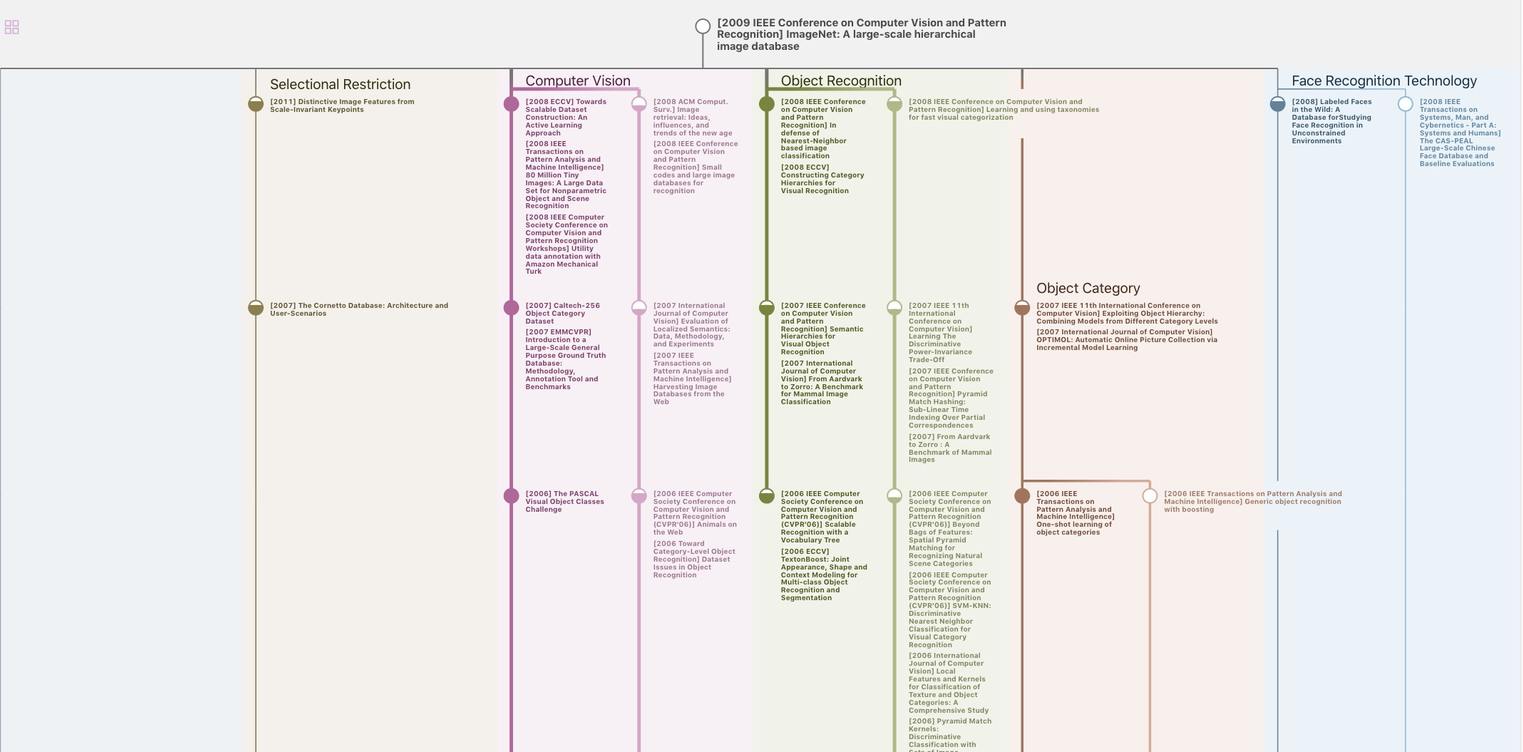
生成溯源树,研究论文发展脉络
Chat Paper
正在生成论文摘要