Improved Patient Classification with Language Model Pretraining Over Clinical Notes
arxiv(2019)
摘要
Clinical notes in electronic health records contain highly heterogeneous writing styles, including non-standard terminology or abbreviations. Using these notes in predictive modeling has traditionally required preprocessing (e.g. taking frequent terms or topic modeling) that removes much of the richness of the source data. We propose a pretrained hierarchical recurrent neural network model that parses minimally processed clinical notes in an intuitive fashion, and show that it improves performance for multiple classification tasks on the Medical Information Mart for Intensive Care III (MIMIC-III) dataset, increasing top-5 recall to 89.7% (up by 4.8%) for primary diagnosis classification and AUPRC to 35.2% (up by 2.4%) for multilabel diagnosis classification compared to models that treat the notes as an unordered collection of terms or without pretraining. We also apply an attribution technique to several examples to identify the words and the nearby context that the model uses to make its prediction, and show the importance of the words' context.
更多查看译文
AI 理解论文
溯源树
样例
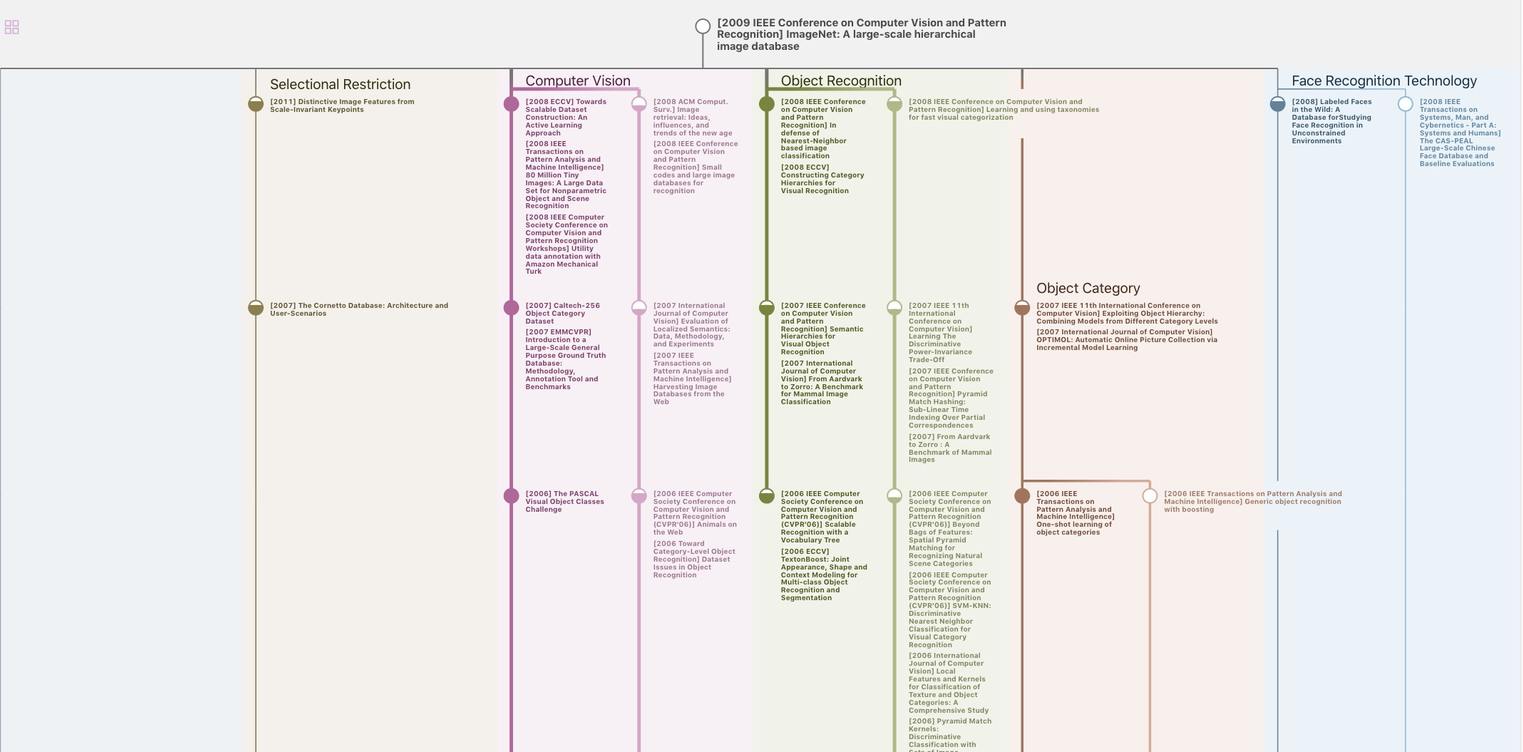
生成溯源树,研究论文发展脉络
Chat Paper
正在生成论文摘要