A new analysis of differential privacy’s generalization guarantees (invited paper)
STOC '21: PROCEEDINGS OF THE 53RD ANNUAL ACM SIGACT SYMPOSIUM ON THEORY OF COMPUTING(2021)
摘要
ABSTRACTWe give a new proof of the "transfer theorem" underlying adaptive data analysis: that any mechanism for answering adaptively chosen statistical queries that is differentially private and sample-accurate is also accurate out-of-sample. Our new proof is elementary and gives structural insights that we expect will be useful elsewhere. We show: 1) that differential privacy ensures that the expectation of any query on the conditional distribution on datasets induced by the transcript of the interaction is close to its true value on the data distribution, and 2) sample accuracy on its own ensures that any query answer produced by the mechanism is close to its conditional expectation with high probability. This second claim follows from a thought experiment in which we imagine that the dataset is resampled from the conditional distribution after the mechanism has committed to its answers. The transfer theorem then follows by summing these two bounds. An upshot of our new proof technique is that the concrete bounds we obtain are substantially better than the best previously known bounds.
更多查看译文
关键词
Adaptive Data Analysis,Differential Privacy,Generalization
AI 理解论文
溯源树
样例
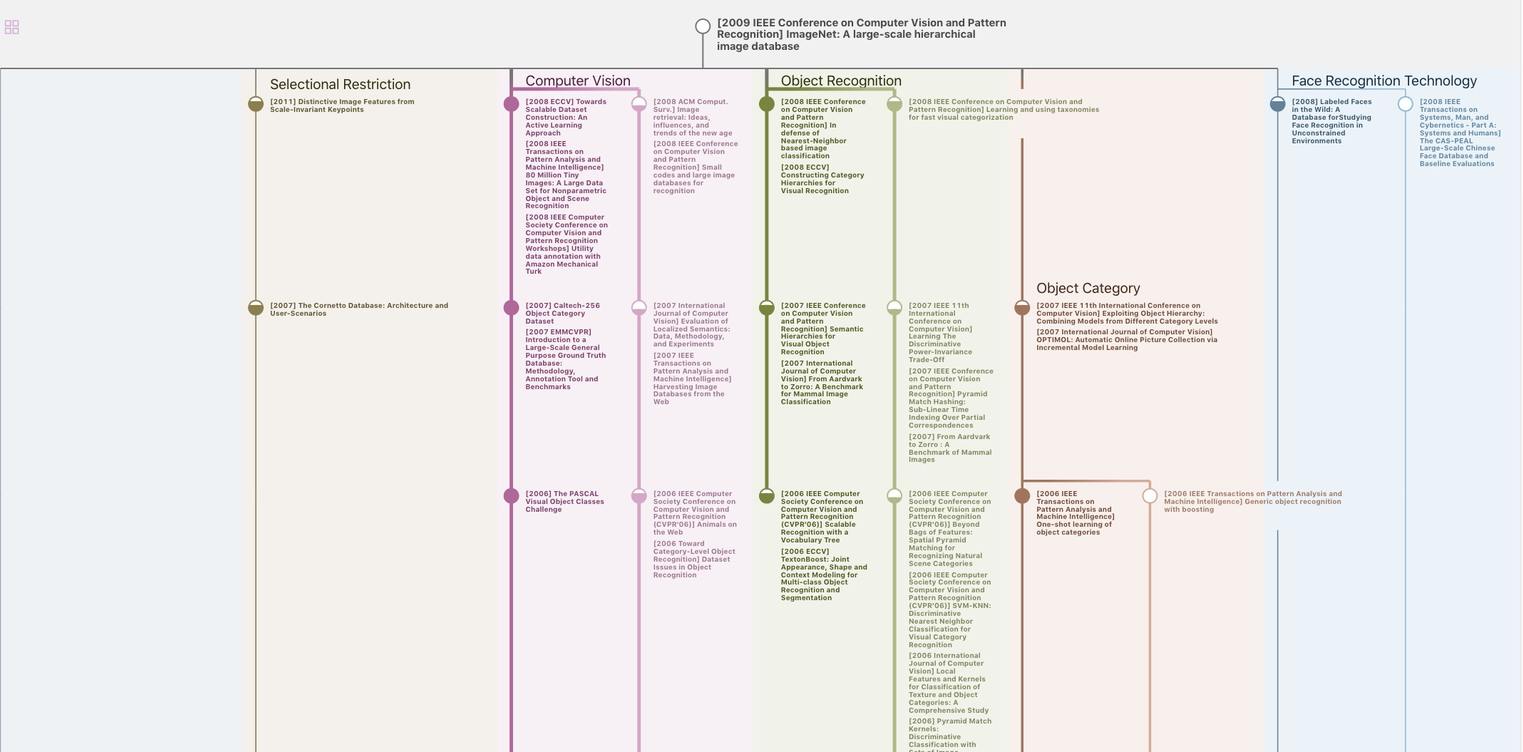
生成溯源树,研究论文发展脉络
Chat Paper
正在生成论文摘要