Sequential Monte Carlo with transformations
STATISTICS AND COMPUTING(2019)
摘要
This paper examines methodology for performing Bayesian inference sequentially on a sequence of posteriors on spaces of different dimensions. For this, we use sequential Monte Carlo samplers, introducing the innovation of using deterministic transformations to move particles effectively between target distributions with different dimensions. This approach, combined with adaptive methods, yields an extremely flexible and general algorithm for Bayesian model comparison that is suitable for use in applications where the acceptance rate in reversible jump Markov chain Monte Carlo is low. We use this approach on model comparison for mixture models, and for inferring coalescent trees sequentially, as data arrives.
更多查看译文
关键词
Bayesian model comparison,Coalescent,Trans-dimensional Monte Carlo
AI 理解论文
溯源树
样例
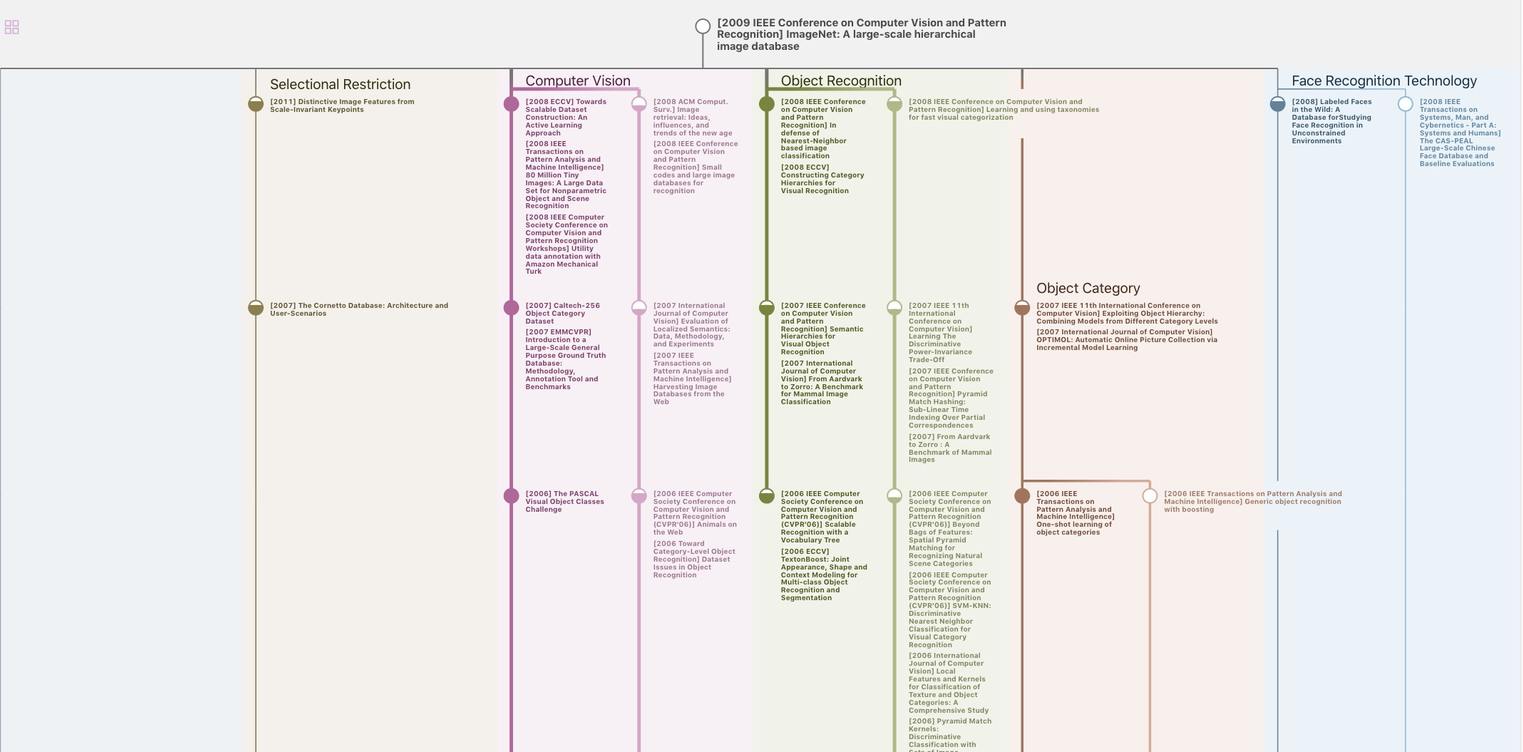
生成溯源树,研究论文发展脉络
Chat Paper
正在生成论文摘要