Towards Understanding the Importance of Shortcut Connections in Residual Networks
ADVANCES IN NEURAL INFORMATION PROCESSING SYSTEMS 32 (NIPS 2019)(2019)
摘要
Residual Network (ResNet) is undoubtedly a milestone in deep learning. ResNet is equipped with shortcut connections between layers, and exhibits efficient training using simple first order algorithms. Despite of the great empirical success, the reason behind is far from being well understood. In this paper, we study a two-layer non-overlapping convolutional ResNet. Training such a network requires solving a non-convex optimization problem with a spurious local optimum. We show, however, that gradient descent combined with proper normalization, avoids being trapped by the spurious local optimum, and converges to a global optimum in polynomial time, when the weight of the first layer is initialized at 0, and that of the second layer is initialized arbitrarily in a ball. Numerical experiments are provided to support our theory.
更多查看译文
关键词
residual network (resnet)
AI 理解论文
溯源树
样例
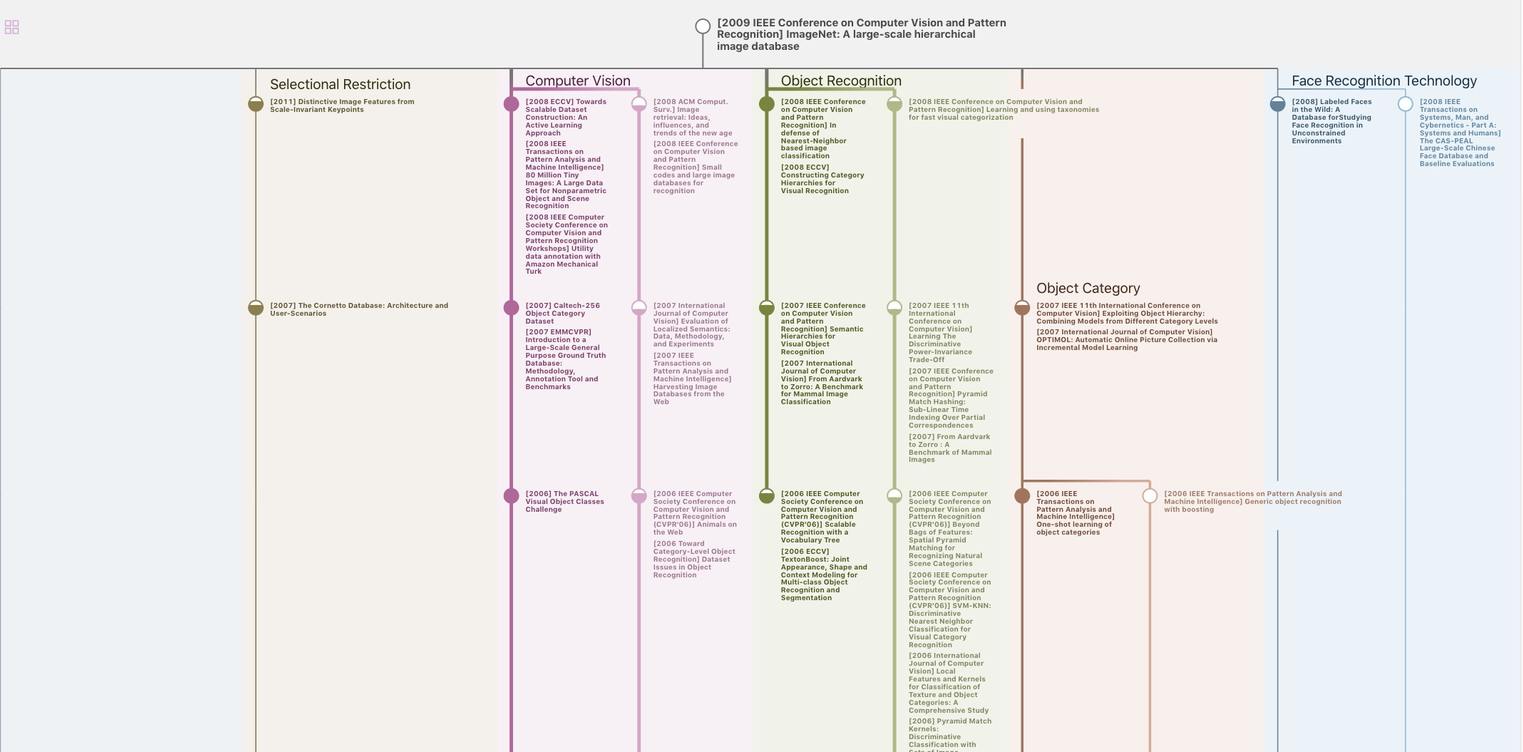
生成溯源树,研究论文发展脉络
Chat Paper
正在生成论文摘要