Transfer Learning For Named Entity Recognition In Financial And Biomedical Documents
INFORMATION(2019)
摘要
Recent deep learning approaches have shown promising results for named entity recognition (NER). A reasonable assumption for training robust deep learning models is that a sufficient amount of high-quality annotated training data is available. However, in many real-world scenarios, labeled training data is scarcely present. In this paper we consider two use cases: generic entity extraction from financial and from biomedical documents. First, we have developed a character based model for NER in financial documents and a word and character based model with attention for NER in biomedical documents. Further, we have analyzed how transfer learning addresses the problem of limited training data in a target domain. We demonstrate through experiments that NER models trained on labeled data from a source domain can be used as base models and then be fine-tuned with few labeled data for recognition of different named entity classes in a target domain. We also witness an interest in language models to improve NER as a way of coping with limited labeled data. The current most successful language model is BERT. Because of its success in state-of-the-art models we integrate representations based on BERT in our biomedical NER model along with word and character information. The results are compared with a state-of-the-art model applied on a benchmarking biomedical corpus.
更多查看译文
关键词
deep learning, entity extraction, named entity recognition, transfer learning, fine-tuning, minimum training data
AI 理解论文
溯源树
样例
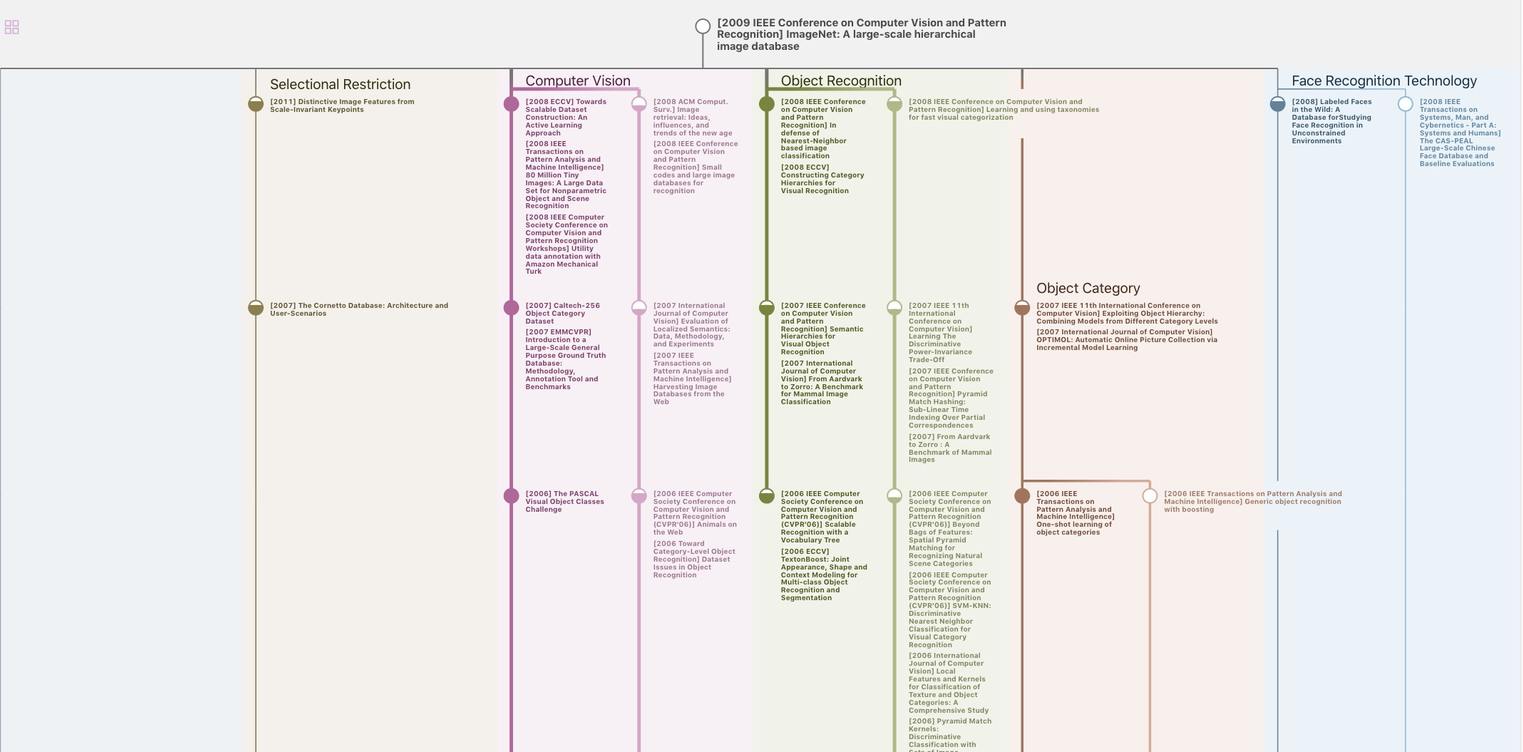
生成溯源树,研究论文发展脉络
Chat Paper
正在生成论文摘要