Receding Horizon Markov Game Autonomous Driving Strategy.
American Control Conference(2019)
摘要
This paper presents a novel human-like autonomous driving algorithms for lane-changing problem. To this end, we present a multi-agent decision-making scheme by blending game theory with the Markov decision process, forming a Markov game (MG). In this decision-making process, interaction of a subject vehicle (SV) and traffic vehicles (TVs) are captured in a mathematically tractable manner via both a cooperative game (max - max) where vehicles perform their decisions for collective objectives and a noncooperative game (max - min) where vehicles perform their decisions for individual objectives. Strategies of the players are computed via a Receding Horizon (RH) approach where optimal solutions are found through an optimization strategy by taking into account current and future constraints. The proposed approach is evaluated in a human-in-the-Ioop (HIL) environment built around a MATLAB/SimulinkldSPACE realtime simulator where the Markov game-guided SV controller interacts with programmed TV s and one human-driven vehicle (HV). Experimental results show that the Markov game driving strategy is capable of finding a safe gap in multi-move traffic that is consistent with human drivers' behaviours in mandatory lane-changing.
更多查看译文
关键词
Markov game driving strategy,multimove traffic,human drivers,mandatory lane-changing,human-like autonomous driving algorithms,lane-changing problem,multiagent decision-making scheme,game theory,Markov decision process,decision-making process,traffic vehicles,noncooperative game,human-in-the-Ioop environment,Markov game-guided SV controller interacts,receding horizon approach,horizon Markov game autonomous driving strategy,MATLAB/SimulinkldSPACE realtime simulator
AI 理解论文
溯源树
样例
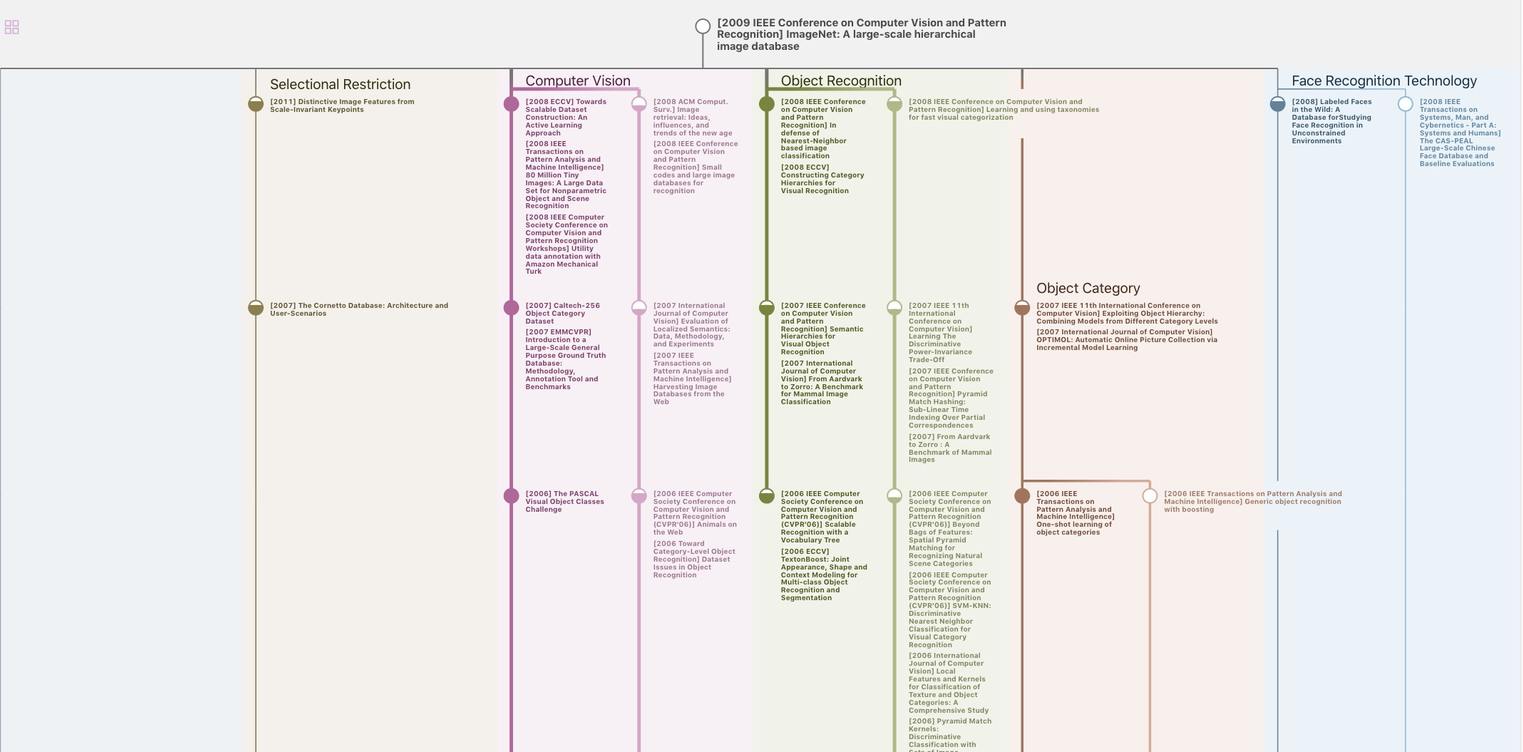
生成溯源树,研究论文发展脉络
Chat Paper
正在生成论文摘要