Product collection recommendation in online retail
Proceedings of the 13th ACM Conference on Recommender Systems(2019)
摘要
Recommender systems are an integral part of eCommerce services, helping to optimize revenue and user satisfaction. Bundle recommendation has recently gained attention by the research community since behavioral data supports that users often buy more than one product in a single transaction. In most cases, bundle recommendations are of the form "users who bought product A also bought products B, C, and D". Although such recommendations can be useful, there is no guarantee that products A, B, C, and D may actually be related to each other. In this paper, we address the problem of collection recommendation, i.e., recommending a collection of products that share a common theme and can potentially be purchased together in a single transaction. We extend on traditional approaches that use mostly transactional data by incorporating both domain knowledge from product suppliers in the form of hierarchies, as well as textual attributes from the products. Our approach starts by combining product hierarchies together with transactional data or domain knowledge to identify candidate sets of product collections. Then, it generates the product collection recommendations from these candidate sets by learning a deep similarity model that leverages textual attributes. Experimental evaluation on real data from the Home Depot online retailer shows that the proposed solution can recommend collections of products with increased accuracy when compared to expert-crafted collections.
更多查看译文
关键词
bundle recommendation, online product recommendation, product taxonomies, text embeddings for recommender systems
AI 理解论文
溯源树
样例
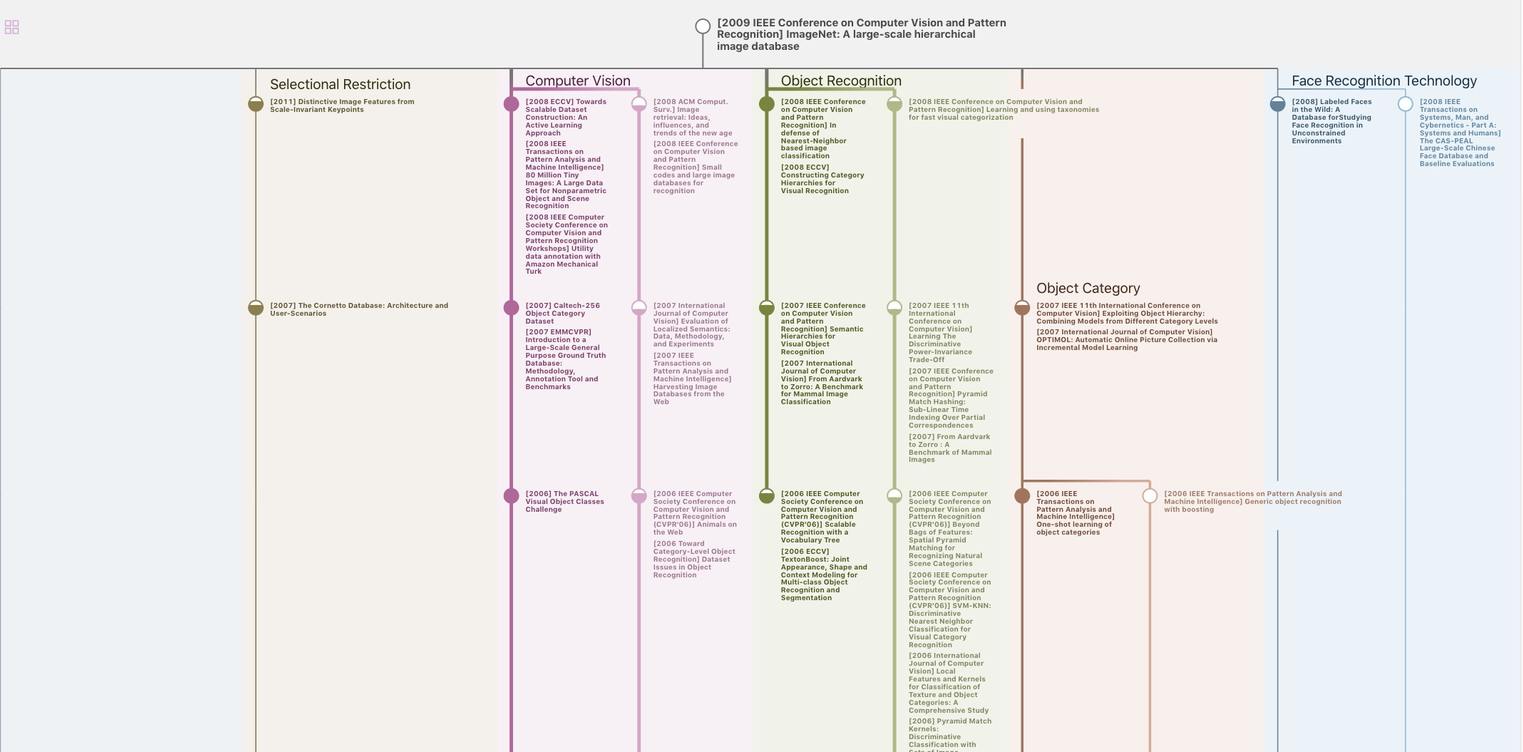
生成溯源树,研究论文发展脉络
Chat Paper
正在生成论文摘要