Analog Neural Networks With Deep-Submicrometer Nonlinear Synapses
IEEE Micro(2019)
摘要
Analog computing is a promising approach to improve the silicon efficiency for inference accelerators in extremely resource-constrained environments. Existing analog circuit proposals for neural networks, however, fall short of realizing the full potential of analog computing because they implement linear synapses, leading to circuits that are either area inefficient or vulnerable to process variation. In this paper, we first present a novel nonlinear analog synapse circuit design that is dense and inherently less sensitive to process variation. We then propose an interpolation-based methodology to train nonlinear synapses built with deep-submicrometer transistors. Our analog neural network achieves a 29× and 582× improvement in computational density relative to state-of-the-art digital and analog inference accelerators, respectively.
更多查看译文
关键词
Synapses,Training,Mathematical model,Neurons,Transistors,Fabrication,Biological neural networks
AI 理解论文
溯源树
样例
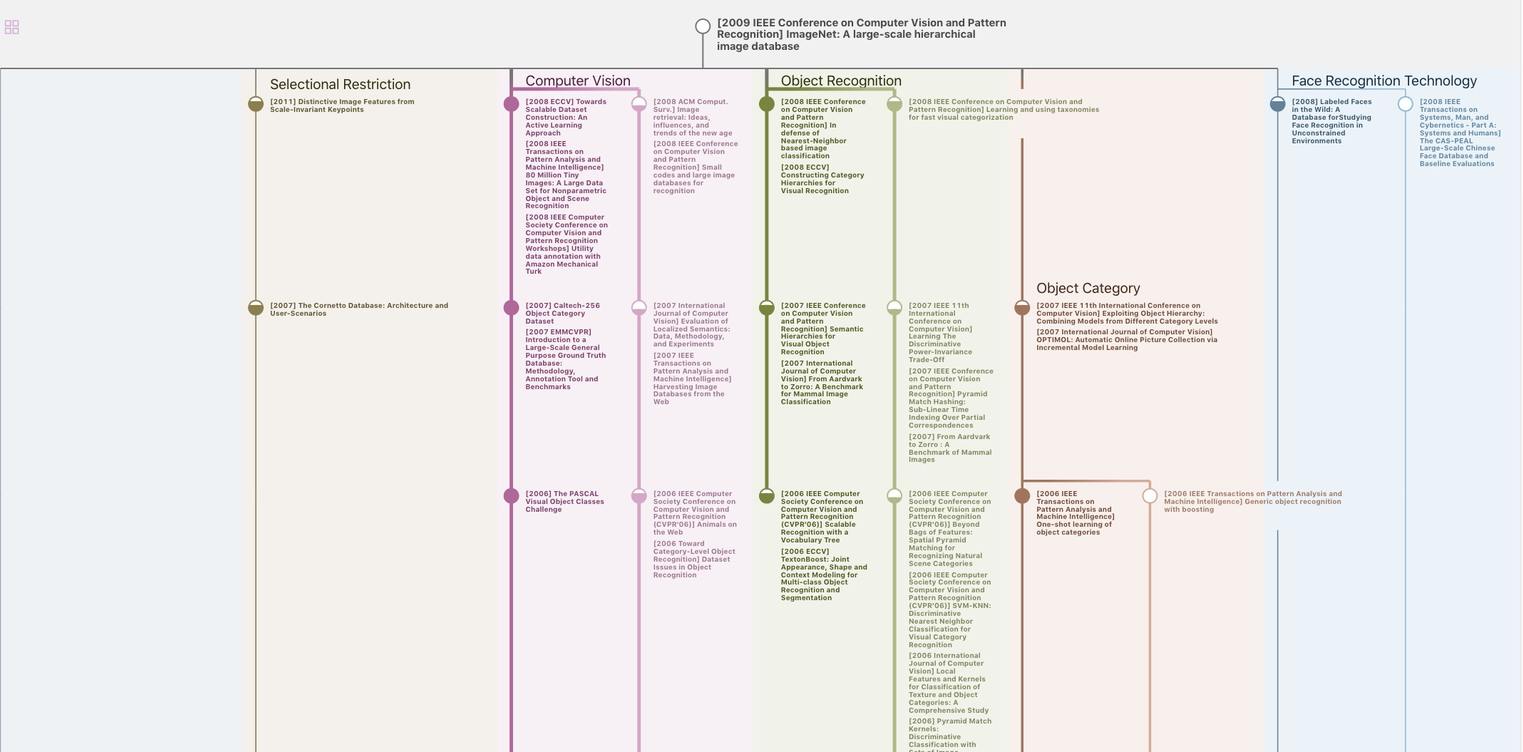
生成溯源树,研究论文发展脉络
Chat Paper
正在生成论文摘要