Multi-Feature Sparse Representation Classification Method Based on Clustering
Proceedings of the 2019 International Conference on Artificial Intelligence and Computer Science(2019)
摘要
In complex environments, the point cloud data obtained by LiDAR Often have shadows and occlusion, which greatly reduces the accuracy and the robustness of target classification. To solve this problem, this paper proposes a robust LiDAR point cloud recognition method, called Multi-Feature Sparse Representation Classification based on Clustering (MFSRCC). Firstly, all training data are used to generate a 3D-SIFT multi-feature dictionary. Secondly, the data are reconstructed on the basis of a complete dictionary. Finally, the sparse coefficients are clustered by K-means, and hence the classifier is constructed according to the principle of minimum cluster center value. The experimental results performed on Large-Scale Point Cloud Classification benchmark show that the proposed method can significantly improve the recognition rate of LiDAR point cloud objects, and it has strong robustness to interference information.
更多查看译文
关键词
3D-SIFT, LiDAR, Object identification, Sparse representation
AI 理解论文
溯源树
样例
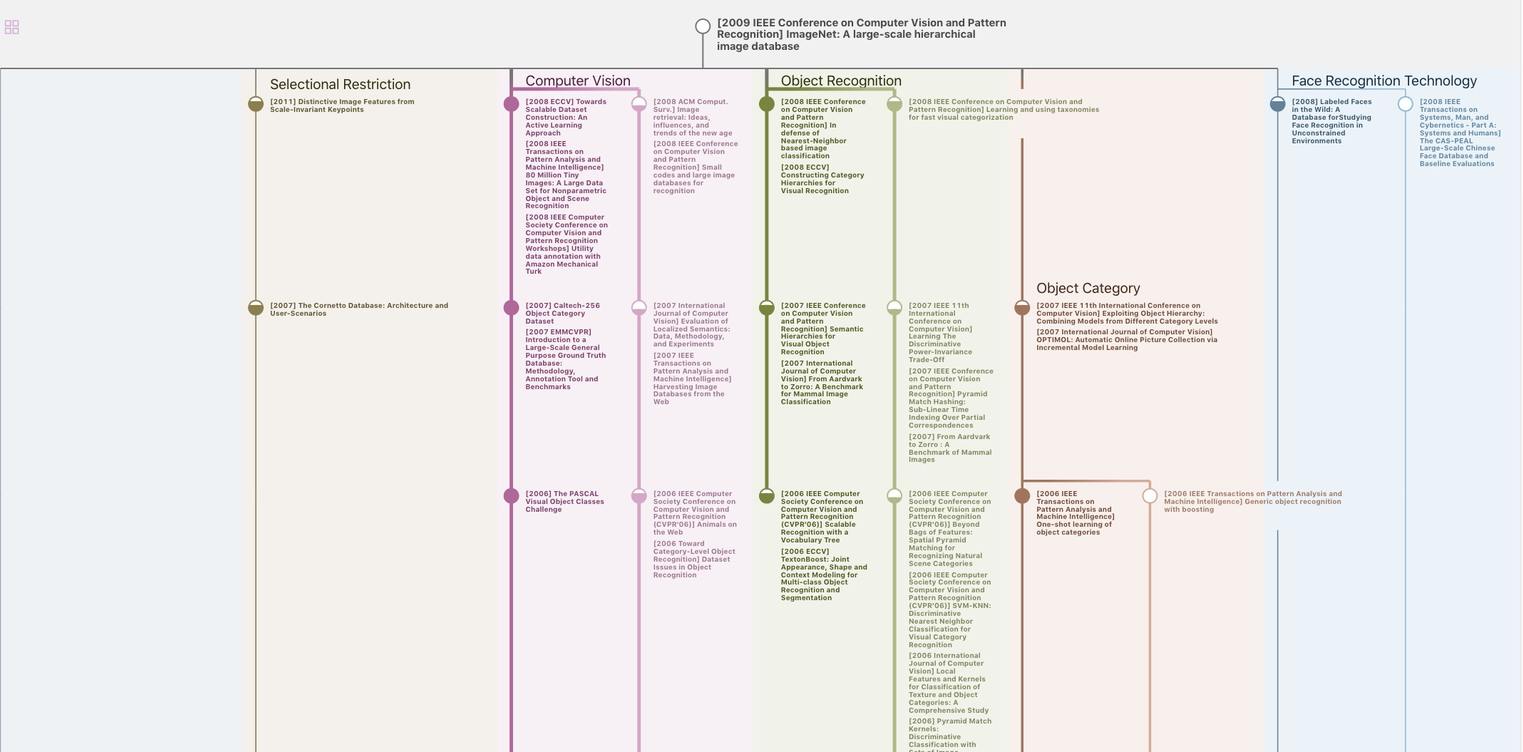
生成溯源树,研究论文发展脉络
Chat Paper
正在生成论文摘要