Joint Monocular 3d Vehicle Detection And Tracking
2019 IEEE/CVF INTERNATIONAL CONFERENCE ON COMPUTER VISION (ICCV 2019)(2019)
摘要
Vehicle 3D extents and trajectories are critical cues for predicting the future location of vehicles and planning future agent ego-motion based on those predictions. In this paper, we propose a novel online framework for 3D vehicle detection and tracking from monocular videos. The framework can not only associate detections of vehicles in motion over time, but also estimate their complete 3D bounding box information from a sequence of 2D images captured on a moving platform. Our method leverages 3D box depth-ordering matching for robust instance association and utilizes 3D trajectory prediction for re-identification of occluded vehicles. We also design a motion learning module based on an LSTM for more accurate long-term motion extrapolation. Our experiments on simulation, KITTI, and Argoverse datasets show that our 3D tracking pipeline offers robust data association and tracking. On Argoverse, our image-based method is significantly better for tracking 3D vehicles within 30 meters than the LiDAR-centric baseline methods.
更多查看译文
关键词
data association,image-based method,online framework,monocular videos,3D box depth-ordering matching,instance association,motion learning module,monocular 3D vehicle detection,monocular 3D vehicle tracking,agent ego-motion,3D bounding box information,3D trajectory prediction,motion extrapolation,LSTM
AI 理解论文
溯源树
样例
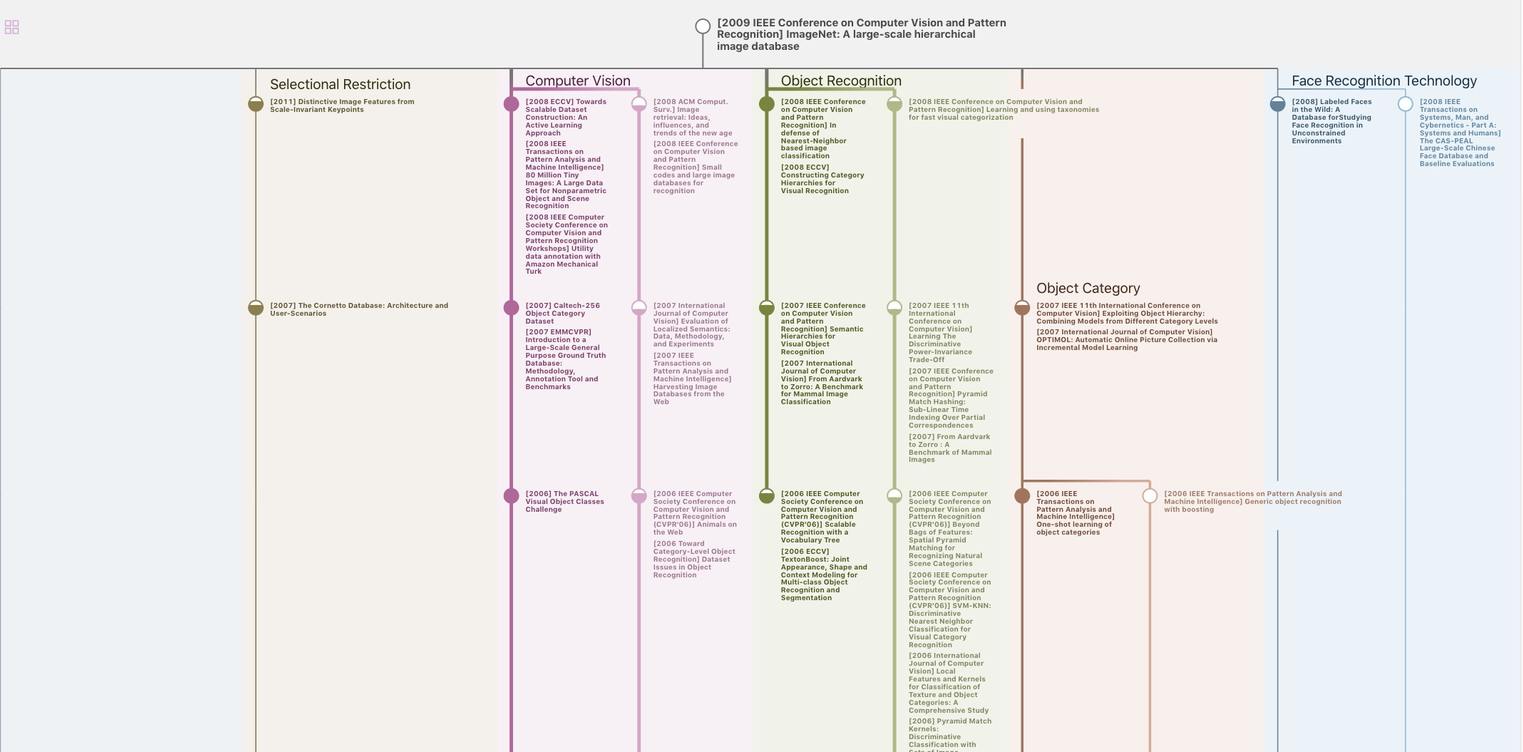
生成溯源树,研究论文发展脉络
Chat Paper
正在生成论文摘要