Feedback Learning for Improving the Robustness of Neural Networks
CoRR(2019)
摘要
Recent research studies revealed that neural networks are vulnerable to adversarial attacks. State-of-the-art defensive techniques add various adversarial examples in training to improve models' adversarial robustness. However, these methods are not universal and can't defend unknown or non-adversarial evasion attacks. In this paper, we analyze the model robustness in the decision space. A feedback learning method is then proposed, to understand how well a model learns and to facilitate the retraining process of remedying the defects. The evaluations according to a set of distance-based criteria show that our method can significantly improve models' accuracy and robustness against different types of evasion attacks. Moreover, we observe the existence of inter-class inequality and propose to compensate for it by changing the proportions of examples generated in different classes.
更多查看译文
关键词
robustness,neural networks,decision space,evasion attacks,feedback learning
AI 理解论文
溯源树
样例
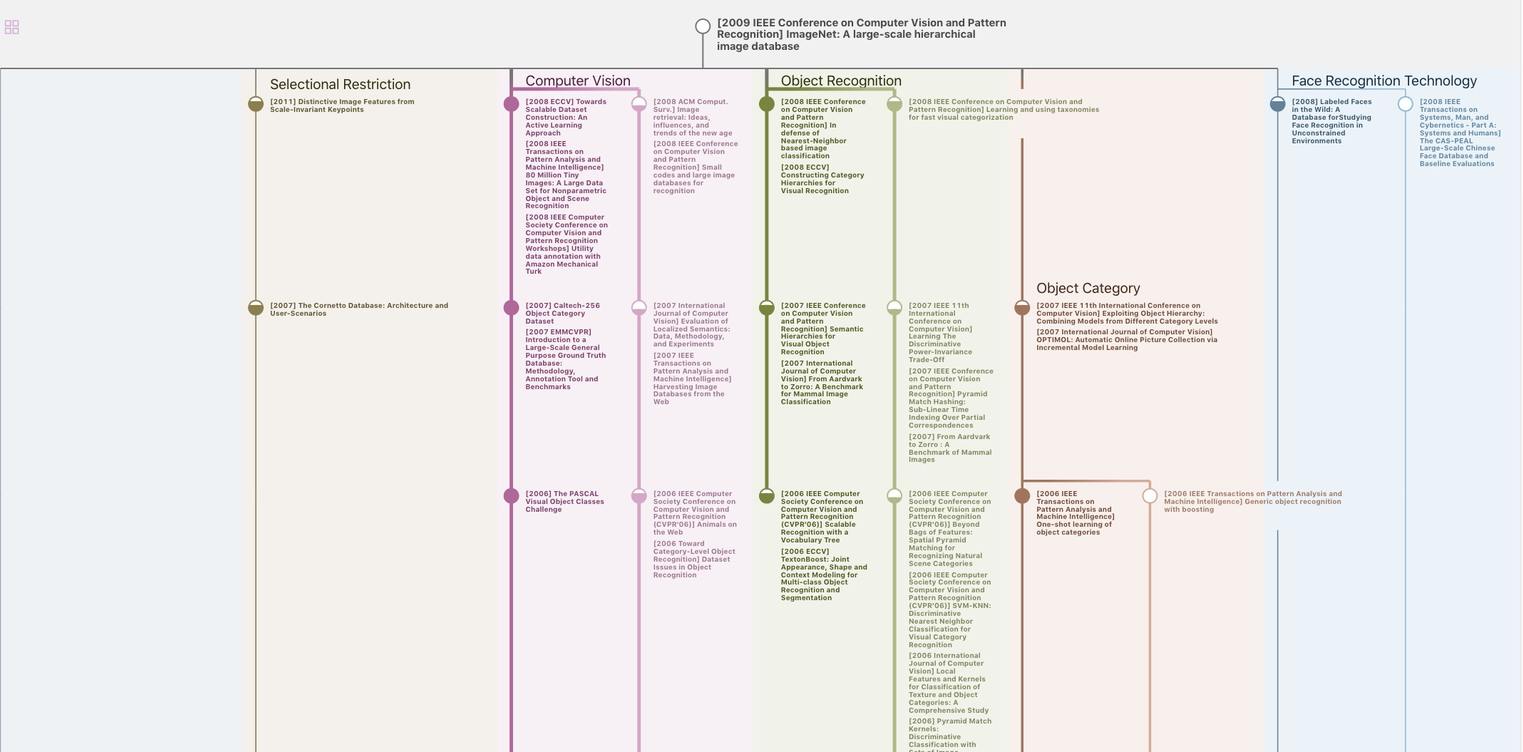
生成溯源树,研究论文发展脉络
Chat Paper
正在生成论文摘要