Weighted Broad Learning System and Its Application in Nonlinear Industrial Process Modeling
IEEE Transactions on Neural Networks and Learning Systems(2020)
摘要
Broad learning system (BLS) is a novel neural network with effective and efficient learning ability. BLS has attracted increasing attention from many scholars owing to its excellent performance. This article proposes a weighted BLS (WBLS) based on BLS to tackle the noise and outliers in an industrial process. WBLS provides a unified framework for easily using different methods of calculating the weighted penalty factor. Using the weighted penalty factor to constrain the contribution of each sample to modeling, the normal and abnormal samples were allocated higher and lower weights to increase and decrease their contributions, respectively. Hence, the WBLS can eliminate the bad effect of noise and outliers on the modeling. The weighted ridge regression algorithm is used to compute the algorithm solution. Weighted incremental learning algorithms are also developed using the weighted penalty factor to tackle the noise and outliers in the additional samples and quickly increase nodes or samples without retraining. The proposed weighted incremental learning algorithms provide a unified framework for using different methods of computing weights. We test the feasibility of the proposed algorithms on some public data sets and a real-world application. Experiment results show that our method has better generalization and robustness.
更多查看译文
关键词
Broad learning system (BLS),incremental learning algorithm,noise and outliers,weighted penalty factor
AI 理解论文
溯源树
样例
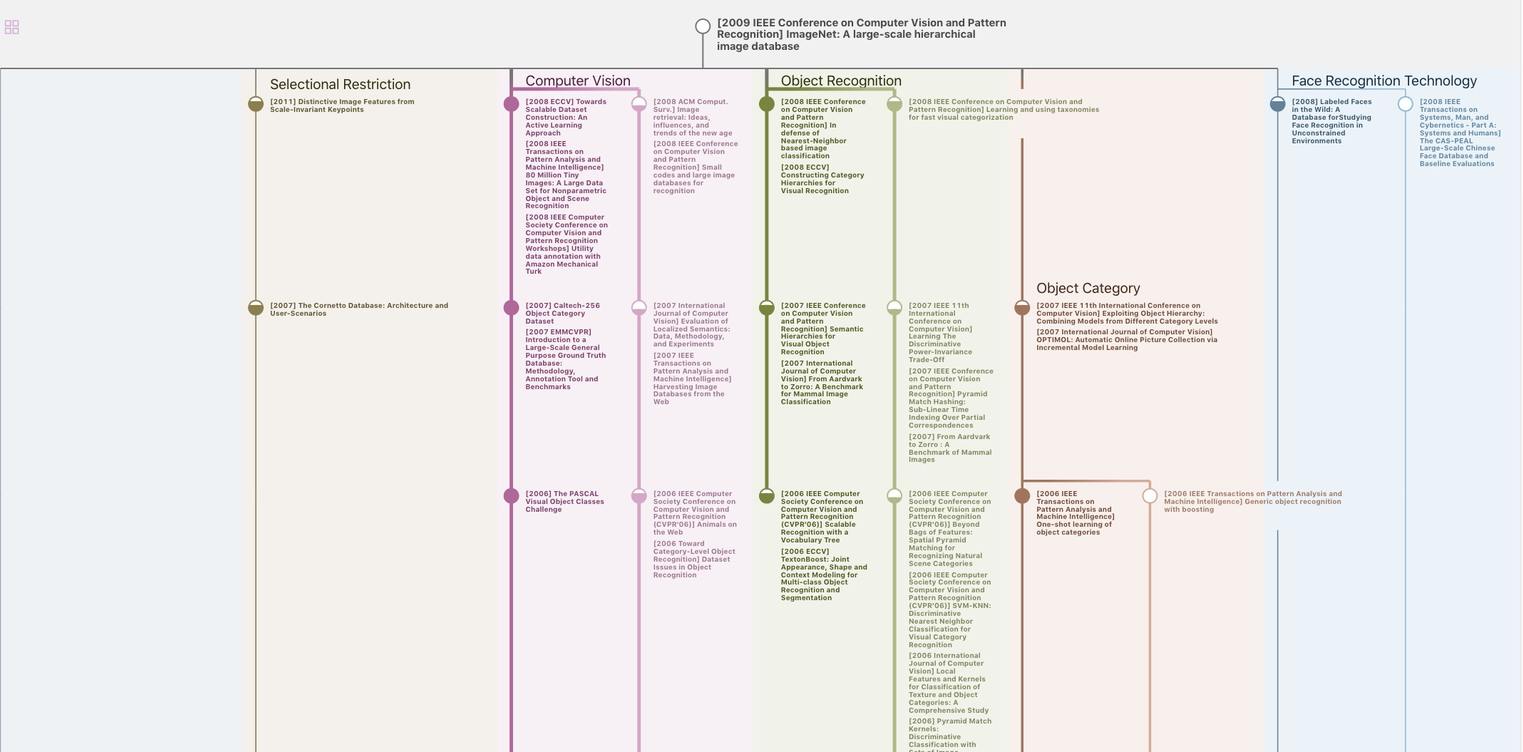
生成溯源树,研究论文发展脉络
Chat Paper
正在生成论文摘要