Machine-Learning Prediction of Tumor Antigen Immunogenicity in the Selection of Therapeutic Epitopes.
CANCER IMMUNOLOGY RESEARCH(2019)
摘要
Current tumor neoantigen calling algorithms primarily rely on epitope/major histocompatibility complex (MHC) binding affinity predictions to rank and select for potential epitope targets. These algorithms do not predict for epitope immunogenicity using approaches modeled from tumor-specific antigen data. Here, we describe peptide-intrinsic biochemical features associated with neoantigen and minor histocompatibility mismatch antigen immunogenicity and present a gradient boosting algorithm for predicting tumor antigen immunogenicity. This algorithm was validated in two murine tumor models and demonstrated the capacity to select for therapeutically active antigens. Immune correlates of neoantigen immunogenicity were studied in a pan-cancer data set from The Cancer Genome Atlas and demonstrated an association between expression of immunogenic neoantigens and immunity in colon and lung adenocarcinomas. Lastly, we present evidence for expression of an out-of-frame neoantigen that was capable of driving antitumor cytotoxic T-cell responses. With the growing clinical importance of tumor vaccine therapies, our approach may allow for better selection of therapeutically relevant tumor-specific antigens, including nonclassic out-of-frame antigens capable of driving antitumor immunity.
更多查看译文
关键词
tumor antigen immunogenicity,machine-learning machine-learning,prediction
AI 理解论文
溯源树
样例
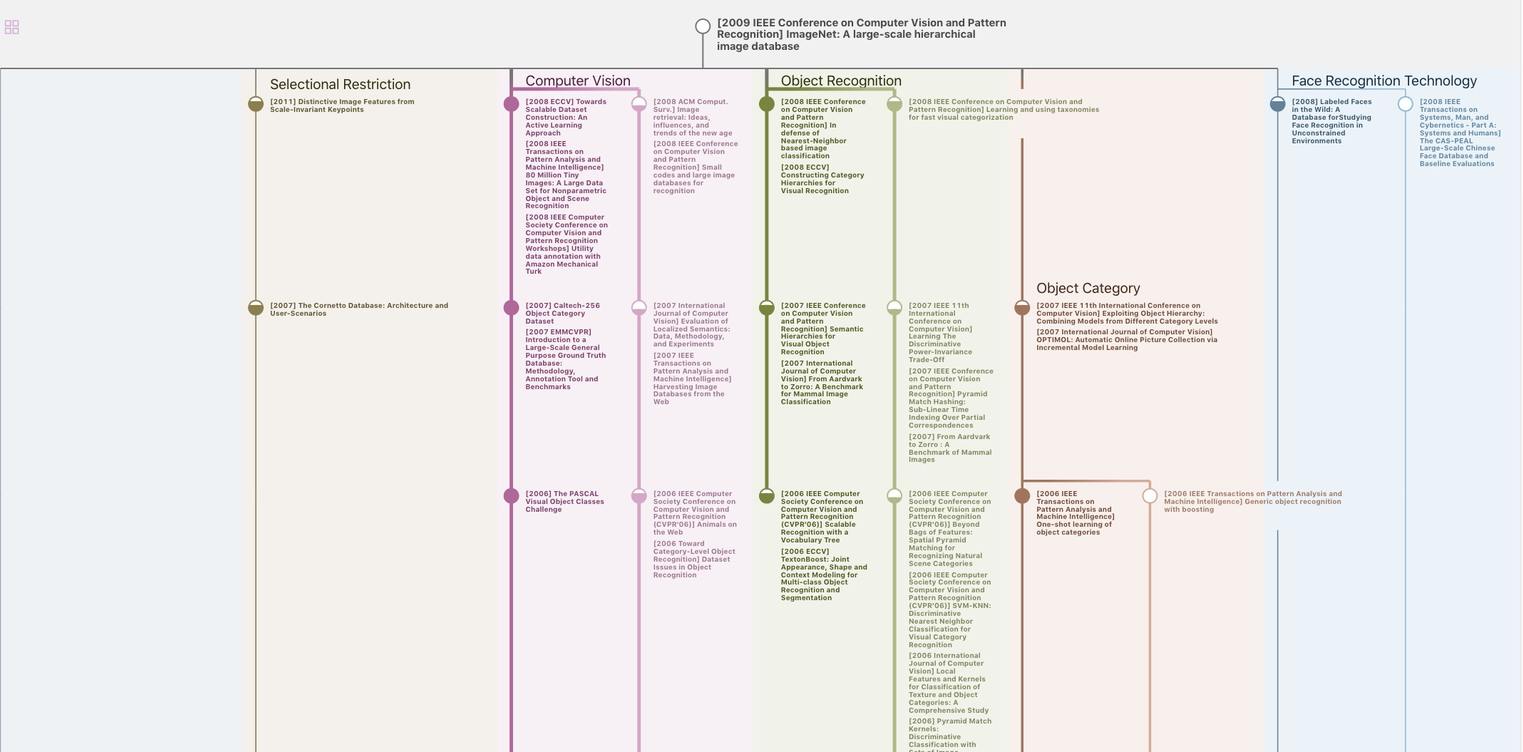
生成溯源树,研究论文发展脉络
Chat Paper
正在生成论文摘要