Automated Robot Skill Learning From Demonstration For Various Robot Systems
ADVANCES IN ARTIFICIAL INTELLIGENCE, KI 2019(2019)
摘要
Transferring human movements to robotic systems is of high interest to equip the systems with new behaviors without expert knowledge. Typically, skills are often only learned for a very specific setup and a certain robot. We propose a modular framework to learn skills that is applicable on different robotic systems without adaptations. Our work builds on the recently introduced BesMan Learning Platform, which comprises the full workflow to transfer human demonstrations to a system, including automatized behavior segmentation, imitation learning, reinforcement learning for motion refinement, and methods to generalize to related tasks. For this paper, we extend this approach in order that different skills can be imitated by various systems in an automated fashion with a minimal amount of configuration, e.g., definition of the target system and environment. For this, we focus on the imitation of the demonstrated movements and show their transferability without movement refinement. We demonstrate the generality of the approach on a large dataset, consisting of about 700 throwing demonstrations. Nearly all of these human demonstrations are successfully transferred to four different robot target systems, namely Universal Robot's UR5 and UR10, KUKA LBR iiwa, and DFKI's robot COMPI. An analysis of the quality of the imitated movement on the real UR5 robot shows that useful throws can be executed on the system which can be used as starting points for further movement refinement.
更多查看译文
关键词
Behavior learning, Learning from demonstration, Behavior segmentation, Imitation learning, Transfer learning, Manipulation, Robotics
AI 理解论文
溯源树
样例
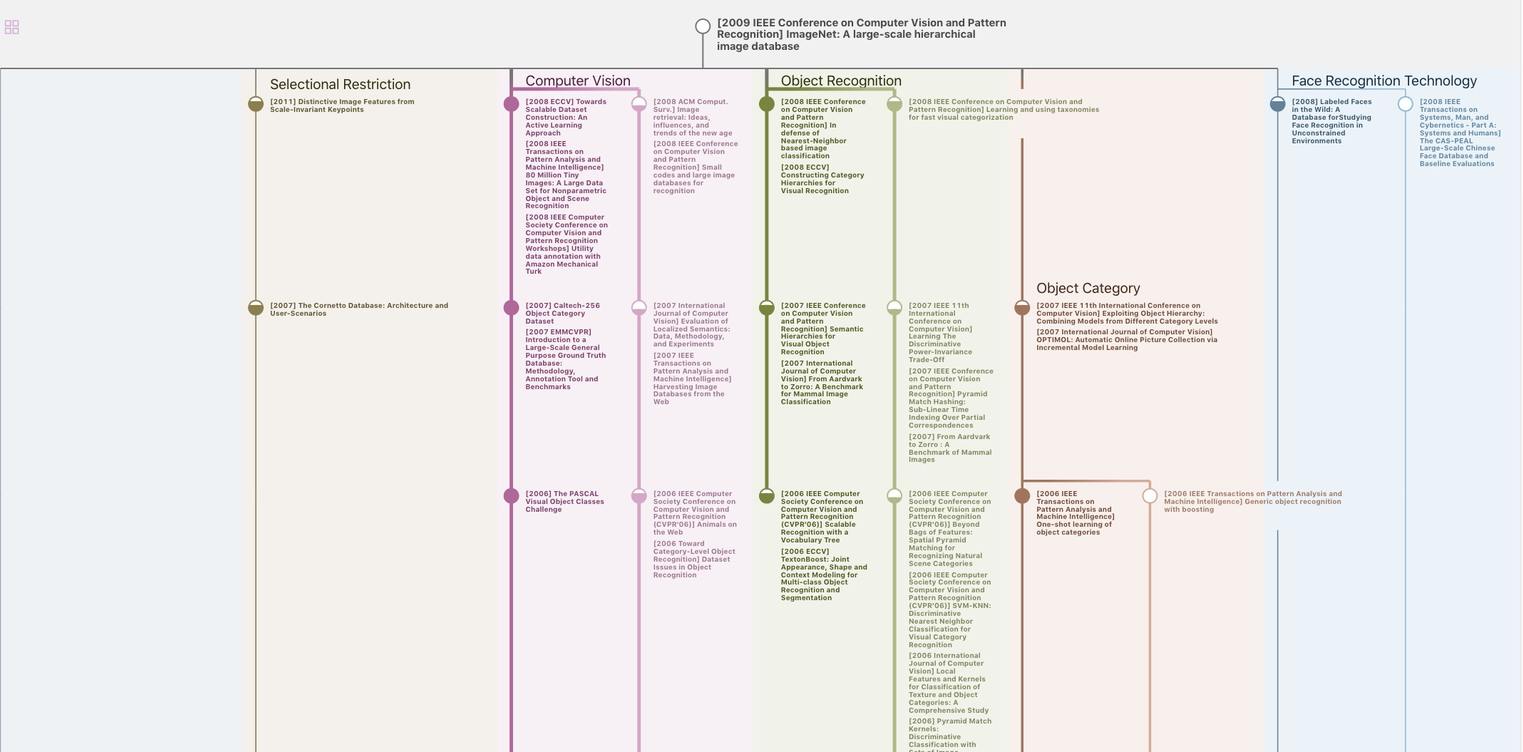
生成溯源树,研究论文发展脉络
Chat Paper
正在生成论文摘要