Brain-Like Object Recognition with High-Performing Shallow Recurrent ANNs
ADVANCES IN NEURAL INFORMATION PROCESSING SYSTEMS 32 (NIPS 2019)(2019)
摘要
Deep convolutional artificial neural networks (ANNs) are the leading class of candidate models of the mechanisms of visual processing in the primate ventral stream. While initially inspired by brain anatomy, over the past years, these ANNs have evolved from a simple eight-layer architecture in AlexNet to extremely deep and branching architectures, demonstrating increasingly better object categorization performance, yet bringing into question how brain-like they still are. In particular, typical deep models from the machine learning community are often hard to map onto the brain's anatomy due to their vast number of layers and missing biologically-important connections, such as recurrence. Here we demonstrate that better anatomical alignment to the brain and high performance on machine learning as well as neuroscience measures do not have to be in contradiction. We developed CORnetS , a shallow ANN with four anatomically mapped areas and recurrent connectivity, guided by Brain-Score, a new large-scale composite of neural and behavioral benchmarks for quantifying the functional fidelity of models of the primate ventral visual stream. Despite being significantly shallower than most models, CORnetS is the top model on Brain-Score and outperforms similarly compact models on ImageNet. Moreover, our extensive analyses of CORnetS circuitry variants reveal that recurrence is the main predictive factor of both Brain-Score and ImageNet top-1 performance. Finally, we report that the temporal evolution of the CORnetS "IT" neural population resembles the actual monkey IT population dynamics. Taken together, these results establish CORnetS , a compact, recurrent ANN, as the current best model of the primate ventral visual stream. 33rd Conference on Neural Information Processing Systems (NeurIPS 2019), Vancouver, Canada. 0 .2 .4 .6 .8 ImageNet top-1 performance .25 .3 .35 .4 .45 Brain-Score r = .90 .7 .75 .8 .85 ImageNet top-1 performance .37 .38 .39 .4 .41 .42 Brain-Score r: n.s. Figure 1: Synergizing machine learning and neuroscience through Brain-Score (top). By quantifying brain-likeness of models, we can compare models of the brain and use insights gained to inform the next generation of models. Green dots represent popular deep neural networks while gray dots correspond to various exemplary small-scale models (BaseNets) that demonstrate the relationship between ImageNet performance and Brain-Score on a wider range of performances (see Section 4.1). CORnetS is the current best model on Brain-Score. CORnetS area circuitry (bottom left). The model consists of four areas which are pre-mapped to cortical areas V1, V2, V4, and IT in the ventral stream. V1 COR is feed-forward and acts as a pre-processor to reduce the input complexity. V2 COR , V4 COR and IT COR are recurrent (within area). See Section 2.1 for details.
更多查看译文
关键词
object recognition,population dynamics,top model,visual processing,brain anatomy
AI 理解论文
溯源树
样例
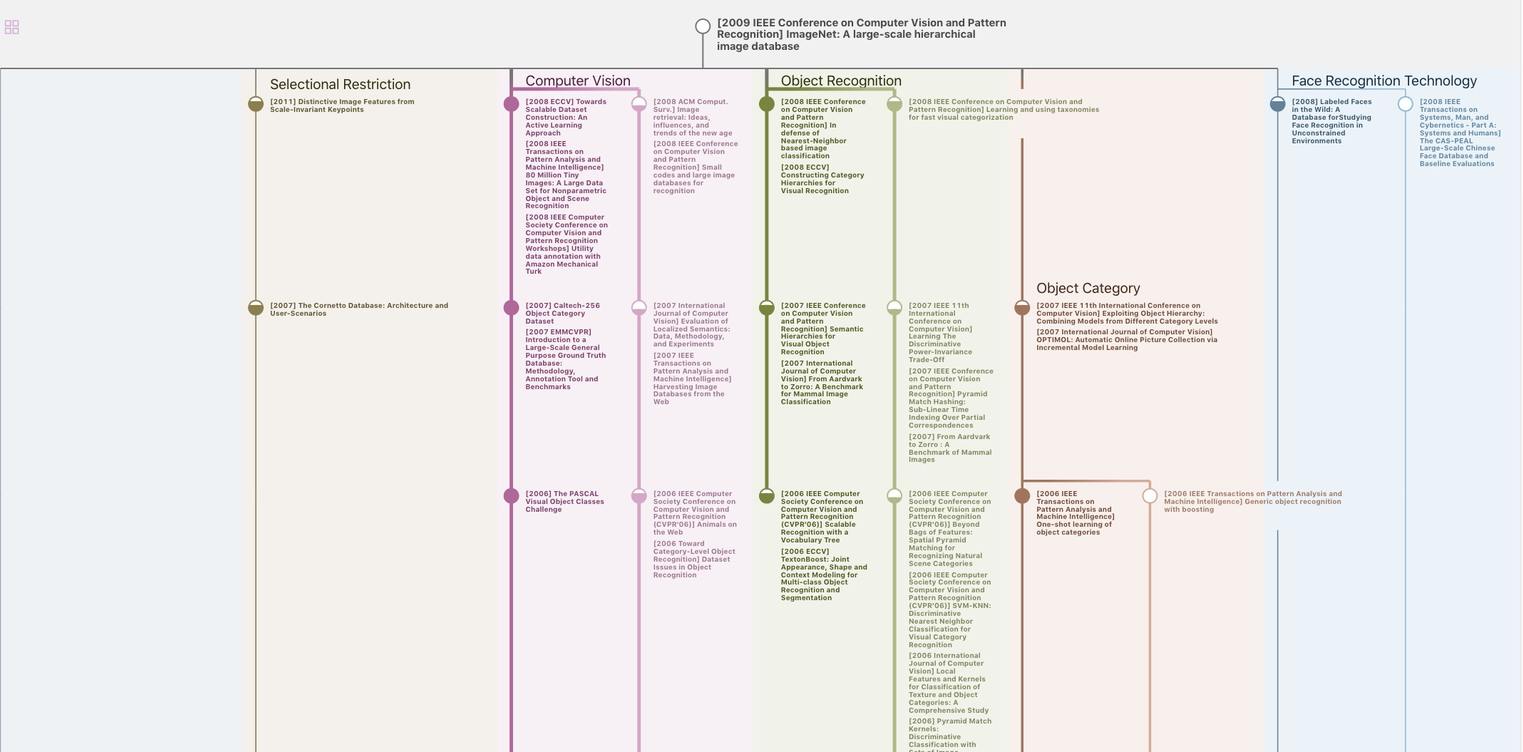
生成溯源树,研究论文发展脉络
Chat Paper
正在生成论文摘要