Predicting gene regulatory interactions based on spatial gene expression data and deep learning.
PLOS COMPUTATIONAL BIOLOGY(2019)
摘要
Reverse engineering of gene regulatory networks (GRNs) is a central task in systems biology. Most of the existing methods for GRN inference rely on gene co-expression analysis or TF-target binding information, where the determination of co-expression is often unreliable merely based on gene expression levels, and the TF-target binding data from high-throughput experiments may be noisy, leading to a high ratio of false links and missed links, especially for large-scale networks. In recent years, the microscopy images recording spatial gene expression have become a new resource in GRN reconstruction, as the spatial and temporal expression patterns contain much abundant gene interaction information. Till now, the spatial expression resources have been largely underexploited, and only a few traditional image processing methods have been employed in the image-based GRN reconstruction. Moreover, co-expression analysis using conventional measurements based on image similarity may be inaccurate, because it is the local-pattern consistency rather than global-image-similarity that determines gene-gene interactions. Here we present GripDL (Gene regulatory interaction prediction via Deep Learning), which incorporates high-confidence TF-gene regulation knowledge from previous studies, and constructs GRNs for Drosophila eye development based on Drosophila embryonic gene expression images. Benefitting from the powerful representation ability of deep neural networks and the supervision information of known interactions, the new method outperforms traditional methods with a large margin and reveals new intriguing knowledge about Drosophila eye development.
更多查看译文
关键词
spatial gene expression data,gene expression,regulatory interactions,deep learning
AI 理解论文
溯源树
样例
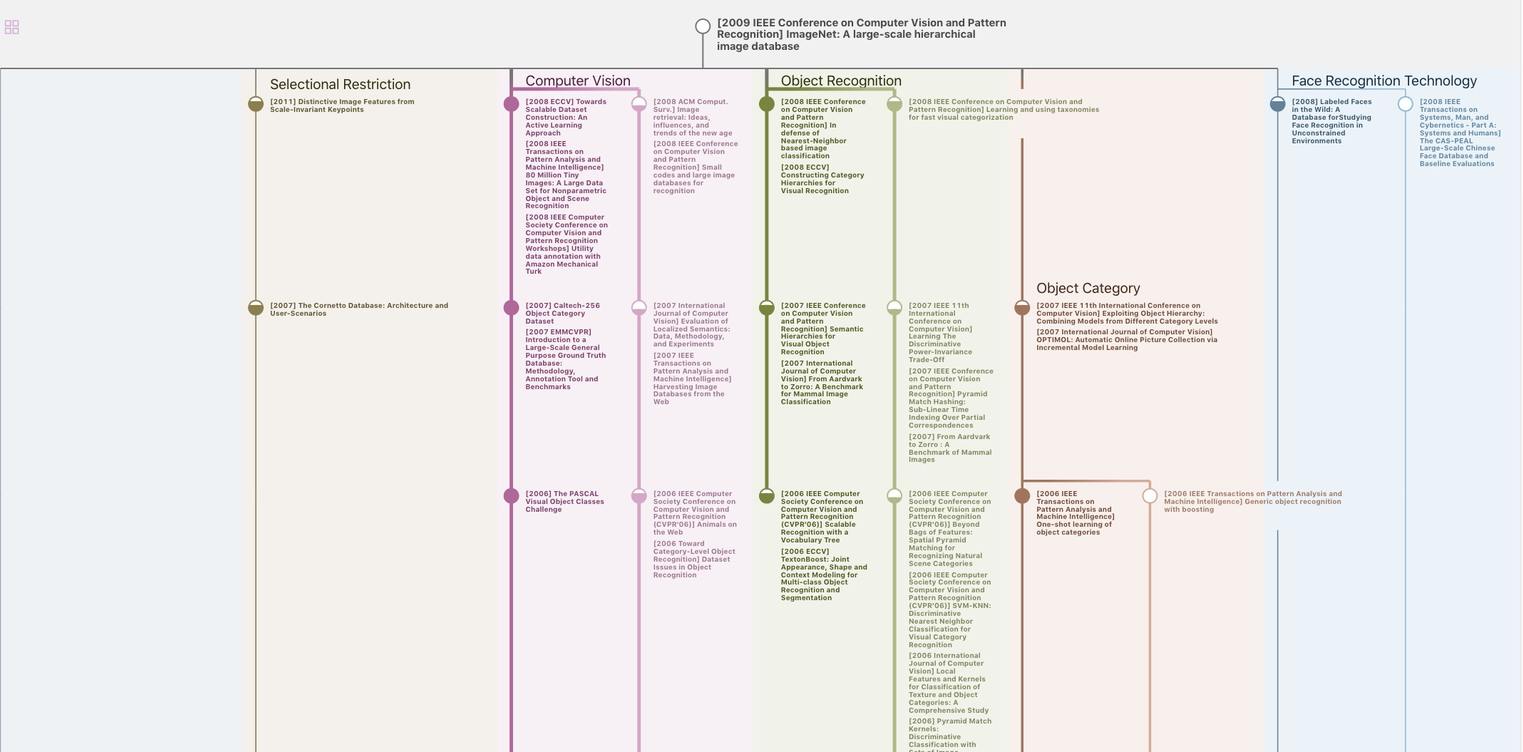
生成溯源树,研究论文发展脉络
Chat Paper
正在生成论文摘要