An Unsupervised Drift Detector For Online Imbalanced Evolving Streams
PROCEEDINGS OF THE 8TH INTERNATIONAL CONFERENCE ON DATA SCIENCE, TECHNOLOGY AND APPLICATIONS (DATA)(2019)
摘要
Detecting concept drift from an imbalanced evolving stream is challenging task. At high degree of imbalance ratios, the poor or nil performance estimates of the learner from minority class tends to drift detection failures. To ameliorate this problem, we propose a new drift detection and adaption framework. Proposed drift detection mechanism is carried out in two phases includes unsupervised and supervised drift detection with queried labels. The adaption framework is based on the batch wise active learning. Comparative results on four synthetic and one real world balanced and imbalanced evolving streams with other prominent drift detection methods indicates that our approach is better in detecting the drift with low false positive rates.
更多查看译文
关键词
Class Imbalance, Evolving Stream, Concept Drift, Active Learning, Hypothesis Tests
AI 理解论文
溯源树
样例
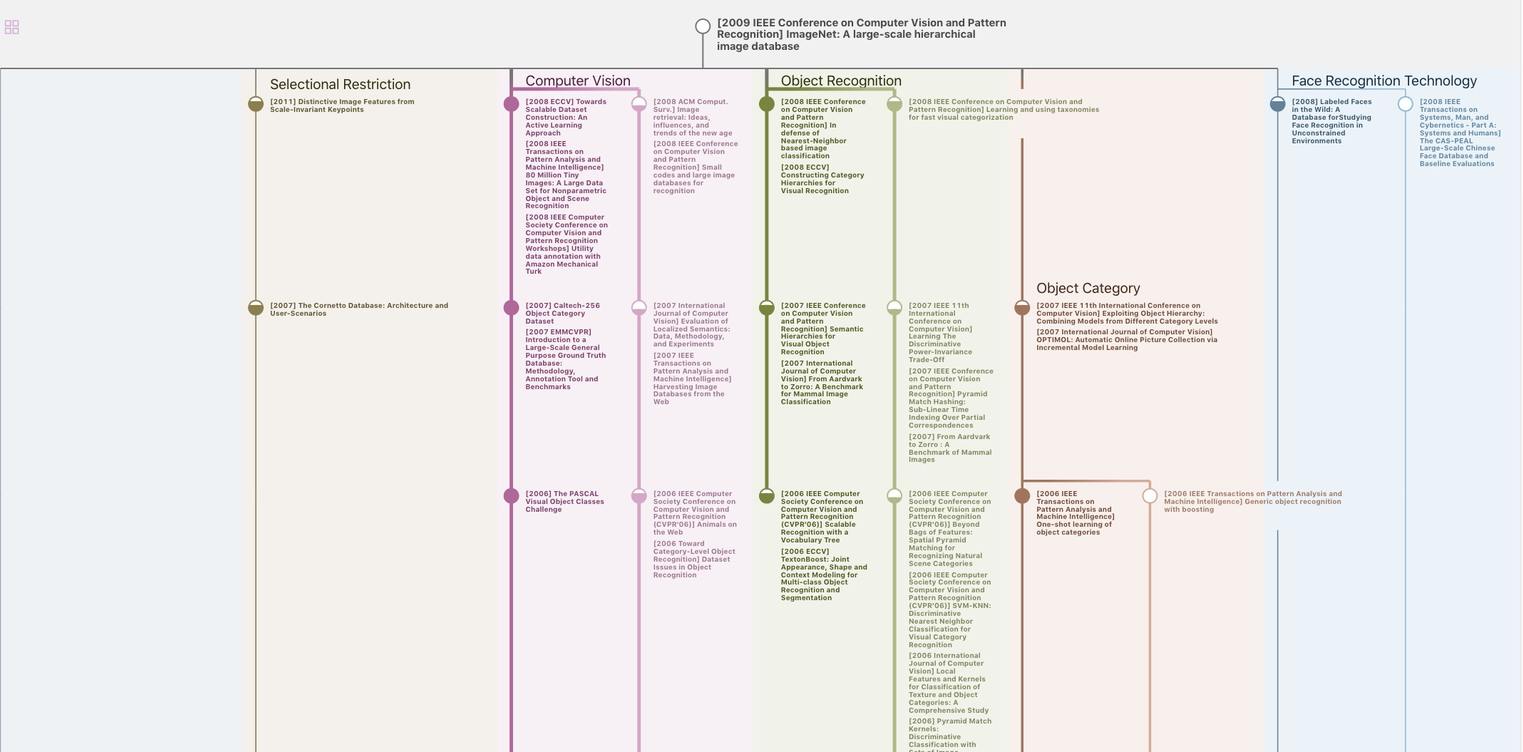
生成溯源树,研究论文发展脉络
Chat Paper
正在生成论文摘要