Effective and Efficient Variable-Length Data Series Analytics.
PhD@VLDB(2019)
摘要
In the last twenty years, data series similarity search has emerged as a fundamental operation at the core of several analysis tasks and applications related to data series collections. Many solutions to different mining problems work by means of similarity search. In this regard, all the proposed solutions require the prior knowledge of the series length on which similarity search is performed. In several cases, the choice of the length is critical and sensibly influences the quality of the expected outcome. Unfortunately, the obvious brute-force solution, which provides an outcome for all lengths within a given range is computationally untenable. In this Ph.D. work, we present the first solutions that inherently support scalable and variable-length similarity search in data series, applied to sequence/subsequences matching, motif and discord discovery problems.The experimental results show that our approaches are up to orders of magnitude faster than the alternatives. They also demonstrate that we can remove the unrealistic constraint of performing analytics using a predefined length, leading to more intuitive and actionable results, which would have otherwise been missed.
更多查看译文
关键词
series,data,variable-length
AI 理解论文
溯源树
样例
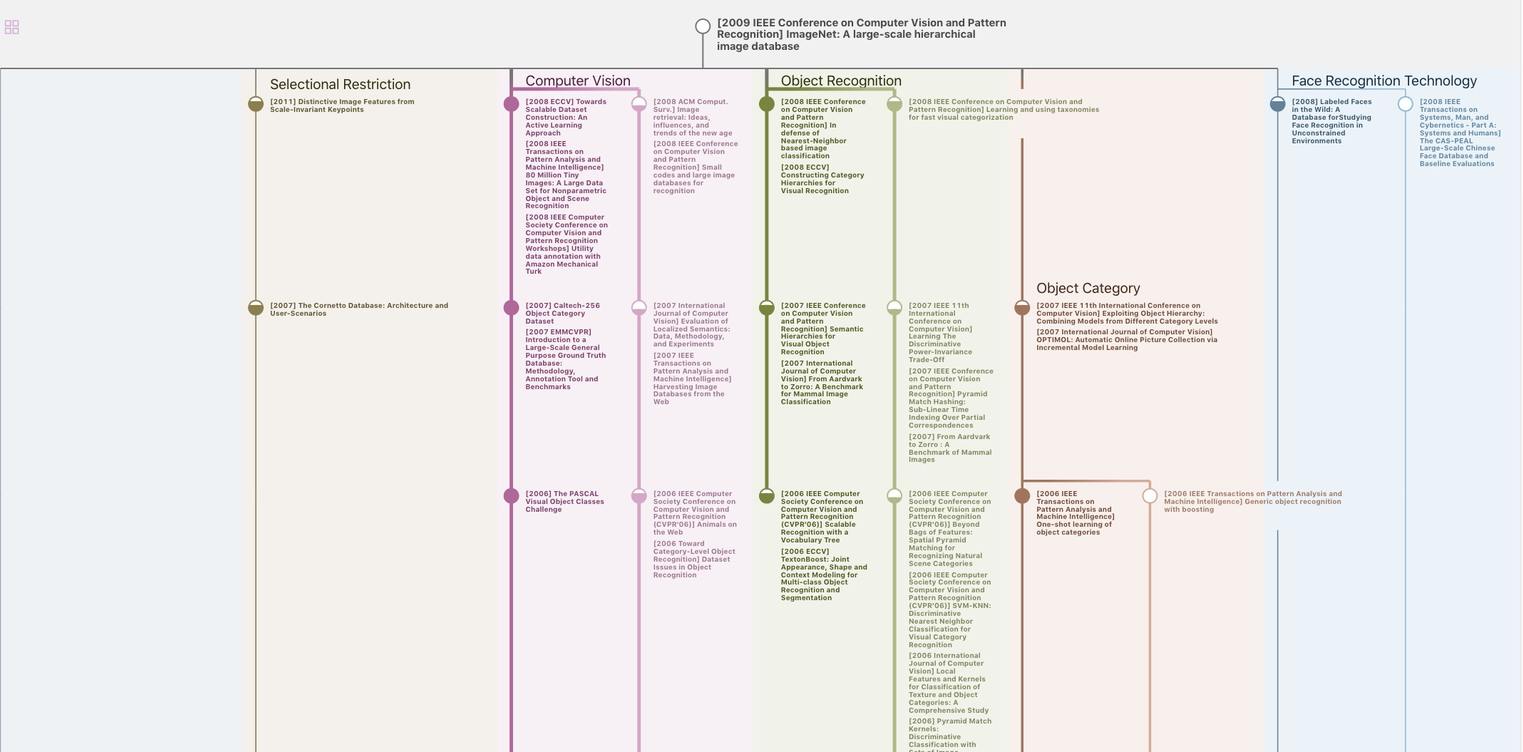
生成溯源树,研究论文发展脉络
Chat Paper
正在生成论文摘要