Predicting G-quadruplexes from DNA Sequences Using Multi-Kernel Convolutional Neural Networks
BCB(2019)
摘要
G-quadruplexes are nucleic acid secondary structures that form within guanine-rich DNA sequences. G-quadruplex formation can affect chromatin architecture and gene regulation and has been associated with genomic instability, genetic diseases and cancer progression. Here, we present a new method, called G4detector, to predict G-quadruplexes from DNA sequences based on multi-kernel convolutional neural networks. The code and benchmarks are publicly available on \urlgithub.com/OrensteinLab/G4detector. As part of this study, we generated novel benchmarks to train and test different computational methods for the task of G4 prediction. We used the high-throughputin vitro data generated by the G4-seq protocol %(Chamberset al. 2015). ~\citechambers2015high. We turned each of the three sets into a classification problem by augmenting it with a negative set, using three types of negatives: \beginenumerate \item random : random genomic sequences \item dishuffle : randomly shuffled positives while preserving dinucleotide frequencies \item PQ : predicted G-quadruplexes in the human genome according to a regular the expression: \beginequation [G]^3+ [ACGT]^1-7 [G]^3+ [ACGT]^1-7 [G]^3+ [ACGT]^1-7 [G]^3+ \endequation \endenumerate We used the genomic coordinates as measured in the G4 ChIP-seq experiment %(H\"a nsel-Hertschet al. 2016) ~\citehansel2016g to create anin vivo benchmark. Since the G4 structures and the loops that comprise them vary in size, using a kernel of fixed size might not be beneficial for identifying the features that characterize a G4 structure. Instead, our method, G4detector, employs three parallel one-dimensional convolution layers. %(Zhanget al. 2018). ~\citezhang2018high. The final output assigns to each input DNA sequence a probability of forming a G4 structure. We compared G4detector to three other methods: GraphProt %(Maticzkaet al. 2014), ~\citematiczka2014graphprot, Quadron %(Sahakyanet al. 2017), ~\citesahakyan2017machine and G4Hunter %(Bedratet al. 2016). ~\citebedrat2016re. According to the results G4detector outperforms all competing methods in predictingin vitro G4 formation as well as in predictingin vivo formation, maintaining the highest area under the receiver operating curve (AUC) score, as summarized in Figure~\reffig:all. \beginfigure [!h] \centering \beginsubfigure [a]0.4\textwidth \includegraphics[width=\columnwidth]invitro_c.png \caption łabelfig:Ng1 \endsubfigure \beginsubfigure [b]0.4\textwidth \includegraphics[width=\columnwidth]invivo_c.png \caption łabelfig:Ng2 \endsubfigure \caption[]G4detector outperforms extant methods in predicting G4 formation (a)in vitro and (b)in vivo. %using three different types of stabilizers and negative sets. łabelfig:all \endfigure
更多查看译文
关键词
G-quadruplex, Convolutional neural networks
AI 理解论文
溯源树
样例
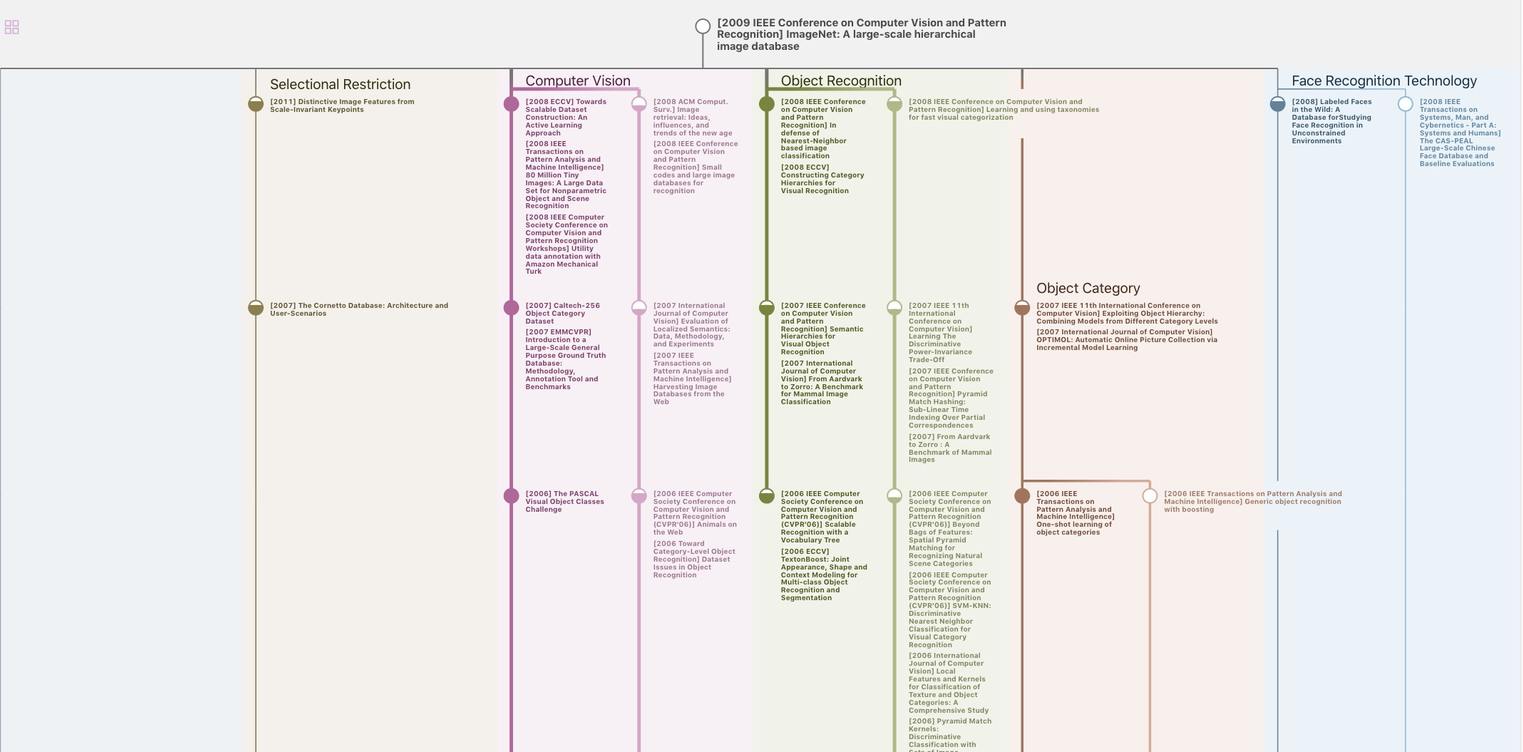
生成溯源树,研究论文发展脉络
Chat Paper
正在生成论文摘要