Document-Level Named Entity Recognition By Incorporating Global And Neighbor Features
INFORMATION RETRIEVAL (CCIR 2019)(2019)
摘要
State-of-the-art named entity recognition models mostly process sentences within a document separately. Sentence-level named entity recognition is easy to cause tagging inconsistency problems for long text documents. In this paper, we first propose to use the neural network to encode global consistency and neighbor relevance among occurrences of a particular token within a document. We first encode sentences within a document independently by a sentence-level BiLSTM layer, then we design a document-level module to encode the relation between occurrences of a particular token. In our document-level module, we use CNN to encode global consistency features and apply BiLSTM to model neighbor relevance features. We further apply a gate to effectively fuse these two non-local features and use a CRF layer to decode labels. We evaluate our model on the CoNLL-2003 dataset. Experimental results show that our model outperforms existing methods.
更多查看译文
关键词
Document-level, Named entity, Global, Neighbor
AI 理解论文
溯源树
样例
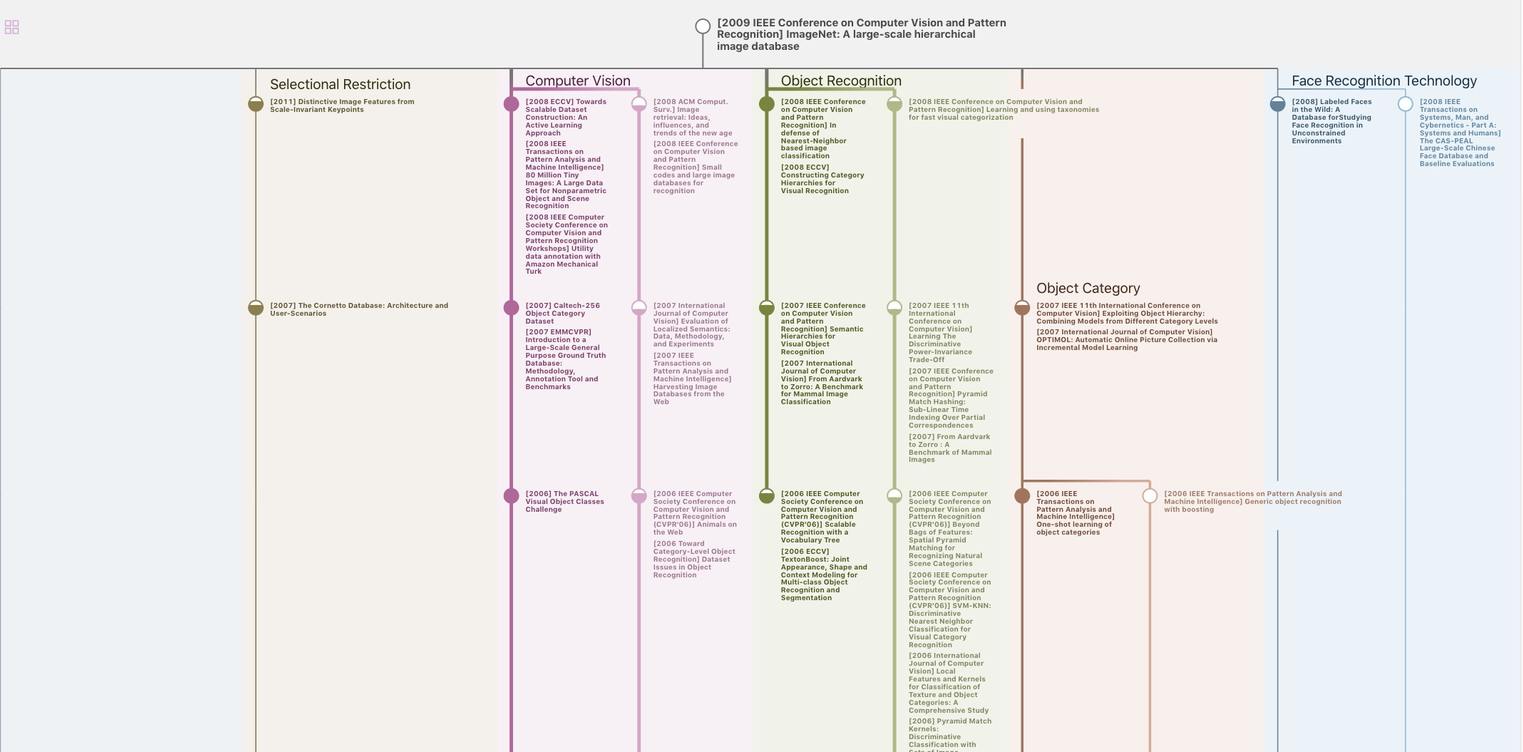
生成溯源树,研究论文发展脉络
Chat Paper
正在生成论文摘要