Simplified Representation Learning Model Based On Parameter-Sharing For Knowledge Graph Completion
INFORMATION RETRIEVAL (CCIR 2019)(2019)
摘要
Knowledge graphs (KG) contain knowledge about the world and provide a structured representation of this knowledge. Current knowledge graphs contain only a small subset of what is true in the world. Knowledge Graph Completion (KGC) task aims to findin missing or errant relationships with the goal of improving the general quality of KGs. Recent years have witnessed great advance of represent learning (RL) based KGC models, which represent entities and relations as elements of a continuous vector space. However, with the deepening of the research, the scale of parameters and the complexity of KGC models become larger and larger, resulting in a serious imbalance between accuracy and computational complexity. Finally, not only the efficiency is not satisfactory, but also the training cost becomes high, which seriously restricts the flexibility and scalability of the KGC model. Therefore, this paper investigates how to enhance the simplicity of KGC model and achieve a reasonable balance between accuracy and complexity. Extensive experiments show that the proposed framework improves the performance of the current represent learning models for KGC task.
更多查看译文
关键词
Knowledge Graph Completion, Simplified model, Parameter-sharing, Representation learning
AI 理解论文
溯源树
样例
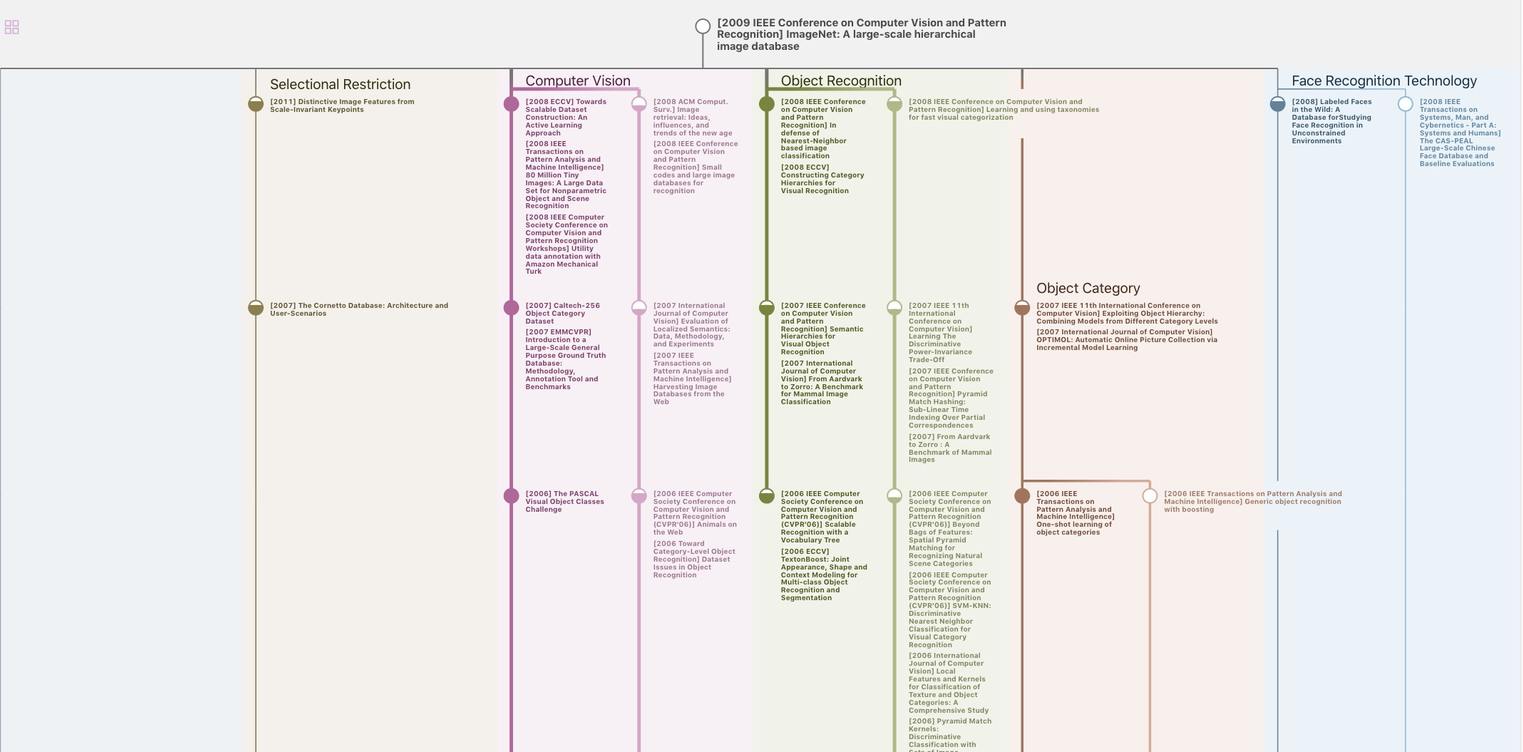
生成溯源树,研究论文发展脉络
Chat Paper
正在生成论文摘要