Heart sound classification using the SNMFNet classifier
PHYSIOLOGICAL MEASUREMENT(2019)
摘要
Objective: Heart sound classification still suffers from the challenges involved in achieving high accuracy in the case of small samples. Dimension reduction attempts to extract low-dimensional features with more discriminability from high-dimensional spaces or raw data, and is popular in learning predictive models that target small sample problems. However, it can also be harmful to classification, because any reduction has the potential to lose information containing category attributes. Approach: For this, a novel SNMFNet classifier is designed to directly associate the dimension reduction process with the classification procedure used for promoting feature dimension reduction to follow the approach that is beneficial for classification, thus making the low-dimensional features more distinguishable and addressing the challenge facing heart sound classification in small samples. Main results: We evaluated our method and representative methods using a public heart sound dataset. The experimental results demonstrate that our method outperforms all comparative models with an obvious improvement in small samples. Furthermore, even if used with relatively sufficient samples, our method performs at least as well as the baseline that uses the same high-dimensional features. Significance: The proposed SNMFNet classifier significantly to improves the small sample problem in heart sound classification.
更多查看译文
关键词
heart sound classification,small samples,semi-non-negative matrix factorization,feature dimension reduction
AI 理解论文
溯源树
样例
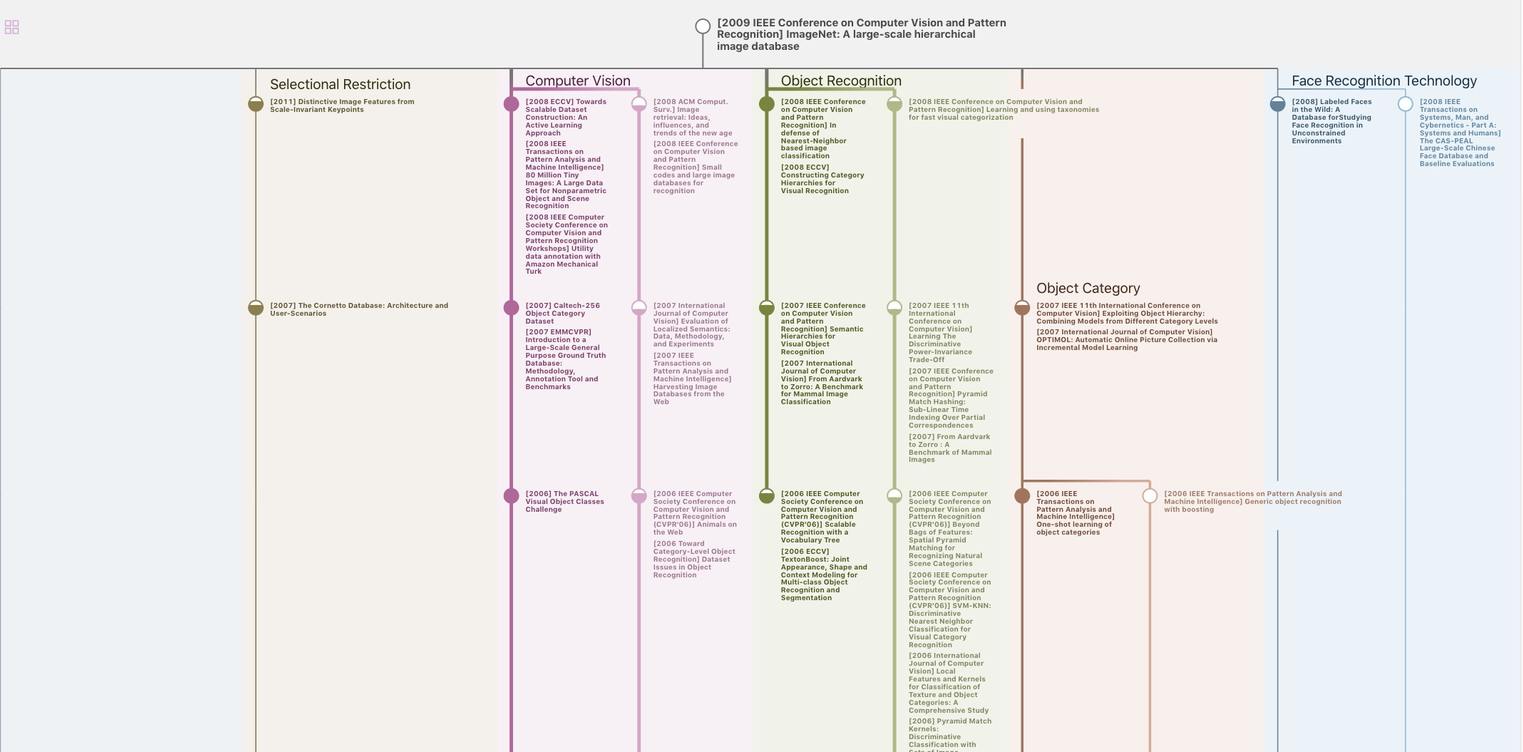
生成溯源树,研究论文发展脉络
Chat Paper
正在生成论文摘要