Adversarial Grasp Objects
2019 IEEE 15th International Conference on Automation Science and Engineering (CASE)(2019)
摘要
Learning-based approaches to robust robot grasp planning can grasp a wide variety of objects, but may be prone to failure on some objects. Inspired by recent results in computer vision, we define a class of “adversarial grasp objects that are physically similar to a given object but significantly less ”graspable” in terms of a specified robot grasping policy. We present three algorithms for synthesizing adversarial grasp objects under the grasp reliability measure of Dex-Net 1.0 for parallel-jaw grippers: 1) two analytic algorithms that perturb vertices on antipodal faces (one that uses random perturbations and one that uses systematic perturbations), and 2) a deep-learning-based approach using a variation of the Cross-Entropy Method (CEM) augmented with a generative adversarial network (GAN) to synthesize classes of adversarial grasp objects represented by discrete Signed Distance Functions. The random perturbation algorithm reduces graspability by 32%, 12%, and 32% for intersected cylinders, intersected prisms, and ShapeNet bottles, respectively, while maintaining shape similarity using geometric constraints. The systematic perturbation algorithm reduces graspability by 32%, 11%, and 21%; and the GAN reduces graspability by 22%, 36%, and 17%, on the same objects. We use the algorithms to generate and 3D print adversarial grasp objects. Simulation and physical experiments confirm that all algorithms are effective at reducing graspability.
更多查看译文
关键词
robust robot grasp planning,computer vision,Dex-Net 1.0,parallel-jaw grippers,deep-learning-based approach,cross-entropy method,generative adversarial network,random perturbation algorithm,discrete signed distance functions,3D print adversarial grasp objects,specified robot grasping policy
AI 理解论文
溯源树
样例
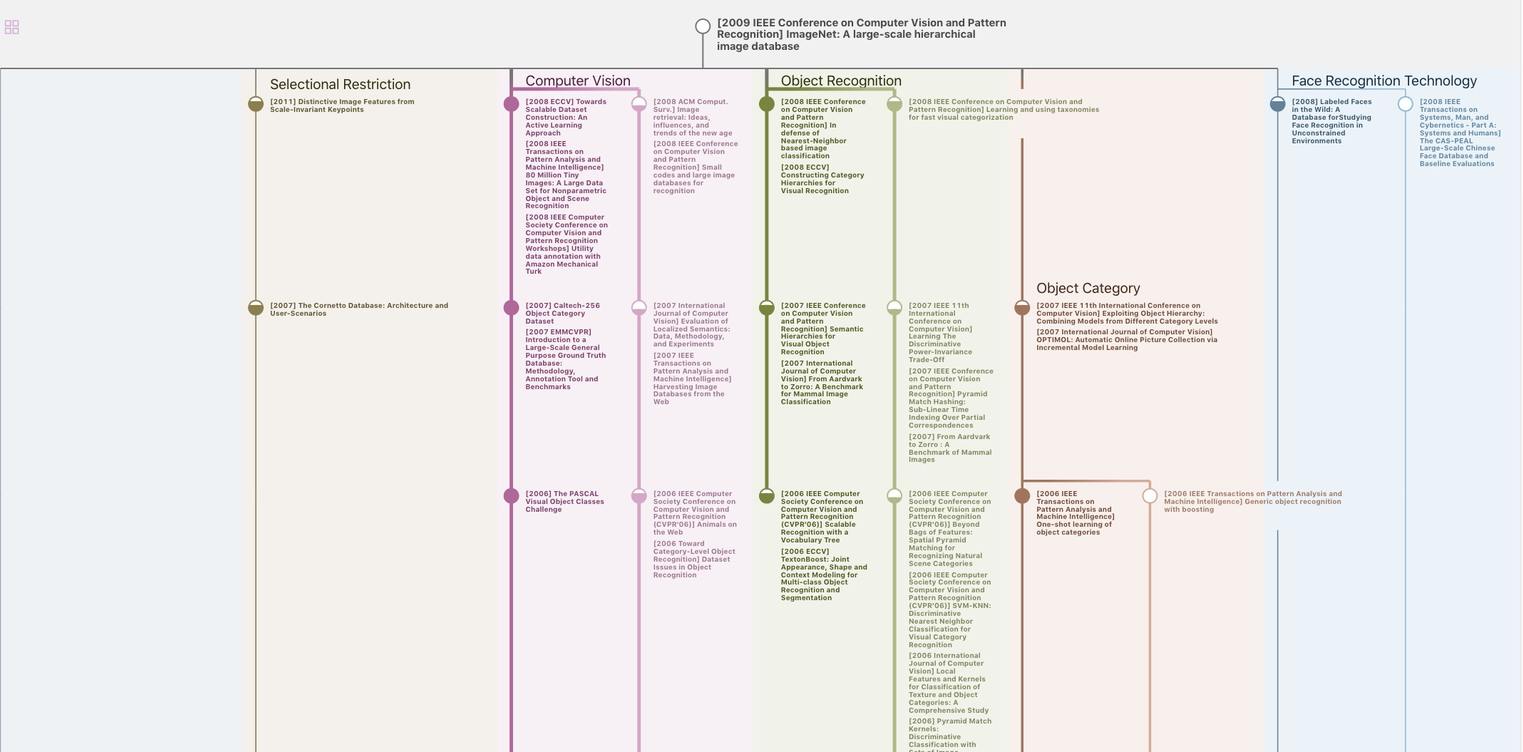
生成溯源树,研究论文发展脉络
Chat Paper
正在生成论文摘要