SRAM on-chip monitoring methodology for high yield and energy efficient memory operation at near threshold voltage
Integration(2020)
摘要
Low power design by near-threshold voltage (NTV) operation is very attractive since it affords to considerably mitigate the sharp increase of power dissipation. However, one key barrier for the use of NTV operation is the significant increase of the SRAM failure. In this work, we propose an on-chip SRAM monitoring methodology that is able to accurately predict the minimum voltage, Vddmin, on each die that does not cause SRAM read and write failures, which are majorities of SRAM failures, under a target confidence level. Precisely, we propose an SRAM monitor, from which we measure a maximum voltage, Vfail, that causes functional failure on that SRAM monitor. Then, we propose a novel methodology of inferring SRAM Vˆddmin on each die from the measured Vfail of SRAM monitor on the same die where Vfail-Vddmin correlation table is built-up in design infra development phase, and Vˆddmin can be directly derived from the measured Vfail referencing the correlation table in silicon production phase. Through experiments, we confirm that our proposed methodology is able to save leakage power by 10.45%, read energy by 4.99%, and write energy by 5.45% in SRAM bitcell array over that by applying a uniform minimum voltage for all dies while meeting the same yield constraint. In addition, the effect of IR drop and process variations of peripheral circuit on Vddmin prediction is taken into account by reflecting them on SRAM bitcell operation. We also solidify our Vddmin prediction methodology by considering the prevention of potential SRAM access failures for high-speed designs as well as the SRAM read and write failures.
更多查看译文
关键词
SRAM failure,On chip monitoring methodology,Near threshold voltage,Low power,Process variation
AI 理解论文
溯源树
样例
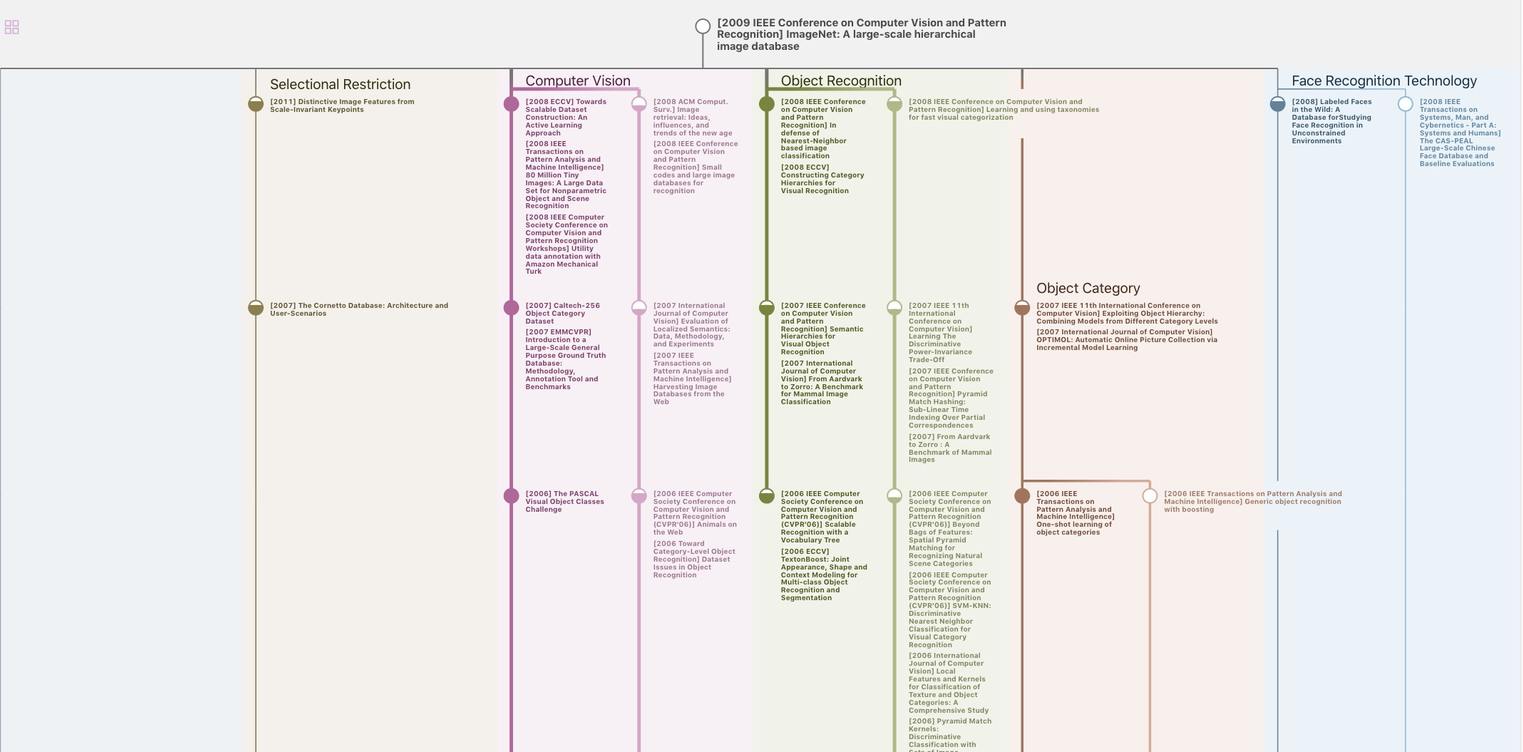
生成溯源树,研究论文发展脉络
Chat Paper
正在生成论文摘要