Image-based Motor Imagery EEG Classification using Convolutional Neural Network
2019 IEEE EMBS International Conference on Biomedical & Health Informatics (BHI)(2019)
摘要
Motor Imagery (MI) based Brain Computer Interface (BCI) has clinical applications such as rehabilitation or communication for patients who have lost motor functions. Accurate classification of motor-imagery based electroencephalography (EEG) is important in developing such BCI applications. We propose an image-based approach to design a convolutional neural network (CNN) to classify EEG signals. In the proposed method, EEG signals were converted into images based on the locations of scalp electrodes, such that spatial correlation between neighbouring EEG channels was taken into consideration. We tested the proposed CNN architecture with both 2D and 3D kernels. EEG data were collected from a locked-in ALS patient over 5 weeks, when the subject was instructed to perform motor-imagery of right hand movement and idle state. Cross-day decoding showed that CNN was able to achieve a 2-class (right vs. idle) classification accuracy of 68.38±7.29% and 65.94±8.52% with 2D and 3D kernels respectively, compared to 55.09±5.74 % for the traditionally used filter bank common spatial pattern (FBCSP) method. We also observed changes in the correlation coefficient of spectral entropy in the EEG data across weeks, and these coefficients reveal frequency bands that are important for decoding. In particular, the CNN architecture with Delta band included performed 6.96% higher than that excluding the Delta band. This study shows that the image-based CNN method improves the classification performance, and the inclusion of Delta band improves classification performance for the current dataset.
更多查看译文
关键词
Electroencephalography,MI-BCI,Convolutional Neural Network,EEG-as-image
AI 理解论文
溯源树
样例
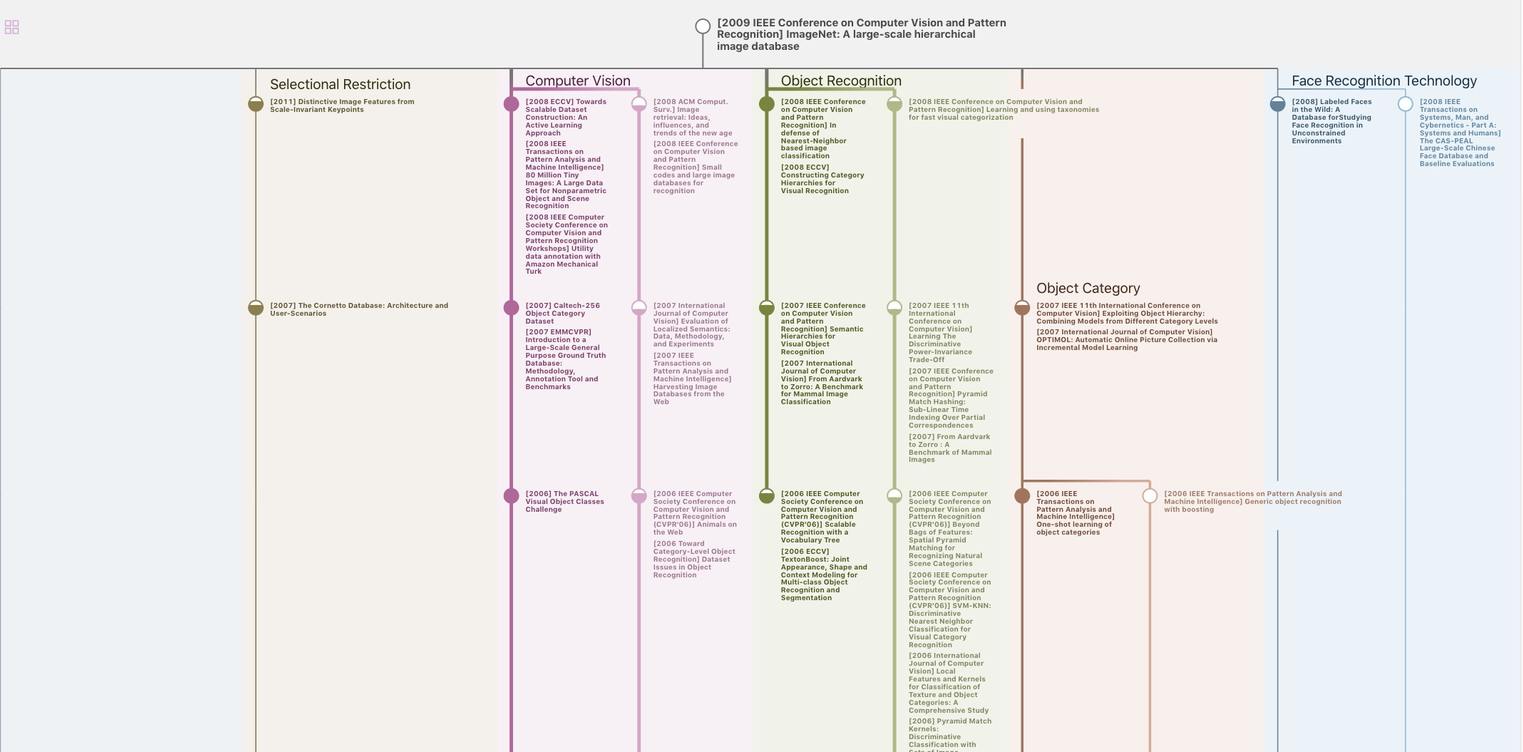
生成溯源树,研究论文发展脉络
Chat Paper
正在生成论文摘要