Sleep Stage Classification Using Bidirectional LSTM in Wearable Multi-sensor Systems
IEEE INFOCOM 2019 - IEEE Conference on Computer Communications Workshops (INFOCOM WKSHPS)(2019)
摘要
Understanding the sleep quality and architecture is essential to human being's health, which is usually represented using multiple sleep stages. A standard sleep stage determination requires Electroencephalography (EEG) signals during the expensive and labor-intensive Polysomnography (PSG) test. To overcome this inconvenience, cardiorespiratory signals are proposed for the same purpose because of the easy and comfortable acquisition by simplified devices. In this paper, we leverage our low-cost wearable multi-sensor system to acquire the cardiorespiratory signals from subjects. Three novel features are designed during the feature extraction. We then apply a Bidirectional Recurrent Neural Network architecture with Long Short-term Memory (BLSTM) to predict the four-class sleep stages. Our prediction accuracy is 80.25% on a large public dataset (417 subjects), and 80.75% on our 32 enrolled subjects, respectively. Our results outperform the previous works which either used small data sets and had the potential over-fitting issues, or used the conventional machine learning methods on large data sets.
更多查看译文
关键词
—Sleep stage,Wearable sensors,Healthcare,Deep learning
AI 理解论文
溯源树
样例
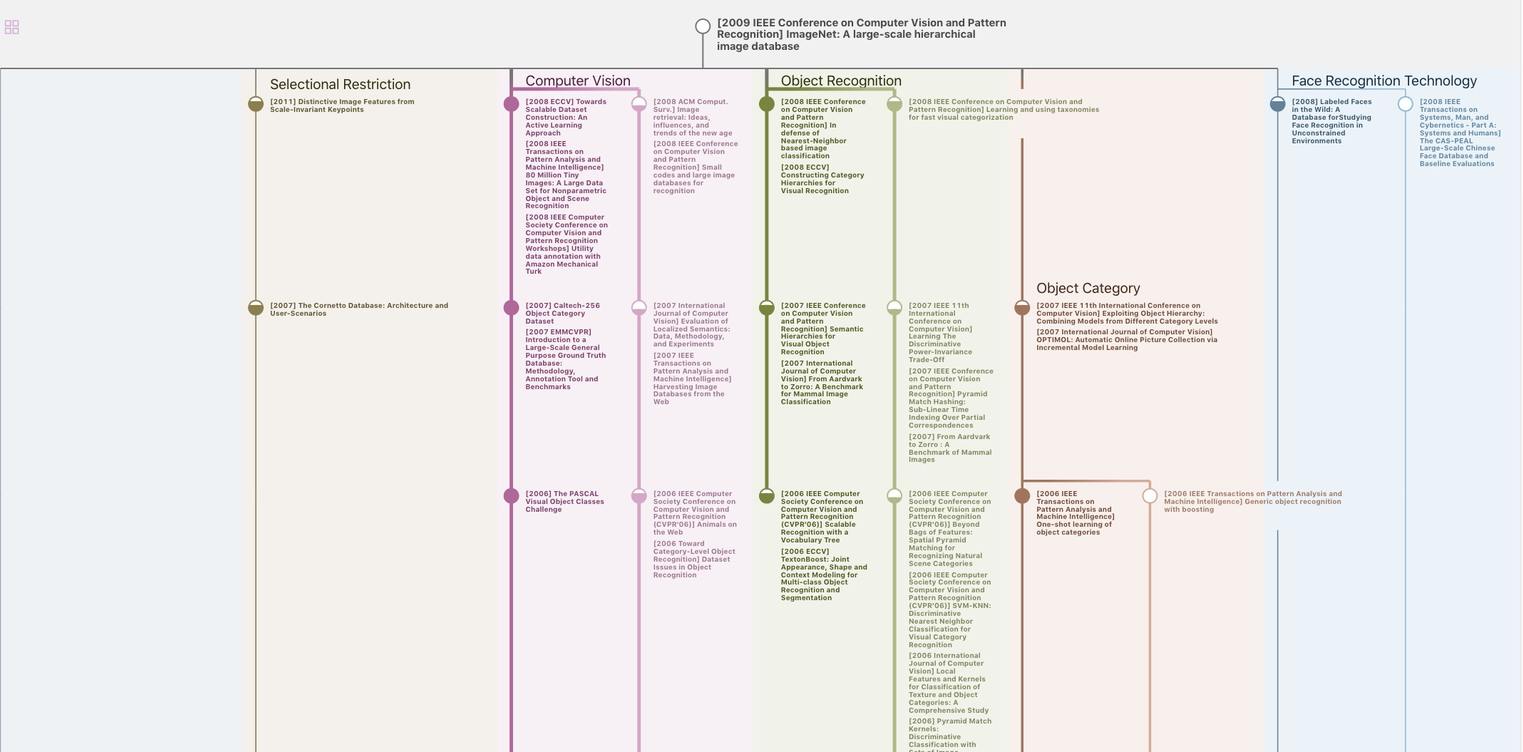
生成溯源树,研究论文发展脉络
Chat Paper
正在生成论文摘要