Attention Convolutional Binary Neural Tree for Fine-Grained Visual Categorization
CVPR(2020)
摘要
Fine-grained visual categorization (FGVC) is an important but challenging task due to high intra-class variances and low inter-class variances caused by deformation, occlusion, illumination, etc. An attention convolutional binary neural tree architecture is presented to address those problems for weakly supervised FGVC. Specifically, we incorporate convolutional operations along edges of the tree structure, and use the routing functions in each node to determine the root-to-leaf computational paths within the tree. The final decision is computed as the summation of the predictions from leaf nodes. The deep convolutional operations learn to capture the representations of objects, and the tree structure characterizes the coarse-to-fine hierarchical feature learning process. In addition, we use the attention transformer module to enforce the network to capture discriminative features. The negative log-likelihood loss is used to train the entire network in an end-to-end fashion by SGD with back-propagation. Several experiments on the CUB-200-2011, Stanford Cars and Aircraft datasets demonstrate that the proposed method performs favorably against the state-of-the-arts.
更多查看译文
关键词
deep convolutional operations,tree structure,coarse-to-fine hierarchical feature learning process,attention transformer module,fine-grained visual categorization,high intra-class variances,low inter-class variances,attention convolutional binary neural tree architecture,weakly supervised FGVC,root-to-leaf computational paths,leaf nodes,negative log-likelihood loss
AI 理解论文
溯源树
样例
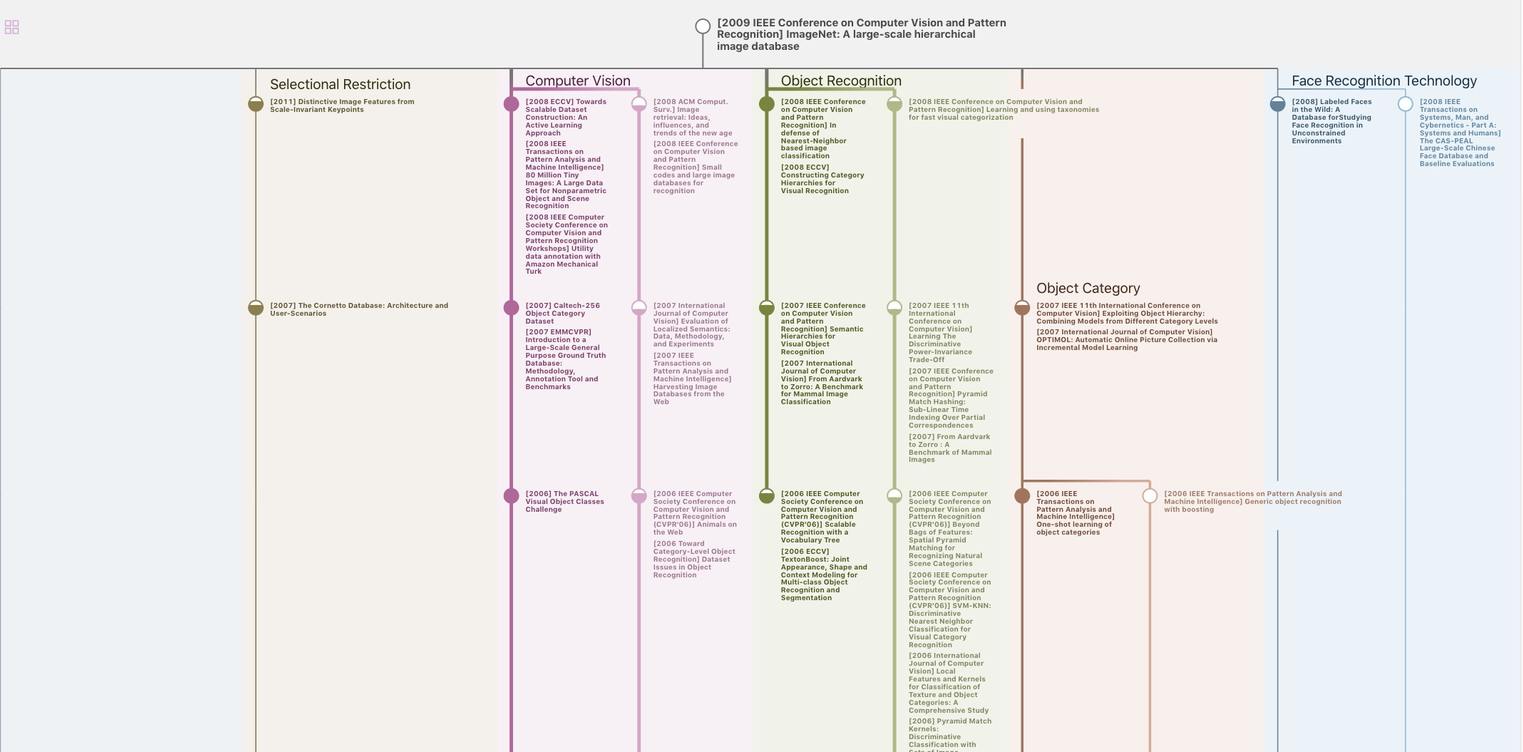
生成溯源树,研究论文发展脉络
Chat Paper
正在生成论文摘要