Adversarial Distillation for Learning with Privileged Provisions
IEEE Transactions on Pattern Analysis and Machine Intelligence(2021)
摘要
Knowledge distillation aims to train a student (model) for accurate inference in a resource-constrained environment. Traditionally, the student is trained by a high-capacity teacher (model) whose training is resource-intensive. The student trained this way is suboptimal because it is difficult to learn the real data distribution from the teacher. To address this issue, we propose to train the student against a discriminator in a minimax game. Such a minimax game has an issue that it can take an excessively long time for the training to converge. To address this issue, we propose adversarial distillation consisting of a student, a teacher, and a discriminator. The discriminator is now a multi-class classifier that distinguishes among the real data, the student, and the teacher. The student and the teacher aim to fool the discriminator via adversarial losses, while they learn from each other via distillation losses. By optimizing the adversarial and the distillation losses simultaneously, the student and the teacher can learn the real data distribution. To accelerate the training, we propose to obtain low-variance gradient updates from the discriminator using a Gumbel-Softmax trick. We conduct extensive experiments to demonstrate the superiority of the proposed adversarial distillation under both accuracy and training speed.
更多查看译文
关键词
Adversarial distillation,generative adversarial network,knowledge distillation,privileged information
AI 理解论文
溯源树
样例
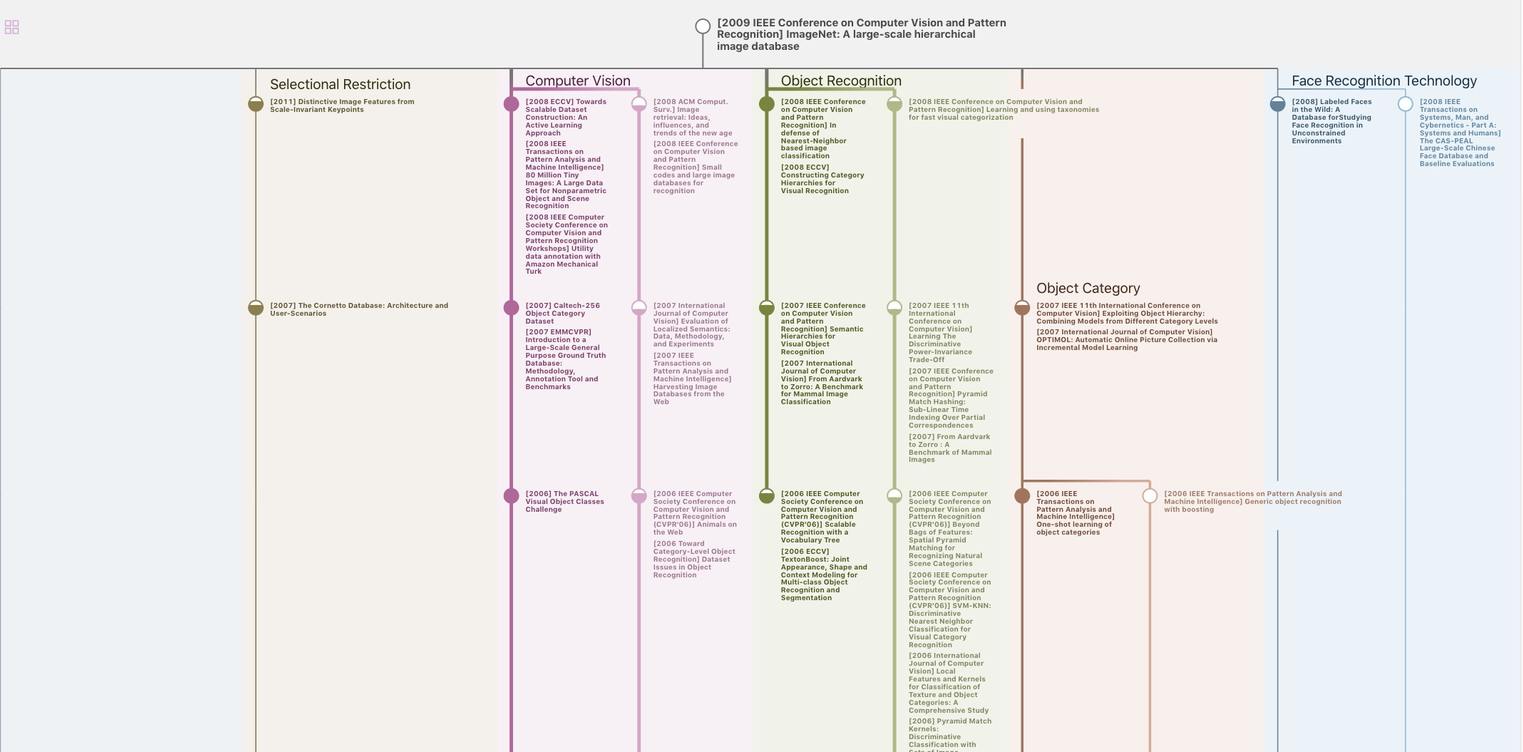
生成溯源树,研究论文发展脉络
Chat Paper
正在生成论文摘要