Margin-Based Generalization Lower Bounds for Boosted Classifiers
ADVANCES IN NEURAL INFORMATION PROCESSING SYSTEMS 32 (NIPS 2019)(2019)
摘要
Boosting is one of the most successful ideas in machine learning. The most well-accepted explanations for the low generalization error of boosting algorithms such as AdaBoost stem from margin theory. The study of margins in the context of boosting algorithms was initiated by Schapire, Freund, Bartlett and Lee (1998) and has inspired numerous boosting algorithms and generalization bounds. To date, the strongest known generalization (upper bound) is the kth margin bound of Gao and Zhou (2013). Despite the numerous generalization upper bounds that have been proved over the last two decades, nothing is known about the tightness of these bounds. In this paper, we give the first margin-based lower bounds on the generalization error of boosted classifiers. Our lower bounds nearly match the kth margin bound and thus almost settle the generalization performance of boosted classifiers in terms of margins.
更多查看译文
关键词
boosting algorithms
AI 理解论文
溯源树
样例
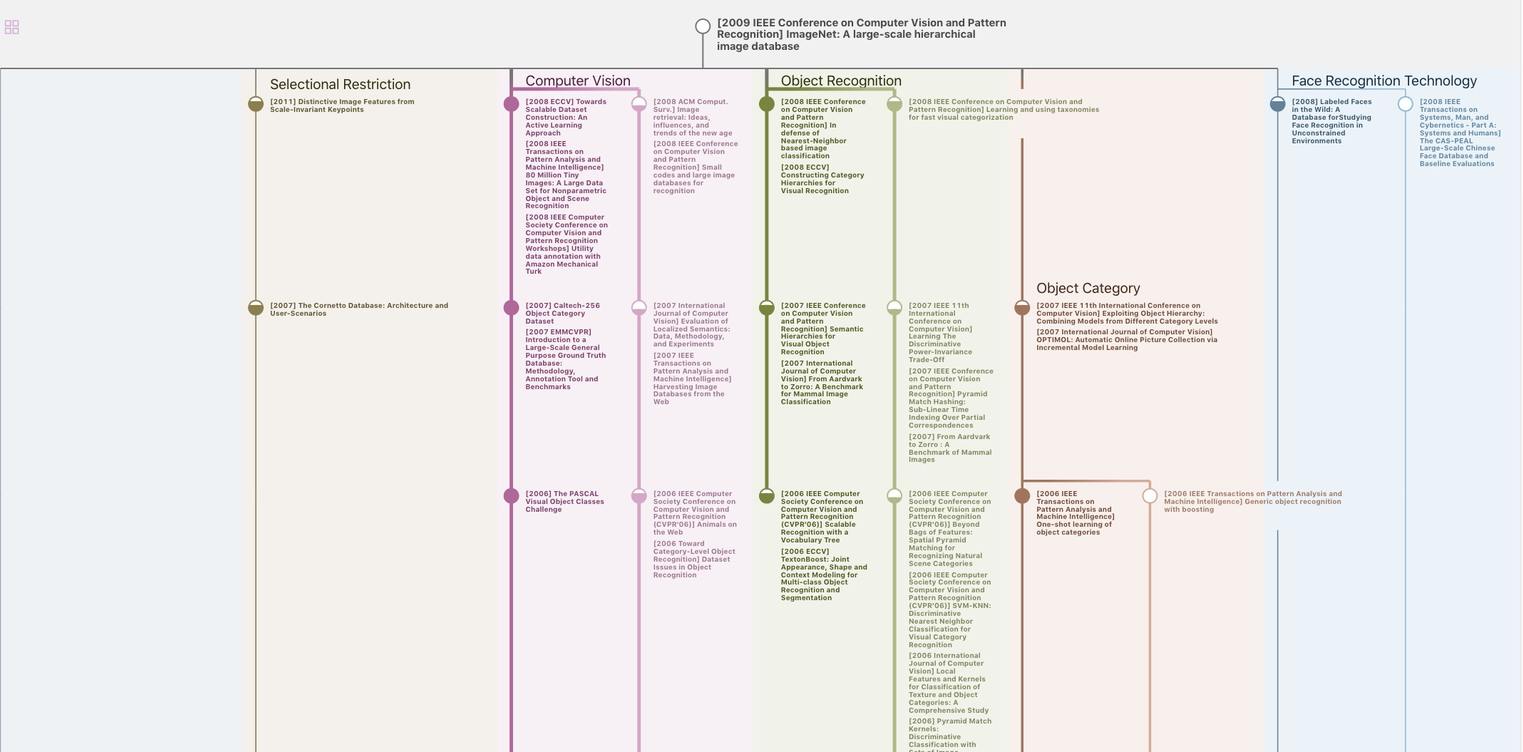
生成溯源树,研究论文发展脉络
Chat Paper
正在生成论文摘要