Debiased/Double Machine Learning For Instrumental Variable Quantile Regressions
arxiv(2021)
摘要
In this study, we investigate the estimation and inference on a low-dimensional causal parameter in the presence of high-dimensional controls in an instrumental variable quantile regression. Our proposed econometric procedure builds on the Neyman-type orthogonal moment conditions of a previous study (Chernozhukov et al. 2018) and is thus relatively insensitive to the estimation of the nuisance parameters. The Monte Carlo experiments show that the estimator copes well with high-dimensional controls. We also apply the procedure to empirically reinvestigate the quantile treatment effect of 401(k) participation on accumulated wealth.
更多查看译文
关键词
quantile treatment effect, instrumental variable, quantile regression, double machine learning, lasso
AI 理解论文
溯源树
样例
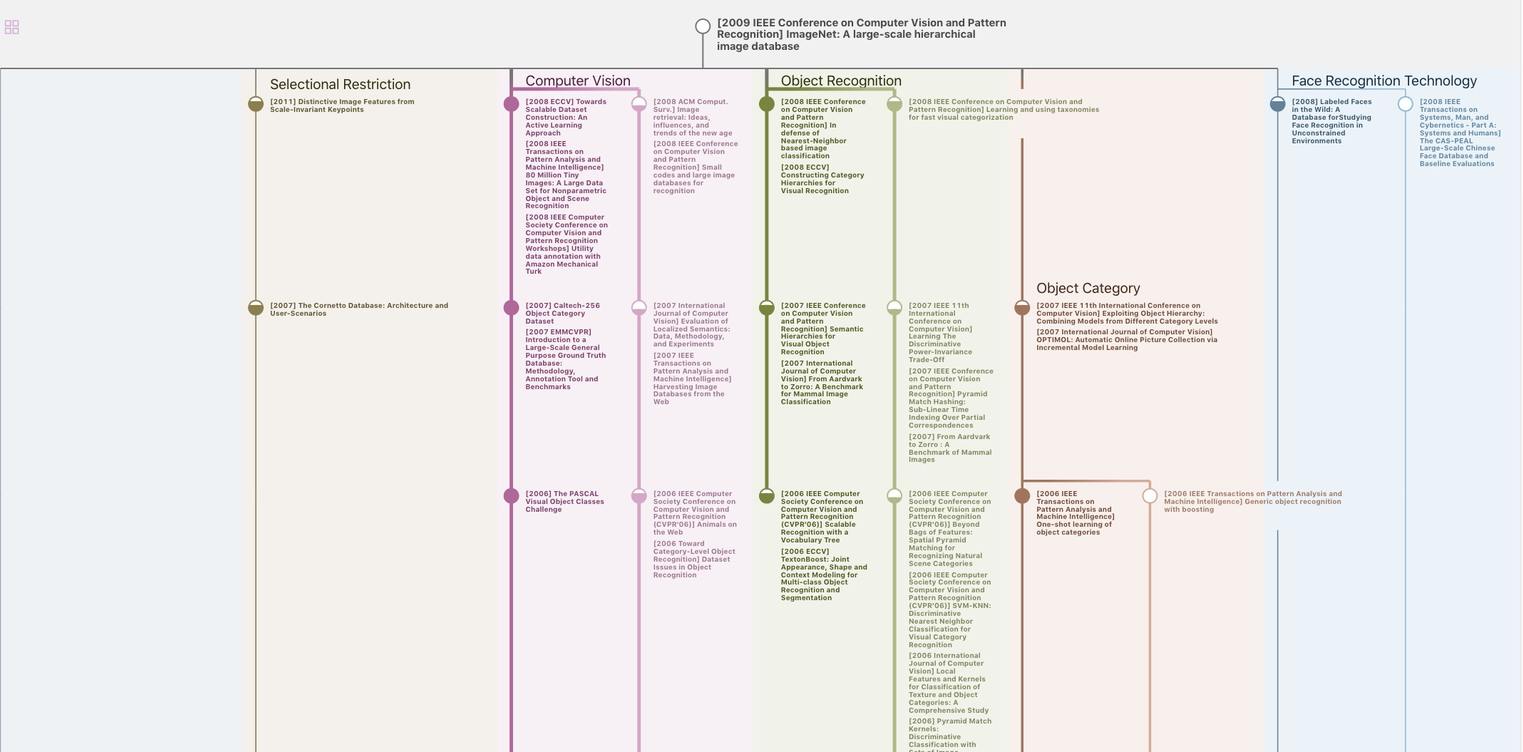
生成溯源树,研究论文发展脉络
Chat Paper
正在生成论文摘要