A Constructive Prediction of the Generalization Error Across Scales
ICLR(2020)
摘要
The dependency of the generalization error of neural networks on model and dataset size is of critical importance both in practice and for understanding the theory of neural networks. Nevertheless, the functional form of this dependency remains elusive. In this work, we present a functional form which approximates well the generalization error in practice. Capitalizing on the successful concept of model scaling (e.g., width, depth), we are able to simultaneously construct such a form and specify the exact models which can attain it across model/data scales. Our construction follows insights obtained from observations conducted over a range of model/data scales, in various model types and datasets, in vision and language tasks. We show that the form both fits the observations well across scales, and provides accurate predictions from small- to large-scale models and data.
更多查看译文
关键词
neural networks, deep learning, generalization error, scaling, scalability, vision, language
AI 理解论文
溯源树
样例
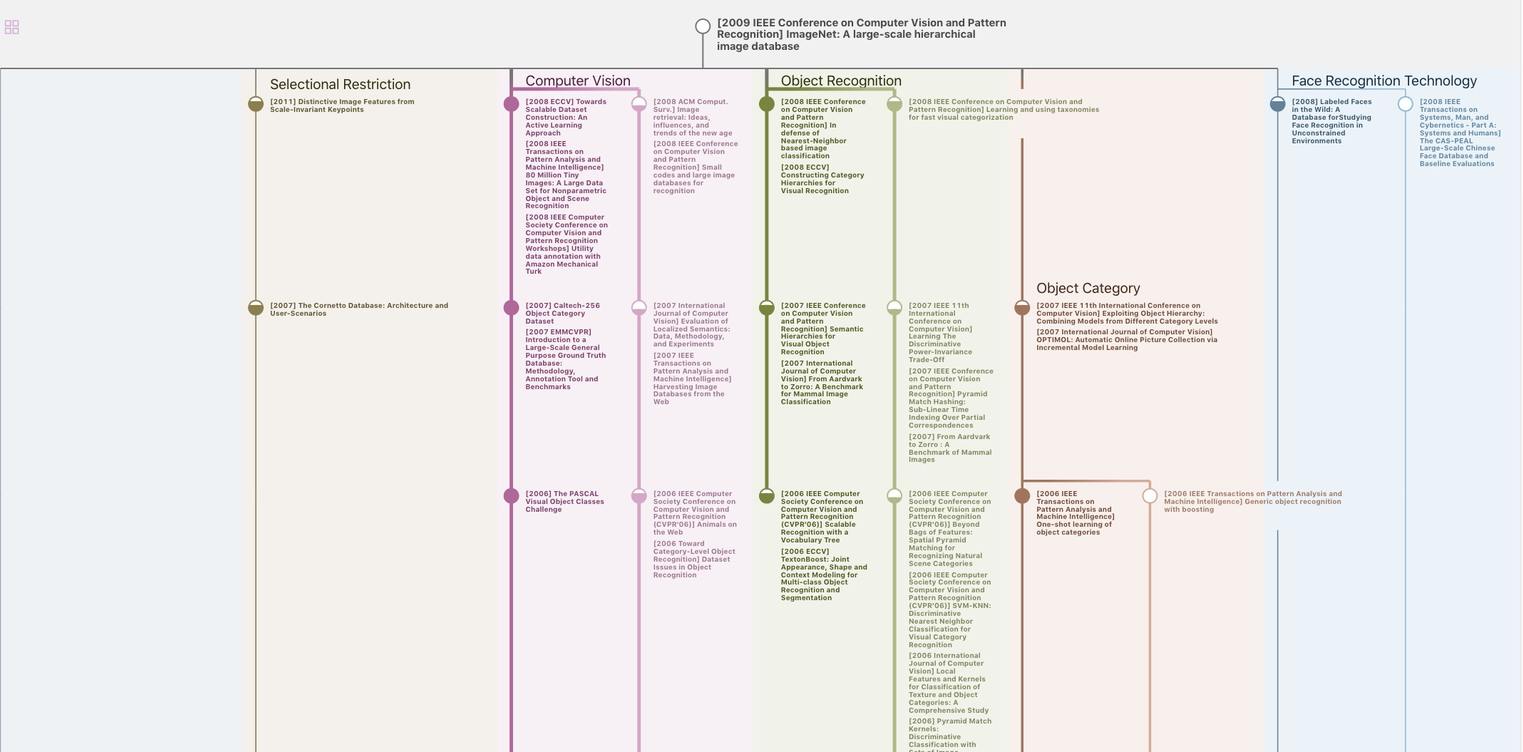
生成溯源树,研究论文发展脉络
Chat Paper
正在生成论文摘要