Learning an Action-Conditional Model for Haptic Texture Generation
ICRA(2020)
摘要
Rich haptic sensory feedback in response to user interactions is desirable for an effective, immersive virtual reality or teleoperation system. However, this feedback depends on material properties and user interactions in a complex, non-linear manner. Therefore, it is challenging to model the mapping from material and user interactions to haptic feedback in a way that generalizes over many variations of the user's input. Current methodologies are typically conditioned on user interactions, but require a separate model for each material. In this paper, we present a learned action-conditional model that uses data from a vision-based tactile sensor (GelSight) and user's action as input. This model predicts an induced acceleration that could be used to provide haptic vibration feedback to a user. We trained our proposed model on a publicly available dataset (Penn Haptic Texture Toolkit) that we augmented with GelSight measurements of the different materials. We show that a unified model over all materials outperforms previous methods and generalizes to new actions and new instances of the material categories in the dataset.
更多查看译文
关键词
Haptic Texture generation,haptic sensory feedback,user interactions,immersive virtual reality,material properties,haptic vibration feedback,Penn Haptic Texture Toolkit,action-conditional model learning,GelSight measurements,teleoperation system,autonomous robot,GelSight image texture
AI 理解论文
溯源树
样例
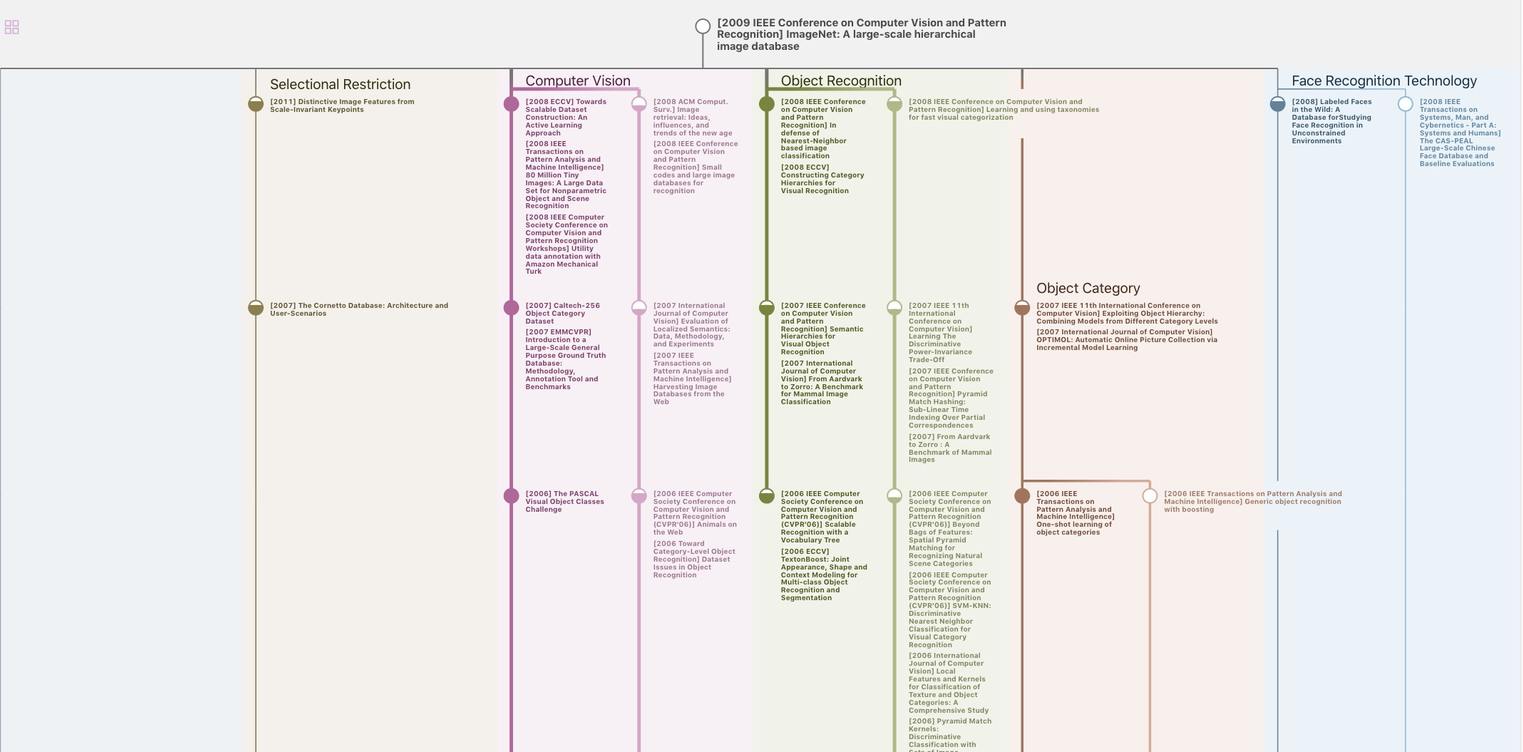
生成溯源树,研究论文发展脉络
Chat Paper
正在生成论文摘要