Wasserstein-2 Generative Networks.
arXiv (Cornell University)(2019)
摘要
We propose a novel end-to-end non-minimax algorithm for training optimaltransport mappings for the quadratic cost (Wasserstein-2 distance). Thealgorithm uses input convex neural networks and a cycle-consistencyregularization to approximate Wasserstein-2 distance. In contrast to popularentropic and quadratic regularizers, cycle-consistency does not introduce biasand scales well to high dimensions. From the theoretical side, we estimate theproperties of the generative mapping fitted by our algorithm. From thepractical side, we evaluate our algorithm on a wide range of tasks:image-to-image color transfer, latent space optimal transport, image-to-imagestyle transfer, and domain adaptation.
更多查看译文
关键词
Volume Rendering,Representation Learning,Rendering
AI 理解论文
溯源树
样例
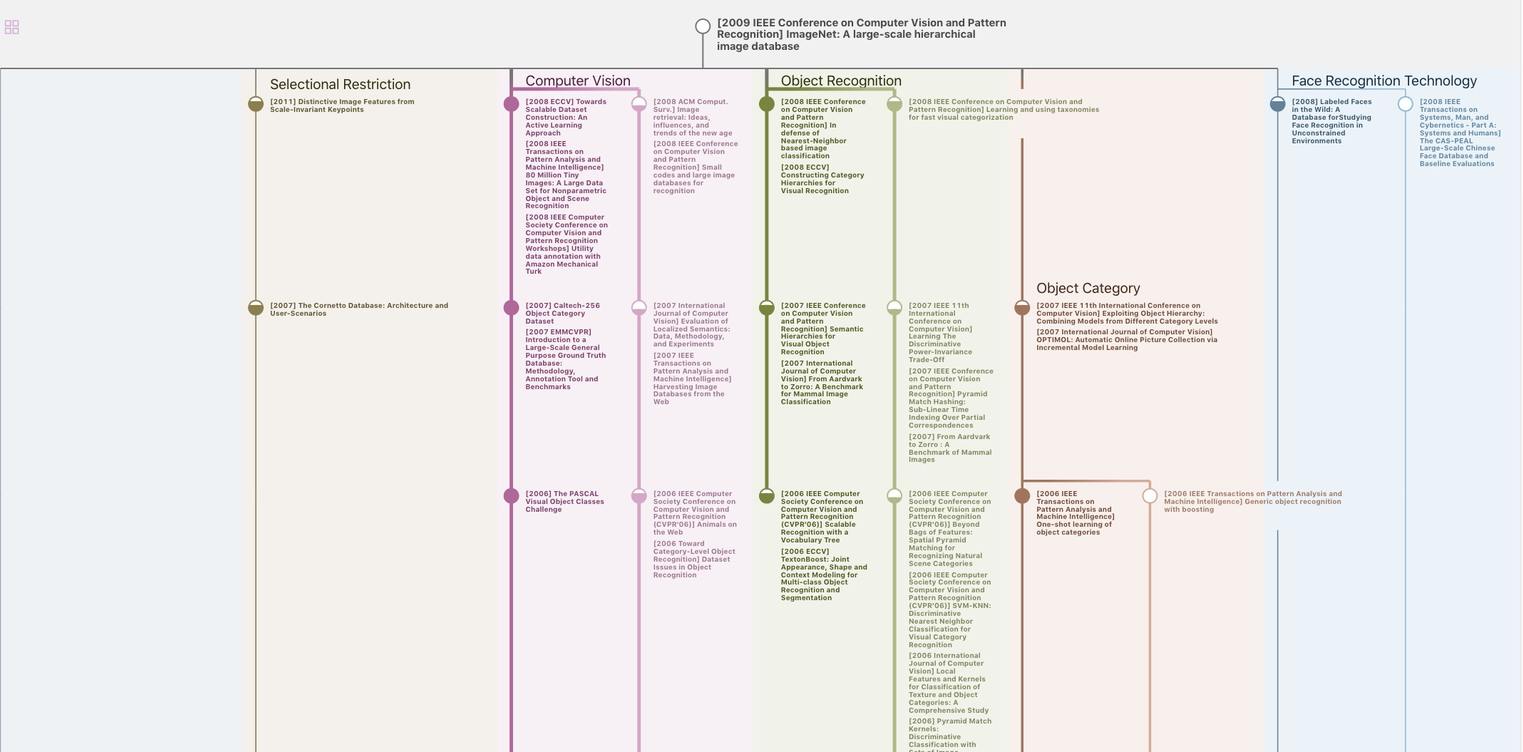
生成溯源树,研究论文发展脉络
Chat Paper
正在生成论文摘要