Model-Free Primal-Dual Methods For Network Optimization With Application To Real-Time Optimal Power Flow
2020 AMERICAN CONTROL CONFERENCE (ACC)(2020)
摘要
This paper examines the problem of real-time optimization of networked systems and develops online algorithms that steer the system towards the optimal trajectory without explicit knowledge of the system model. The problem is modeled as a dynamic optimization problem with timevarying performance objectives and engineering constraints. The design of the algorithms leverages the online zero-order primal-dual projected-gradient method. In particular, the primal step that involves the gradient of the objective function (and hence requires a networked systems model) is replaced by its zero-order approximation with two function evaluations using a deterministic perturbation signal. The evaluations are performed using the measurements of the system output, hence giving rise to a feedback interconnection, with the optimization algorithm serving as a feedback controller. The paper provides some insights on the stability and tracking properties of this interconnection. Finally, the paper applies this methodology to a real-time optimal power flow problem in power systems, and shows its efficacy on the IEEE 37-node distribution test feeder for reference power tracking and voltage regulation.
更多查看译文
关键词
network optimization,power flow,model-free,primal-dual,real-time
AI 理解论文
溯源树
样例
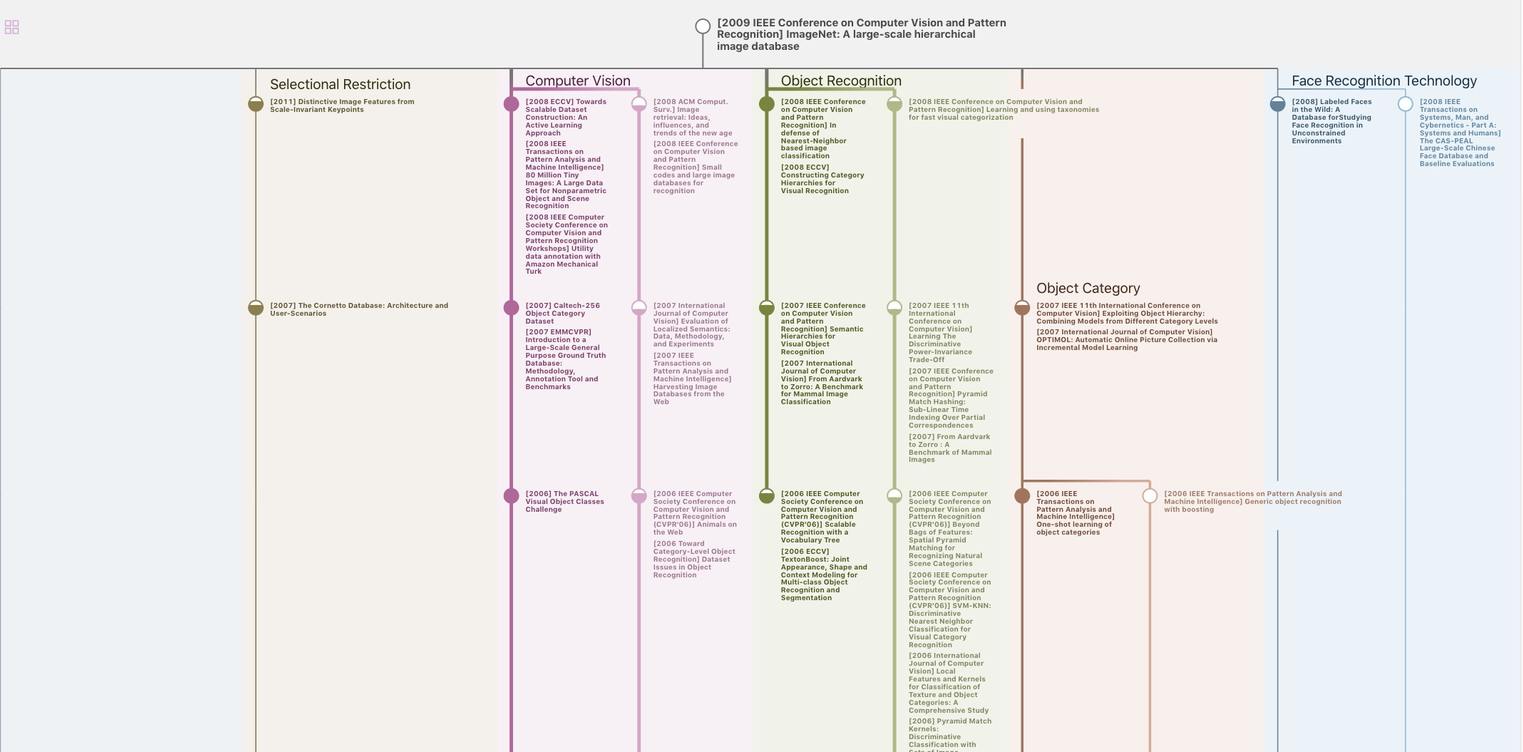
生成溯源树,研究论文发展脉络
Chat Paper
正在生成论文摘要