Procedural Generation using Spatial GANs for Region-Specific Learning of Elevation Data.
CoG(2019)
摘要
Heightmap generation is currently a tedious topic with the majority of generation using Perlin noise which forms a reliable, but sometimes repetitive output. In this paper, a method of generating height maps from real-world digital elevation data taken from specific regions of the planet is proposed. Raw elevation data sourced from NASA’s SRTM (30m) data set is transformed into a height map format, this data is then passed into a two type unsupervised model. The method uses a type of generative adversarial network to learn the spatially-invariant features within the input regions. Producing a network model that can output an extensive amount of varying, but visually and structurally similar height maps to that of the input regions. The visual validity of outputs from the network was tested using data from 262 human participants, with over 90.15% of generated samples being correctly assigned to the original input data with a significance of P u003c0.001.
更多查看译文
关键词
Deep Learning,Generative Adversarial Network,Height Maps,PCG
AI 理解论文
溯源树
样例
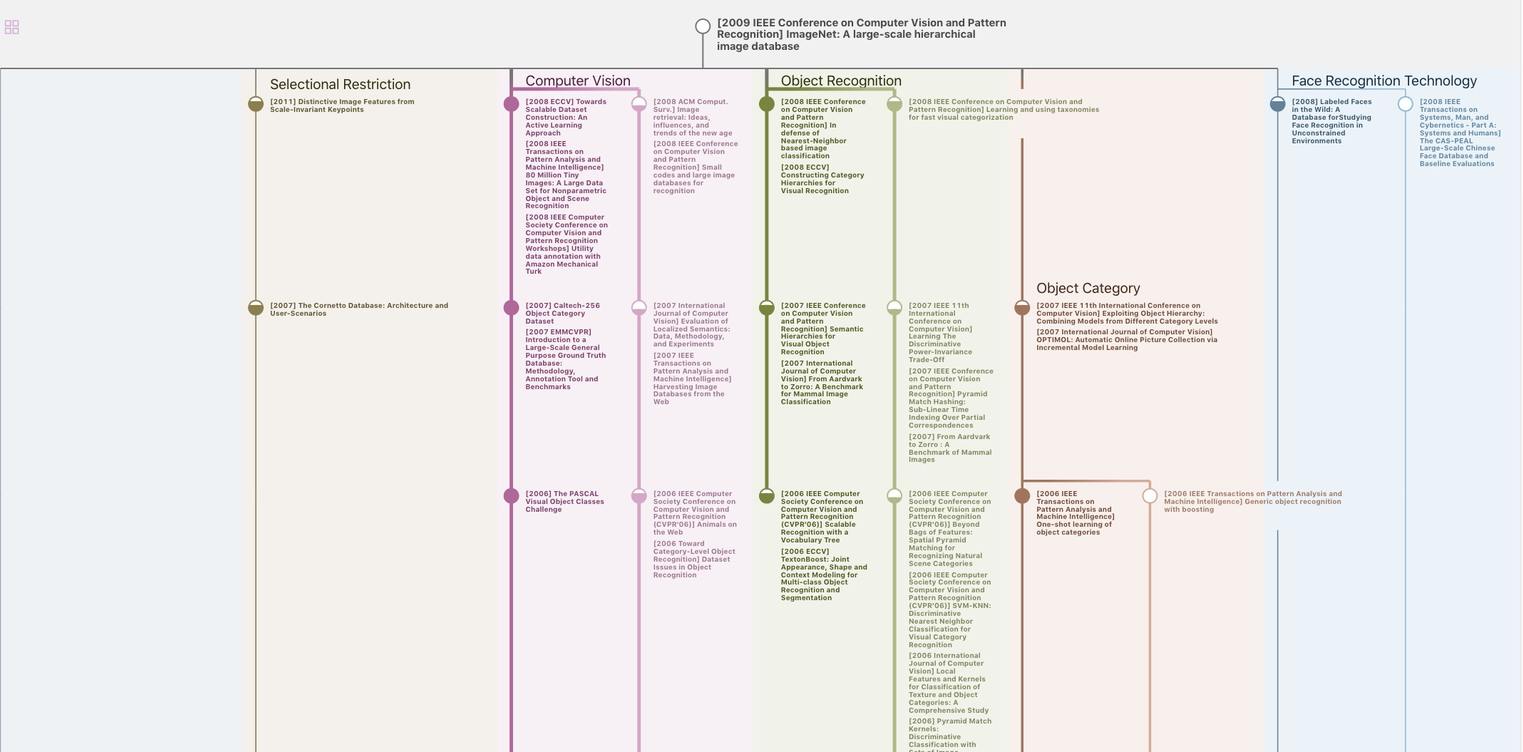
生成溯源树,研究论文发展脉络
Chat Paper
正在生成论文摘要