Hyperspectral Band Selection Using Weighted Kernel Regularization
IEEE Journal of Selected Topics in Applied Earth Observations and Remote Sensing(2019)
摘要
A band selection method named weighted kernel regularization (WKR) is proposed for hyperspectral imagery (HSI) classification. The WKR aims to select dissimilar and class-separable bands to better model the relationship between labeled samples. First, the WKR considers nonlinear structure of hyperspectral data and models nonlinear relations between HSI samples and their class labels using a weighted kernel ridge regression (WKRR) program with respect to sample coefficients. Second, it combines the L
1
penalty term of weights on all bands with the above WKRR program into the unified framework of WKR. The L
1
penalty term considers divergent contributions from different bands in describing nonlinear relations and guarantees the sparsity of band weights. Third, the WKR algorithm implements the KerNel Iterative-based Feature Extraction (KNIFE) algorithm to estimate the proper band weights. The KNIFE linearizes the nonlinear kernels to avoid high computational cost, and iteratively minimizes two convex subproblems with respect to the sample coefficients and band weights. Finally, the first
k
bands with larger weights and larger dissimilarity with other bands are automatically chosen to form the band subset. Experimental results show that the WKR outperforms the state-of-the-art methods in classification accuracies with a lower computational cost.
更多查看译文
关键词
Kernel,Clustering algorithms,Hyperspectral imaging,Linear regression,Earth
AI 理解论文
溯源树
样例
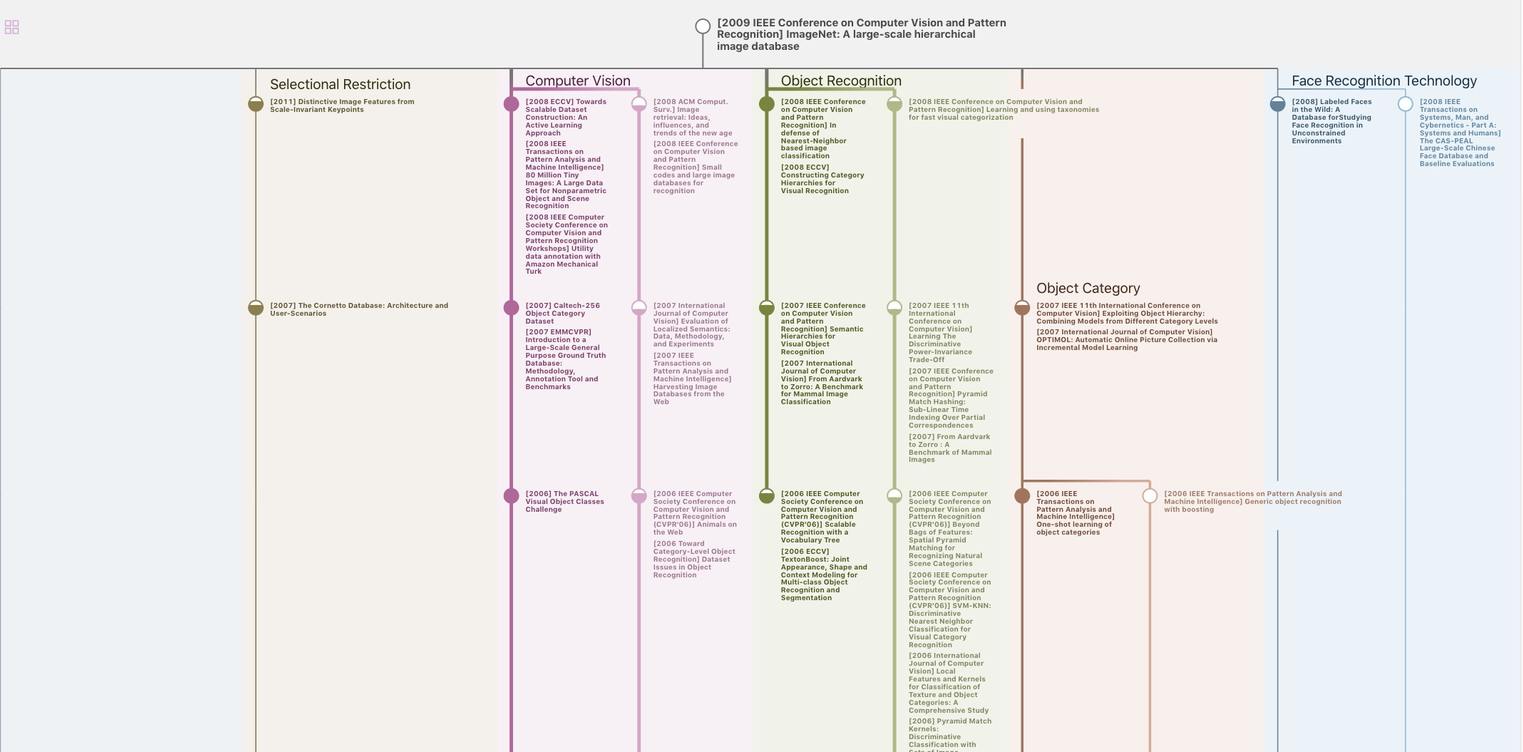
生成溯源树,研究论文发展脉络
Chat Paper
正在生成论文摘要