Pay Attention: Leveraging Sequence Models to Predict the Useful Life of Batteries
CoRR(2019)
摘要
We use data on 124 batteries released by Stanford University to first try to solve the binary classification problem of determining if a battery is "good" or "bad" given only the first 5 cycles of data (i.e., will it last longer than a certain threshold of cycles), as well as the prediction problem of determining the exact number of cycles a battery will last given the first 100 cycles of data. We approach the problem from a purely data-driven standpoint, hoping to use deep learning to learn the patterns in the sequences of data that the Stanford team engineered by hand. For both problems, we used a similar deep network design, that included an optional 1-D convolution, LSTMs, an optional Attention layer, followed by fully connected layers to produce our output. For the classification task, we were able to achieve very competitive results, with validation accuracies above 90%, and a test accuracy of 95%, compared to the 97.5% test accuracy of the current leading model. For the prediction task, we were also able to achieve competitive results, with a test MAPE error of 12.5% as compared with a 9.1% MAPE error achieved by the current leading model (Severson et al. 2019).
更多查看译文
AI 理解论文
溯源树
样例
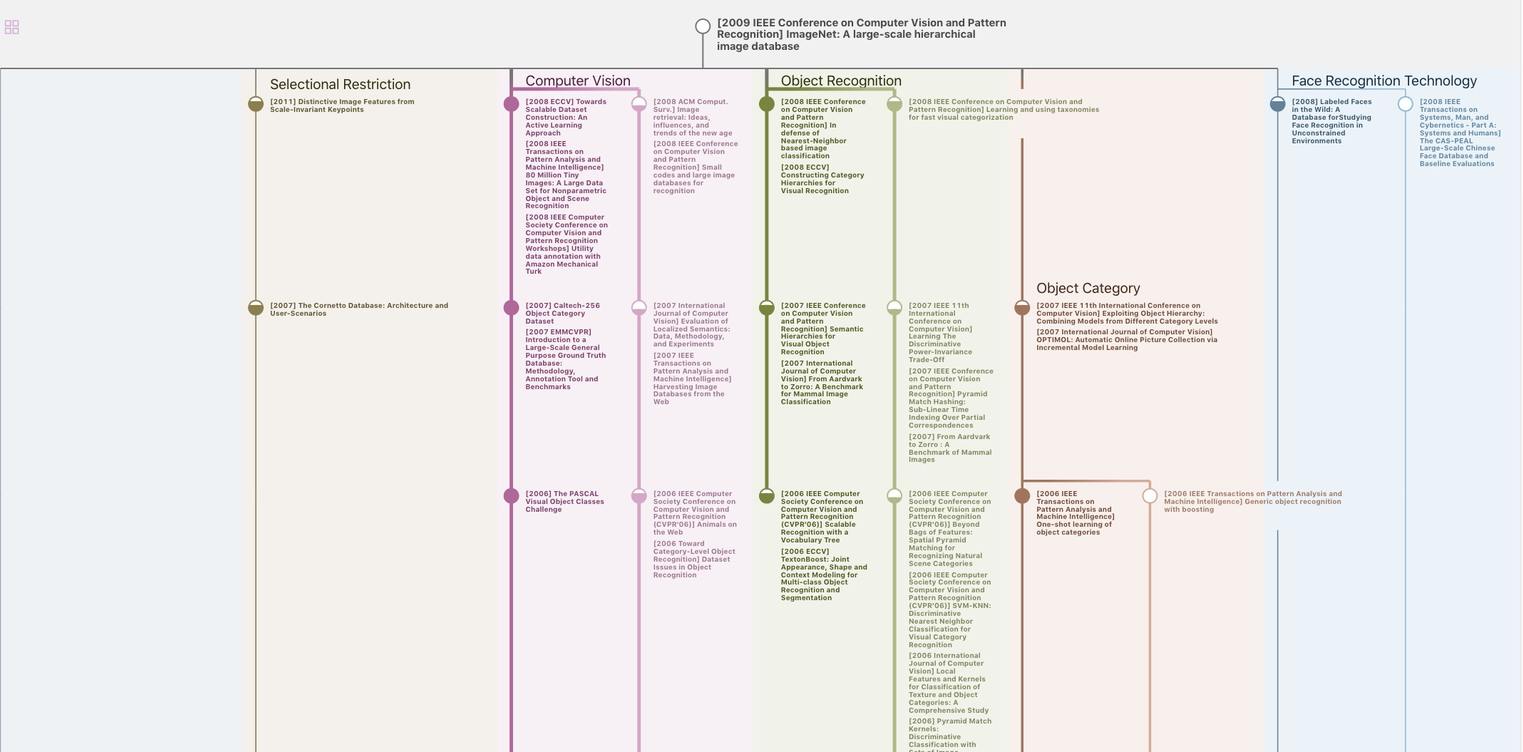
生成溯源树,研究论文发展脉络
Chat Paper
正在生成论文摘要