Analyzing Minimal Complexity Machines
2019 INTERNATIONAL JOINT CONFERENCE ON NEURAL NETWORKS (IJCNN)(2019)
摘要
The minimal complexity machine (MCM) minimizes the maximum distance between training data and the separating hyperplane and is shown to generalize better than the conventional support vector machine. In this paper, we analyze the MCM and clarify the conditions that the solution of MCM is nonunique and unbounded. To resolve the unboundedness, we propose the minimal complexity linear programming support vector machine (MLP SVM), in which the minimization of the maximum distance between training data and the separating hyperplane is added to the linear programming support vector machine (LP SVM). By computer experiments we show that the solution of the MCM is unbounded under some conditions and that the MLP SVM generalizes better than the LP SVM for most of the two-class and multiclass problems.
更多查看译文
关键词
maximum distance,MCM,MLP SVM,minimal complexity linear programming support vector machine,hyperplane separation
AI 理解论文
溯源树
样例
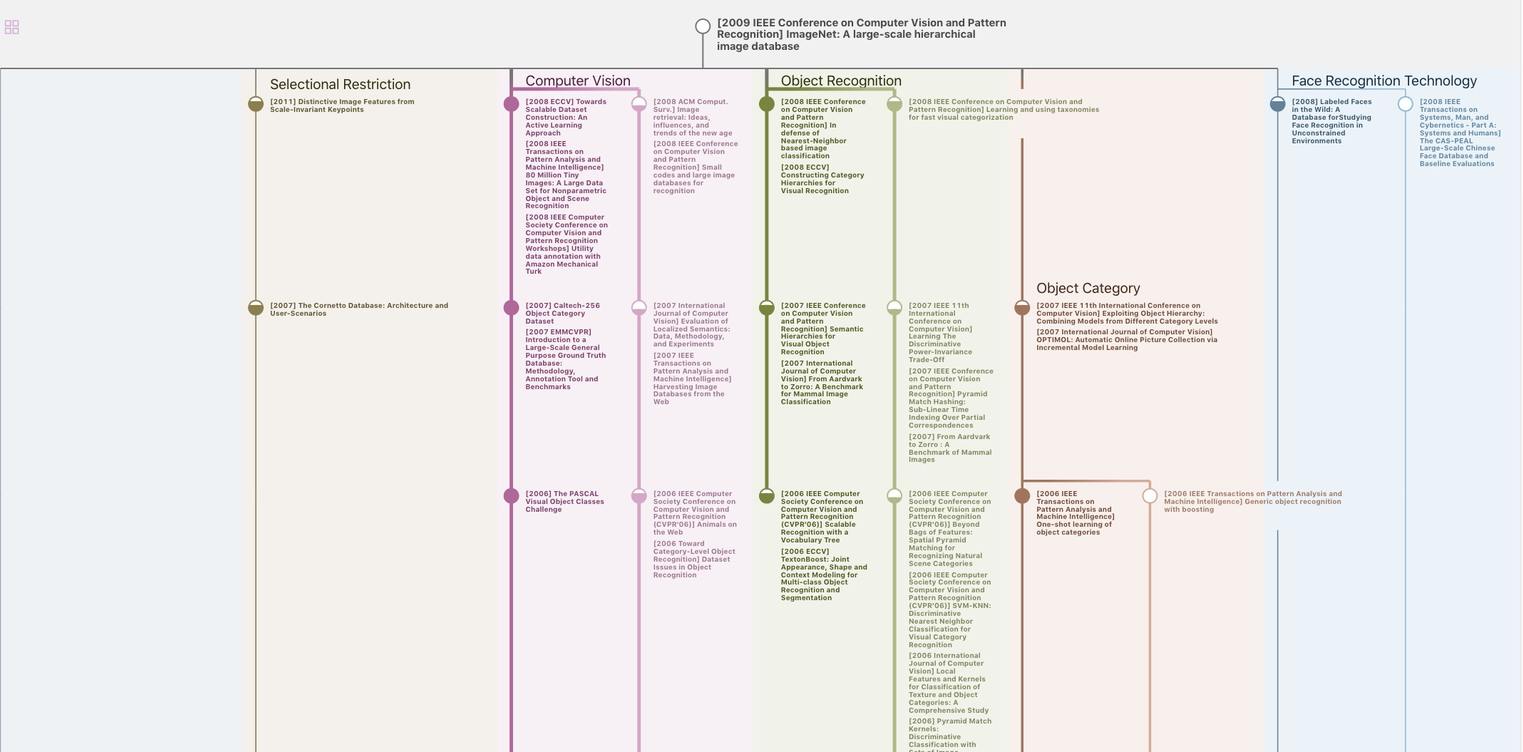
生成溯源树,研究论文发展脉络
Chat Paper
正在生成论文摘要