Efficient and Robust Convolutional Neural Networks via Channel Prioritization and Path Ensemble
2019 INTERNATIONAL JOINT CONFERENCE ON NEURAL NETWORKS (IJCNN)(2019)
摘要
With the growing recognition of both efficiency and security issues in machine learning models, we propose a novel convolutional neural networks (CNNs) training algorithm, called channel prioritization and path ensemble (CPPE), to not only allow dynamically trade-offs between different resource and performance requirements but also enable secure inference without any extra computational cost or memory overhead. Our approach not only prioritizes channels to prune the network in a structured way and ensemble multiple inference paths over different utilization conditions. We demonstrated the effectiveness of channel prioritization by the experiment of the VGG-16 network on various benchmark datasets. The experimental results show that, on the CIFAR-10 dataset, a 10× parameters reduction and a 4× FLOPs reduction can be achieved, with only a 0.2% accuracy drop. Furthermore, we allow CNNs to dynamically trade-offs between resource demand and accuracy with only 4% degradation in accuracy in exchange for 16× FLOPs reduction. By ensembling multiple inference paths, our model can improve robustness against various adversarial attacks without any additional computational cost and memory overhead. Finally, our method is simple and easily applied to any convolutioanl neural networks.
更多查看译文
关键词
efficient inference, model compression, network pruning, security, model ensemble
AI 理解论文
溯源树
样例
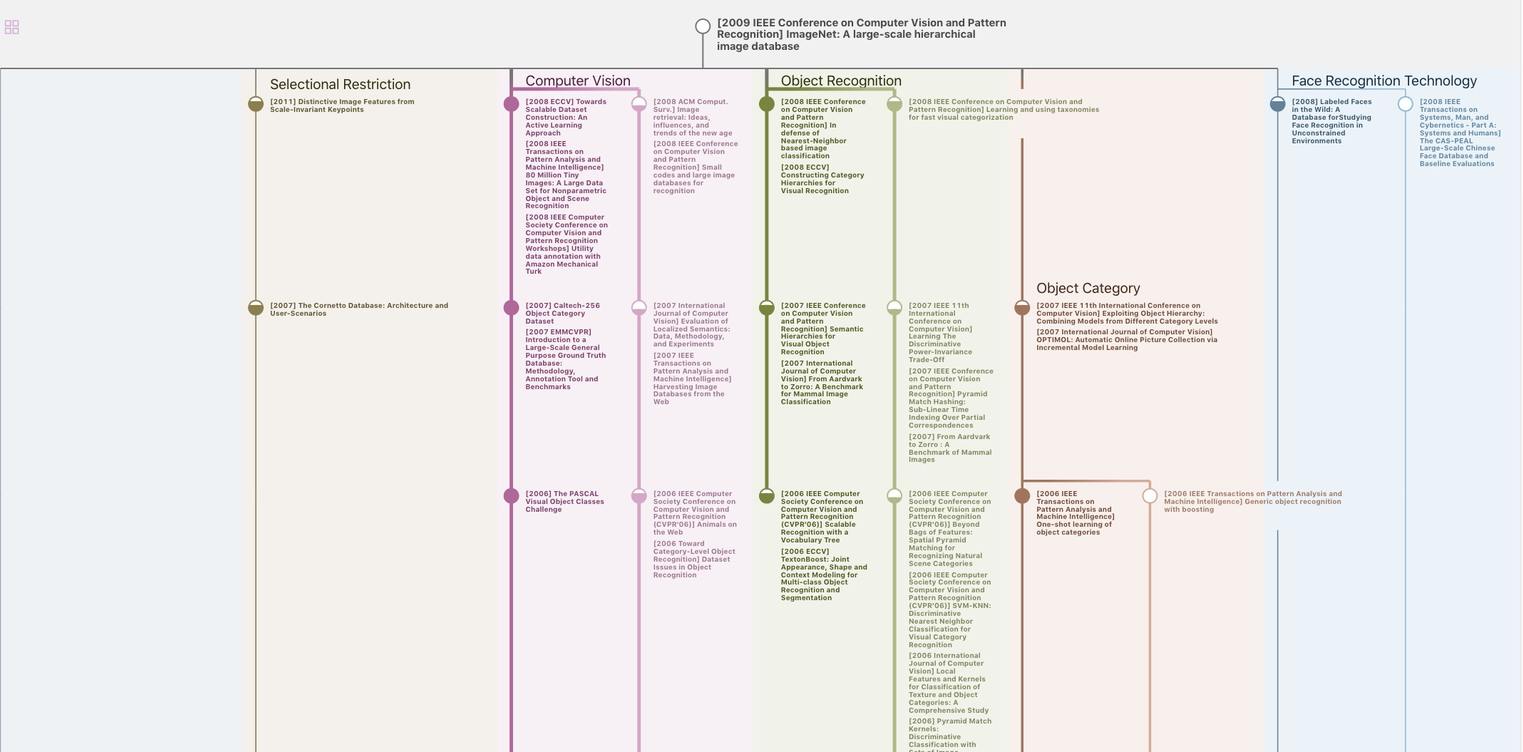
生成溯源树,研究论文发展脉络
Chat Paper
正在生成论文摘要