Skip The Question You Don'T Know: An Embedding Space Approach
2019 INTERNATIONAL JOINT CONFERENCE ON NEURAL NETWORKS (IJCNN)(2019)
摘要
Deep neural network gives people power to generalize hidden patterns behind training data. However, due to limitations on available data collection methods, what neural networks learn should never be expected to deal with all the scenarios: predicting on samples that rarely appear in training set will have very low accuracy. Thus, we design an end-to-end neural network. It learns an inherent discriminative embedding on the training set to perform out-of-distribution(OOD) detection and classification at the same time: both OOD data points and points that resemble those with different labels can be visually observed in this embedding space. Based on this model, we also devise a training scheme that trains on only inliers. Experiments on various datasets and metrics validate that our method outperforms the state-of-art OOD detector.
更多查看译文
关键词
Out-of-Distribution Detection, Machine Learning, Neural Network, Novelty Detection, Embedding
AI 理解论文
溯源树
样例
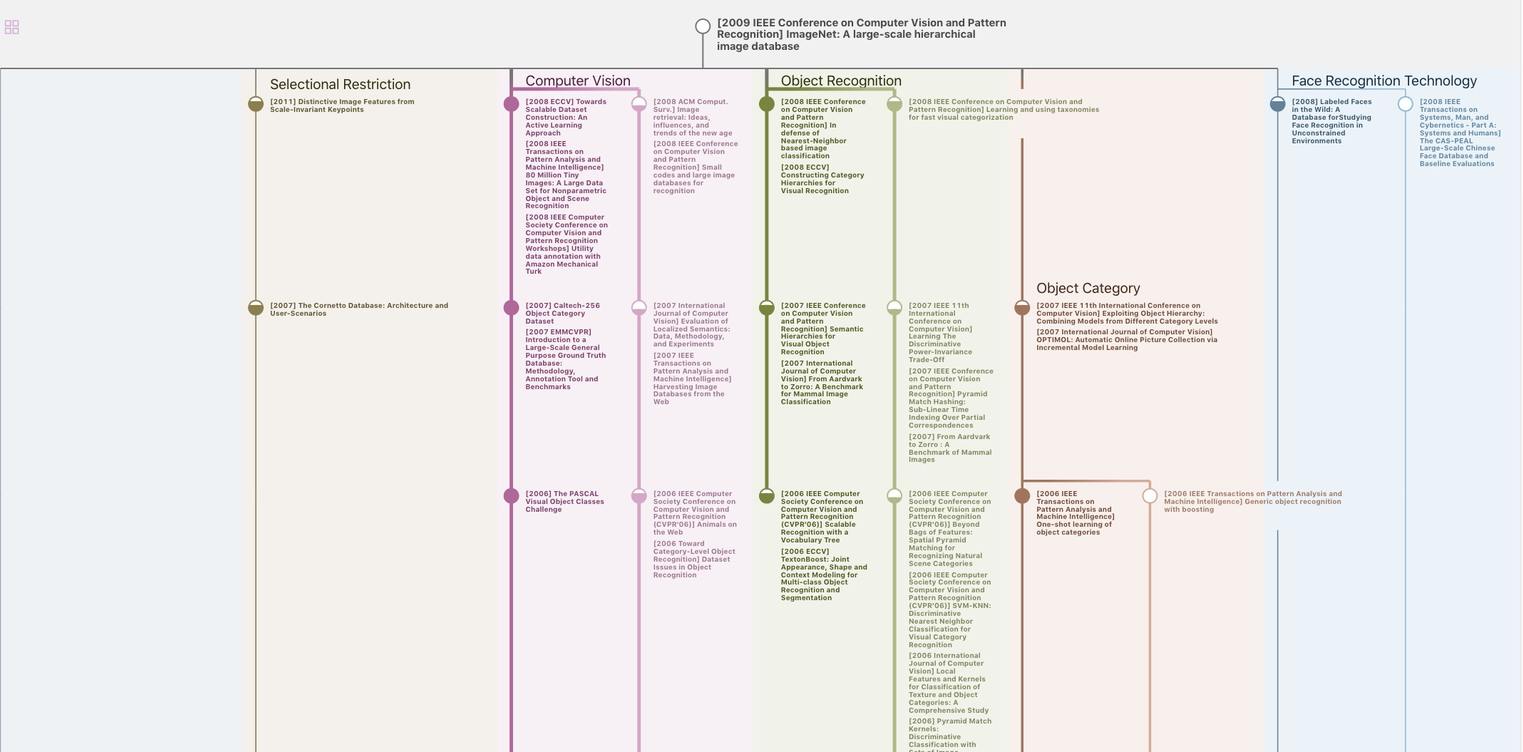
生成溯源树,研究论文发展脉络
Chat Paper
正在生成论文摘要