Peak Area Detection Network For Directly Learning Phase Regions From Raw X-Ray Diffraction Patterns
2019 INTERNATIONAL JOINT CONFERENCE ON NEURAL NETWORKS (IJCNN)(2019)
摘要
X-ray diffraction (XRD) is a well-known technique used by scientists and engineers to determine the atomic-scale structures as a basis for understanding the composition-structure-property relationship of materials. The current approach for the analysis of XRD data is a multi-stage process requiring several intensive computations such as integration along 2 theta for conversion to 1D patterns (intensity-2 theta), background removal by polynomial fitting, and indexing against a large database of reference peaks. It impacts the decisions about the subsequent experiments of the materials under investigation and delays the overall process. In this paper, we focus on eliminating such multi-stage XRD analysis by directly learning the phase regions from the raw (2D) XRD image. We introduce a peak area detection network (PADNet) that directly learns to predict the phase regions using the raw XRD patterns without any need for explicit preprocessing and background removal. PADNet contains specially designed large symmetrical convolutional filters at the first layer to capture the peaks and automatically remove the background by computing the difference in intensity counts across different symmetries. We evaluate PADNet using two sets of XRD patterns collected from SLAC and Bruker D-8 for the Sn-Ti-Zn-O composition space; each set contains 177 experimental XRD patterns with their phase regions. We find that PADNet can successfully classify the XRD patterns independent of the presence of background noise and perform better than the current approach of extrapolating phase region labels based on 1D XRD patterns.
更多查看译文
关键词
PADNet,peak area detection network,atomic-scale structures,intensive computations,reference peaks,X-ray diffraction,composition-structure-property,polynomial fitting,XRD image,phase region labels,multistage XRD analysis elimination,symmetrical convolutional filters,composition space,1D XRD patterns,SnTiZnO
AI 理解论文
溯源树
样例
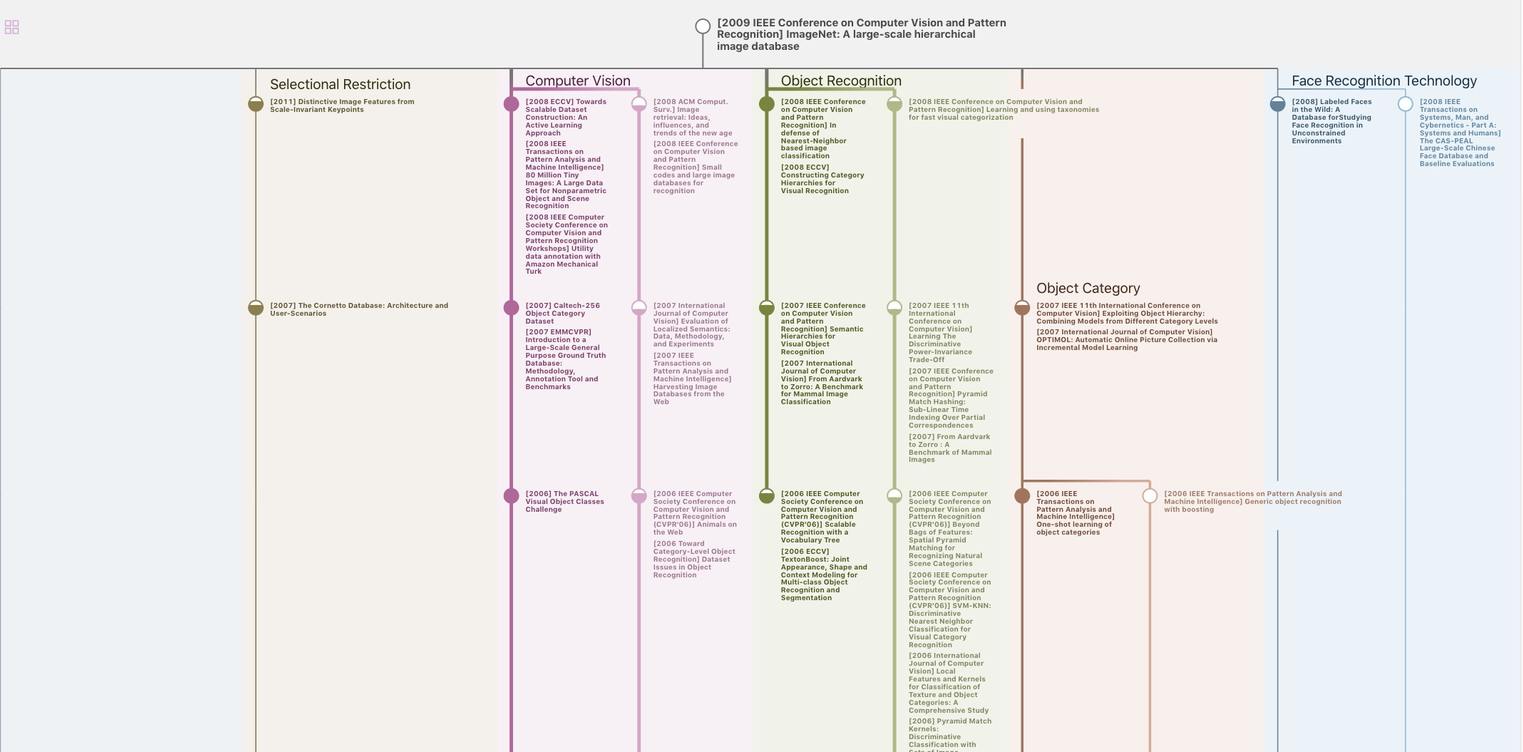
生成溯源树,研究论文发展脉络
Chat Paper
正在生成论文摘要