Gated Sequential Recommendation With Dynamic Memory Network
2019 INTERNATIONAL JOINT CONFERENCE ON NEURAL NETWORKS (IJCNN)(2019)
摘要
Recommender systems provide users with a ranked list of items based on users' historical behavior. Existing methods usually embed users with their temporal dynamics, which may have lost their long-term, intrinsic preferences. RNN is widely adopted to model users' behaviors for its expressiveness on modeling sequential information. However, RNN-based methods are insufficient to capture users' intrinsic preferences due to short-term dependency issues. In this paper, we aim to make progress from two perspectives: 1. extract users' intrinsic preferences from their historical behaviors more effectively and robustly; 2. explore a fine-tuning way to combine users' intrinsic preferences and external dynamic interests. To do so, we propose a modification of Dynamic Memory Network with gating mechanism for generating a dynamic, precise representation for individual users. We also design an automatic and implicit method, and it utilizes the intrinsic preferences as a trigger signal to search the input sequences and retrieves the temporal dynamics of users in the memory module. As a result, a desirable combination of temporal dynamics and intrinsic preferences is achieved. Extensive experiments on three benchmark datasets have shown our model's superiority over the existing baseline method. Further analysis of the attention weights of each hop demonstrated the effectiveness of the proposed method.
更多查看译文
关键词
sequential recommendation, dynamic memory, gating mechanism, RNN
AI 理解论文
溯源树
样例
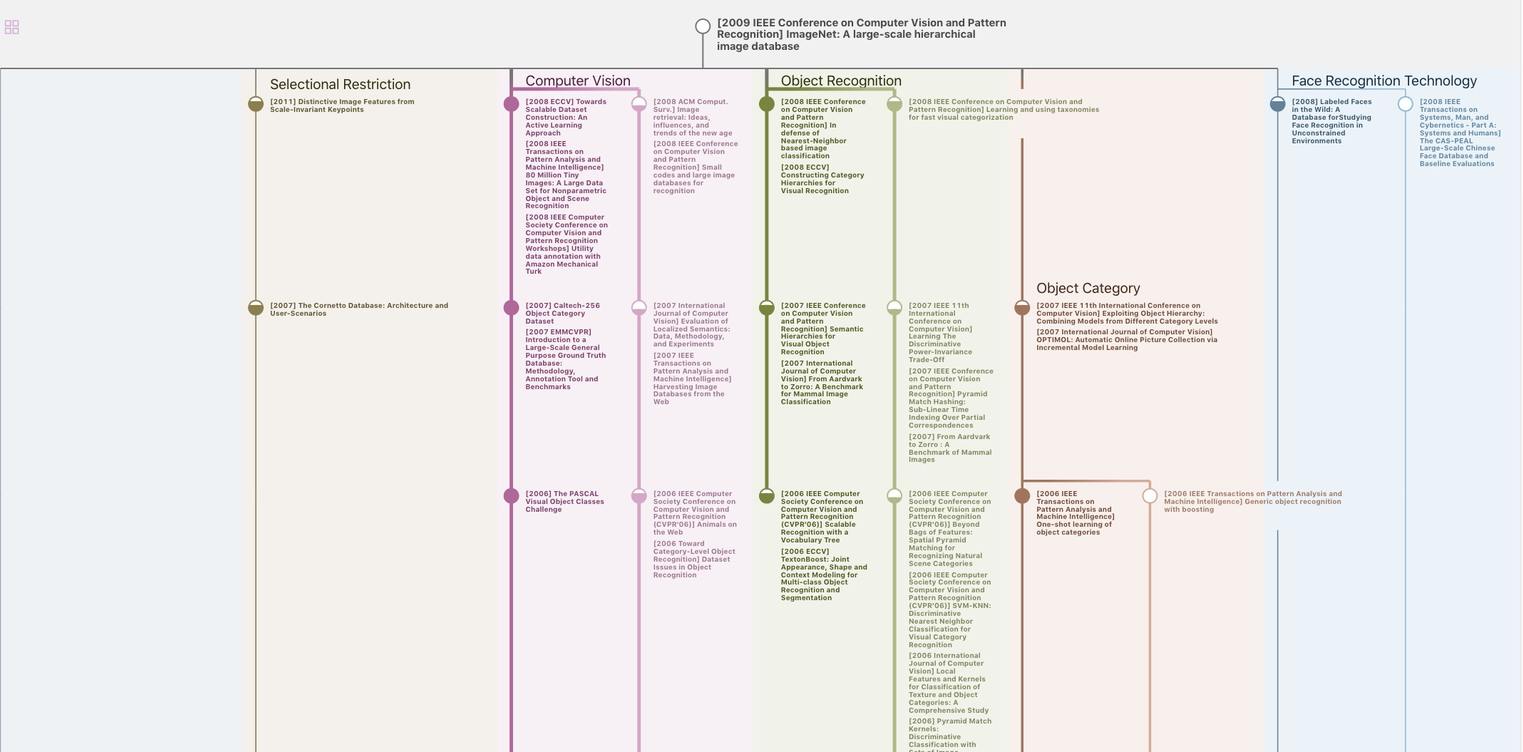
生成溯源树,研究论文发展脉络
Chat Paper
正在生成论文摘要