Rit2fis: A Recurrent Interval Type 2 Fuzzy Inference System And Its Rule Base Estimation
2019 INTERNATIONAL JOINT CONFERENCE ON NEURAL NETWORKS (IJCNN)(2019)
摘要
Two of the major challenges associated with time series modelling are handling uncertainty present in the data and tracing its dynamical behaviour. A Recurrent Interval Type 2 Fuzzy Inference System or RIT2FIS is presented in this paper. RIT2FIS adopts an interval type 2 fuzzy inference mechanism for superior handling of uncertainty. The memory neurons employed in its hidden and output layer, retain the temporal information, making RIT2FIS highly proficient in tracing system dynamics at a granular level. RIT2FIS also benefits from incorporating a k-means algorithm inspired approach to cluster the data in an unsupervised manner. An 'Elbow Method' is utilized next to determine the optimal clustering which is then employed as the optimal fuzzy rule base for RIT2FIS, eliminating the necessity of expert knowledge for fuzzy initiation. The antecedent and consequent parameters of RIT2FIS are updated using a gradient descent based back-propagation through time algorithm where the learning is made self-regulatory to avoid over-fitting and ensure generalization. Performance of RIT2FIS is evaluated against popular neuro-fuzzy methods on different benchmark and real-world time series problems which distinctly indicates an improved accuracy and a parsimonious rule base.
更多查看译文
关键词
Recurrent Neural Fuzzy Network, type 2 Fuzzy Inference System, Fuzzy Rule Base Estimation, k-Means Clustering, Time Series Forecasting, Dynamic System Identification
AI 理解论文
溯源树
样例
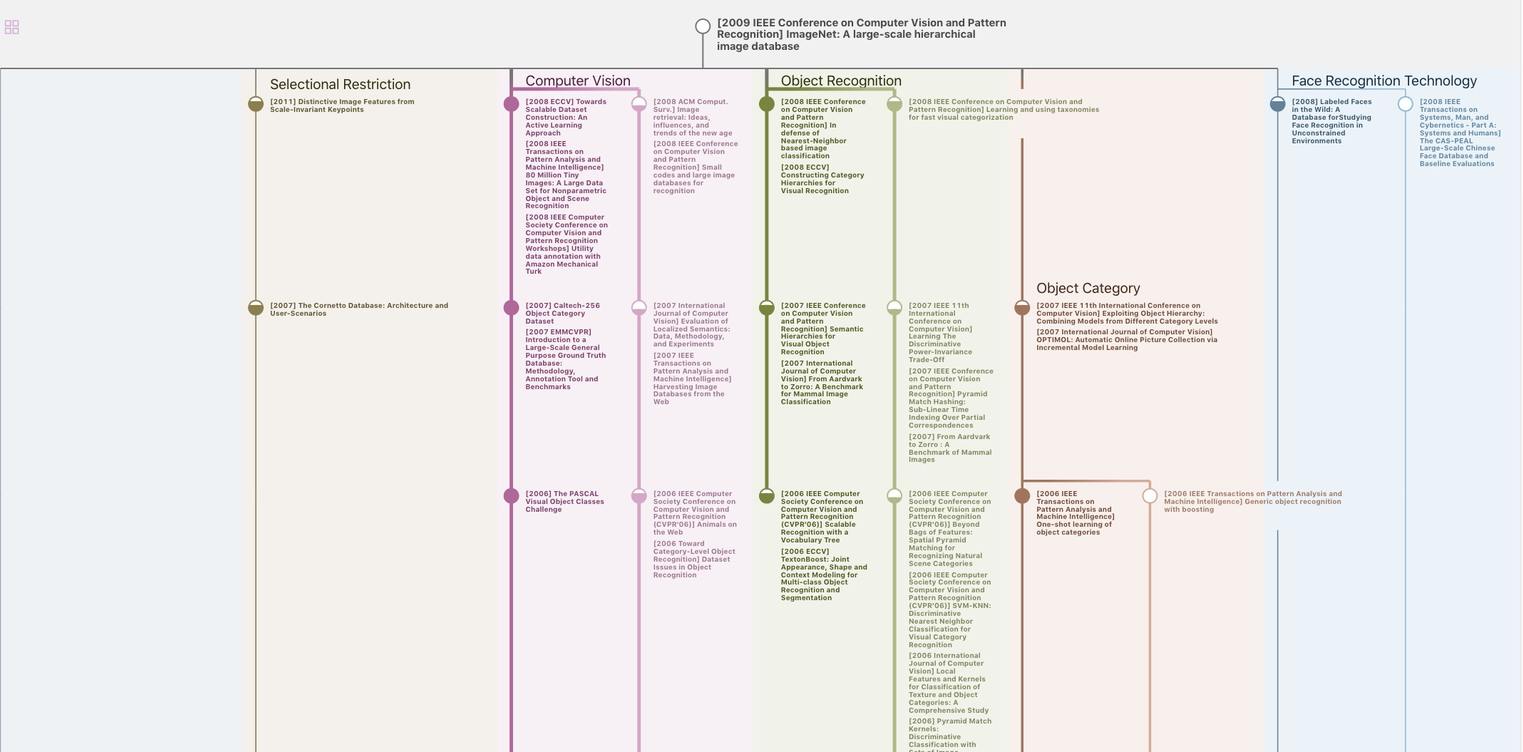
生成溯源树,研究论文发展脉络
Chat Paper
正在生成论文摘要