Fast and Efficient Text Classification with Class-based Embeddings
2019 International Joint Conference on Neural Networks (IJCNN)(2019)
摘要
Current state-of-the-art approaches for Natural Language Processing tasks such as text classification are either based on Recurrent or Convolutional Neural Networks. Notwithstanding, those approaches often require a long time to train, or large amounts of memory to store the entire trained models. In this paper, we introduce a novel neural network architecture for ultra-fast, memory-efficient text classification. The proposed architecture is based on word embeddings trained directly over the class space, which allows for fast, efficient, and effective text classification. We divide the proposed architecture into four main variations that present distinct capabilities for learning temporal relations. We perform several experiments across four widely-used datasets, in which we achieve results comparable to the state-of-the-art while being much faster and lighter in terms of memory usage. We also present a thorough ablation study to demonstrate the importance of each component within each proposed model. Finally, we show that our model predictions can be visualized and thus easily explained.
更多查看译文
关键词
Text classification,deep learning,neural networks,natural language processing
AI 理解论文
溯源树
样例
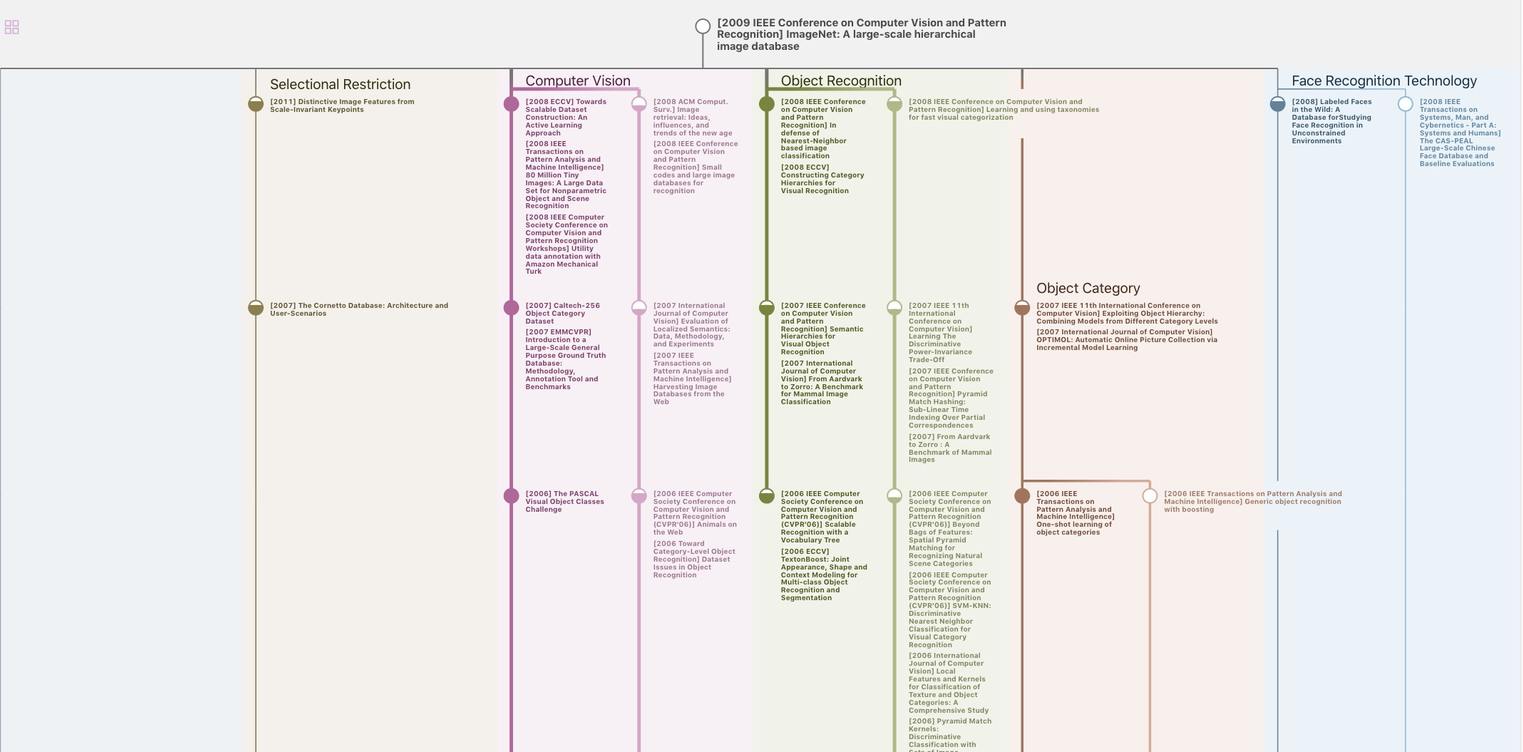
生成溯源树,研究论文发展脉络
Chat Paper
正在生成论文摘要