Deep Learning Based Domain Knowledge Integration For Small Datasets: Illustrative Applications In Materials Informatics
2019 INTERNATIONAL JOINT CONFERENCE ON NEURAL NETWORKS (IJCNN)(2019)
摘要
Deep learning has shown its superiority to traditional machine learning methods in various fields, and in general, its success depends on the availability of large amounts of reliable data. However, in some scientific fields such as materials science, such big data is often expensive or even impossible to collect. Thus given relatively small datasets, most of data-driven methods are based on traditional machine learning methods, and it is challenging to apply deep learning for many tasks in these fields. In order to take the advantage of deep learning even for small datasets, a domain knowledge integration approach is proposed in this work. The efficacy of the proposed approach is tested on two materials science datasets with different types of inputs and outputs, for which domain knowledge-aware convolutional neural networks (CNNs) are developed and evaluated against traditional machine learning methods and standard CNN-based approaches. Experiment results demonstrate that integrating domain knowledge into deep learning can not only improve the model's performance for small datasets, but also make the prediction results more explainable based on domain knowledge.
更多查看译文
关键词
data-driven methods,materials science datasets,deep learning,convolutional neural networks,machine learning methods,domain knowledge integration,CNN-based approach,big data
AI 理解论文
溯源树
样例
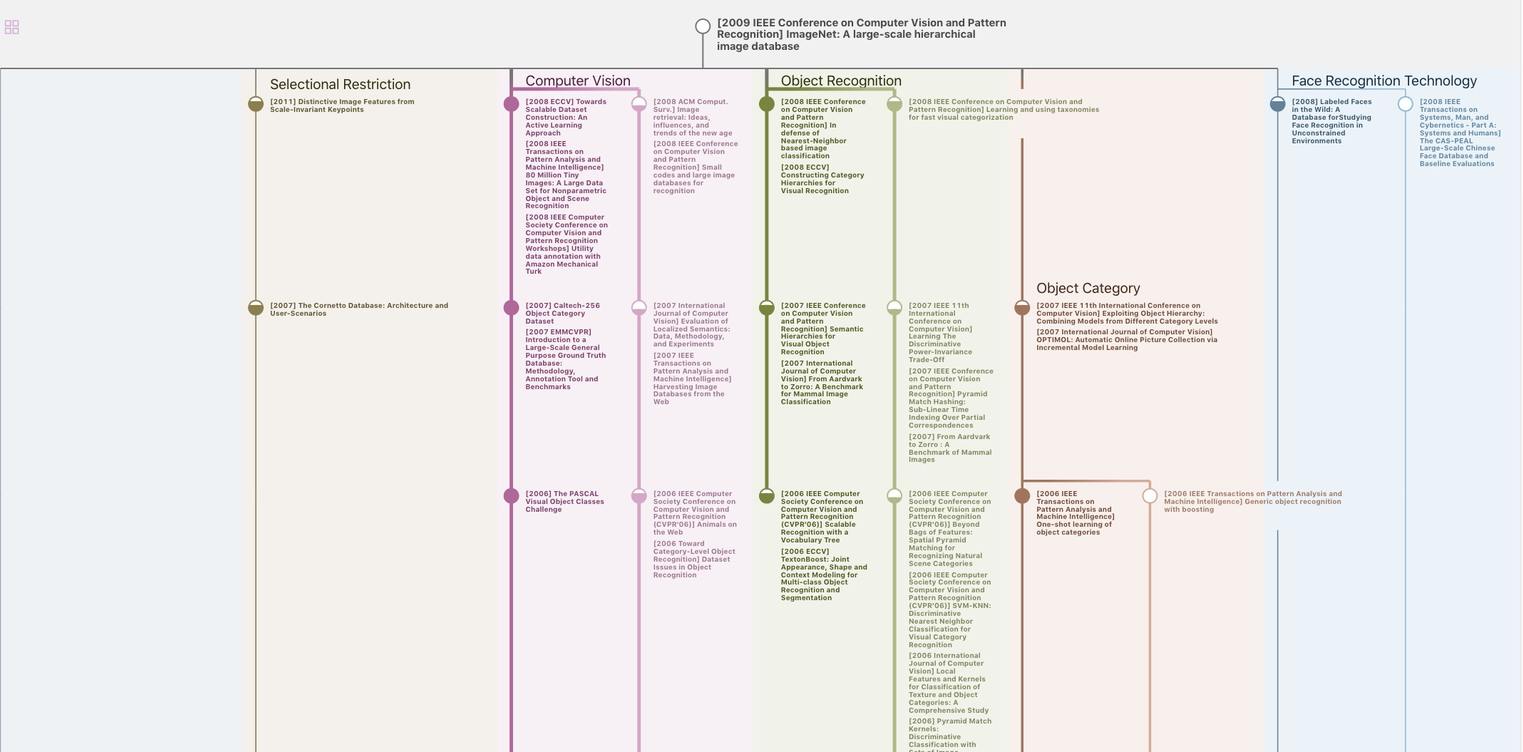
生成溯源树,研究论文发展脉络
Chat Paper
正在生成论文摘要