A Robust Embedding Method For Anomaly Detection On Attributed Networks
2019 INTERNATIONAL JOINT CONFERENCE ON NEURAL NETWORKS (IJCNN)(2019)
摘要
Anomalies detection is to spot the objects whose patterns singularly differ from the reference majority. Attributed networks often contain node attributes and network structure, which are widely used for real-life applications. Meanwhile, how to detect anomalies on attributed networks has caused a lot of attention. Most existing works on anomaly detection attempt to incorporate node attributes with the network structure. However, there may exist structurally irrelevant attributes in the networks, which may have adverse effects on the detection results. Besides, the heterogeneity of node attributes and network structure may further make the detection of anomalies difficult. In order to overcome the above challenges, in this paper, we propose a Robust Embedding Method for Anomaly Detection on Attributed Networks, called REMAD. Methodologically, the proposed REMAD combines network embedding and residual analysis together. By performing network embedding on the network, REMAD obtains the representative attributes that are closely coherent with the network structure. Simultaneously, by adopting the residual analysis, REMAD characterizes and analyzes the residuals of attribute information to discover anomalies. Experimental results on both synthetic and real-world datasets demonstrate the advantages of our proposed method REMAD against the state-of-the-art anomaly detection methods.
更多查看译文
关键词
Anomaly Detection, Attributed Networks, Network Embedding
AI 理解论文
溯源树
样例
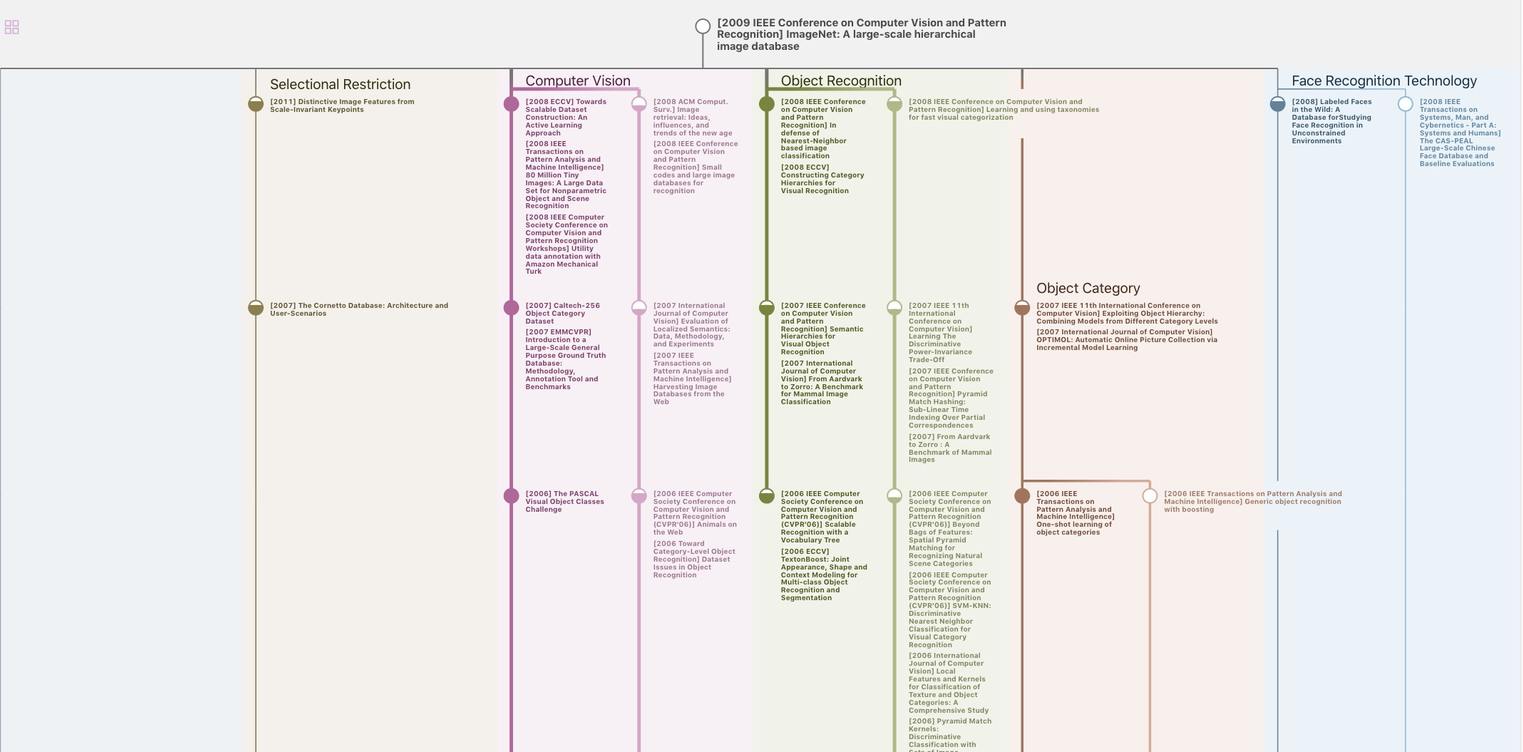
生成溯源树,研究论文发展脉络
Chat Paper
正在生成论文摘要